Revision as of 05:48, 4 July 2021 editCitation bot (talk | contribs)Bots5,435,741 edits Alter: journal, pages. Add: pmc, url, page, s2cid, doi-access, bibcode. Formatted dashes. | Use this bot. Report bugs. | Suggested by Headbomb | #UCB_webform 4/5← Previous edit | Latest revision as of 05:04, 28 October 2024 edit undoCitation bot (talk | contribs)Bots5,435,741 edits Add: bibcode, page, pmc, pmid. | Use this bot. Report bugs. | Suggested by Dominic3203 | Category:Gene expression | #UCB_Category 52/394 | ||
(78 intermediate revisions by 39 users not shown) | |||
Line 1: | Line 1: | ||
{{Short description|Lab technique in cellular biology}} | {{Short description|Lab technique in cellular biology}} | ||
{{cs1 config|name-list-style=vanc|display-authors=6}} | |||
{{Use dmy dates|date=October 2021}} | |||
[[File:Summary_of_RNA-Seq.svg|thumb|500x500px|''Summary of RNA-Seq.'' Within the organism, genes are transcribed and (in | [[File:Summary_of_RNA-Seq.svg|thumb|500x500px|''Summary of RNA-Seq.'' Within the organism, genes are transcribed and (in | ||
an ]) spliced to produce mature mRNA transcripts (red). The mRNA is extracted from the organism, fragmented and copied into stable ds-cDNA (blue). The ds-cDNA is sequenced using ], short-read sequencing methods. These sequences can then be ] to a reference genome sequence to reconstruct which genome regions were being transcribed. This data can be used to annotate where expressed genes are, their relative expression levels, and any alternative splice variants.<ref>{{cite journal | vauthors = Lowe R, Shirley N, Bleackley M, Dolan S, Shafee T | title = Transcriptomics technologies | journal = PLOS Computational Biology | volume = 13 | issue = 5 | pages = e1005457 | date = May 2017 | pmid = 28545146 | pmc = 5436640 | doi = 10.1371/journal.pcbi.1005457 | bibcode = 2017PLSCB..13E5457L }}</ref>]] | an ]) spliced to produce mature mRNA transcripts (red). The mRNA is extracted from the organism, fragmented and copied into stable ds-cDNA (blue). The ds-cDNA is sequenced using ], short-read sequencing methods. These sequences can then be ] to a reference genome sequence to reconstruct which genome regions were being transcribed. This data can be used to annotate where expressed genes are, their relative expression levels, and any alternative splice variants.<ref>{{cite journal | vauthors = Lowe R, Shirley N, Bleackley M, Dolan S, Shafee T | title = Transcriptomics technologies | journal = PLOS Computational Biology | volume = 13 | issue = 5 | pages = e1005457 | date = May 2017 | pmid = 28545146 | pmc = 5436640 | doi = 10.1371/journal.pcbi.1005457 | bibcode = 2017PLSCB..13E5457L | doi-access = free }}</ref>]] | ||
⚫ | '''RNA-Seq''' (named as an abbreviation of |
||
⚫ | '''RNA-Seq''' (named as an abbreviation of '''RNA sequencing''') is a technique that uses ] to reveal the presence and quantity of ] molecules in a biological sample, providing a snapshot of gene expression in the sample, also known as ].<ref>{{cite journal | vauthors = Chu Y, Corey DR | title = RNA sequencing: platform selection, experimental design, and data interpretation | journal = Nucleic Acid Therapeutics | volume = 22 | issue = 4 | pages = 271–4 | date = August 2012 | pmid = 22830413 | pmc = 3426205 | doi = 10.1089/nat.2012.0367 }}</ref><ref name="wang2009"/> | ||
⚫ | Specifically, RNA-Seq facilitates the ability to look at ], ]s, ], mutations/] and changes in gene expression over time, or differences in gene expression in different groups or treatments.<ref name="maher2009"/> |
||
⚫ | Specifically, RNA-Seq facilitates the ability to look at ], ]s, ], mutations/] and changes in ] over time, or differences in gene expression in different groups or treatments.<ref name="maher2009"/> In addition to mRNA transcripts, RNA-Seq can look at different populations of RNA to include total RNA, small RNA, such as ], ], and ].<ref>{{cite journal | vauthors = Ingolia NT, Brar GA, Rouskin S, McGeachy AM, Weissman JS | title = The ribosome profiling strategy for monitoring translation in vivo by deep sequencing of ribosome-protected mRNA fragments | journal = Nature Protocols | volume = 7 | issue = 8 | pages = 1534–50 | date = July 2012 | pmid = 22836135 | pmc = 3535016 | doi = 10.1038/nprot.2012.086 }}</ref> RNA-Seq can also be used to determine ]/] boundaries and verify or amend previously ] ] and ] gene boundaries. Recent advances in RNA-Seq include ], ],<ref>{{cite journal | vauthors = Alpern D, Gardeux V, Russeil J, Mangeat B, Meireles-Filho AC, Breysse R, Hacker D, Deplancke B | title = BRB-seq: ultra-affordable high-throughput transcriptomics enabled by bulk RNA barcoding and sequencing | journal = Genome Biology | volume = 20 | issue = 1 | pages = 71 | date = April 2019 | pmid = 30999927 | pmc = 6474054 | doi = 10.1186/s13059-019-1671-x | doi-access = free }}</ref> 3' mRNA-sequencing''',''' in situ sequencing of fixed tissue, and native RNA molecule sequencing with single-molecule real-time sequencing.<ref>{{cite journal | vauthors = Lee JH, Daugharthy ER, Scheiman J, Kalhor R, Yang JL, Ferrante TC, Terry R, Jeanty SS, Li C, Amamoto R, Peters DT, Turczyk BM, Marblestone AH, Inverso SA, Bernard A, Mali P, Rios X, Aach J, Church GM | title = Highly multiplexed subcellular RNA sequencing in situ | journal = Science | volume = 343 | issue = 6177 | pages = 1360–3 | date = March 2014 | pmid = 24578530 | pmc = 4140943 | doi = 10.1126/science.1250212 | bibcode = 2014Sci...343.1360L }}</ref> Other examples of emerging RNA-Seq applications due to the advancement of bioinformatics algorithms are copy number alteration, microbial contamination, transposable elements, cell type (deconvolution) and the presence of neoantigens.<ref name="Thind_2021">{{cite journal | vauthors = Thind AS, Monga I, Thakur PK, Kumari P, Dindhoria K, Krzak M, Ranson M, Ashford B | title = Demystifying emerging bulk RNA-Seq applications: the application and utility of bioinformatic methodology | journal = Briefings in Bioinformatics | volume = 22 | issue = 6 | date = November 2021 | pmid = 34329375 | doi = 10.1093/bib/bbab259 }}</ref> | ||
⚫ | Prior to RNA-Seq, gene expression studies were done with hybridization-based ]. Issues with microarrays include cross-hybridization artifacts, poor quantification of lowly and highly expressed genes, and needing to know the sequence ].<ref>{{cite journal | vauthors = Kukurba KR, Montgomery SB | title = RNA Sequencing and Analysis | journal = Cold Spring Harbor Protocols | volume = 2015 | issue = 11 | pages = 951–69 | date = April 2015 | pmid = 25870306 | pmc = 4863231 | doi = 10.1101/pdb.top084970 }}</ref> Because of these technical issues, ] transitioned to sequencing-based methods. These progressed from ] of ] libraries, to chemical tag-based methods (e.g., ]), and finally to the current technology, ] of ] (cDNA), notably RNA-Seq. | ||
⚫ | Prior to RNA-Seq, gene expression studies were done with hybridization-based ]. Issues with microarrays include cross-hybridization artifacts, poor quantification of lowly and highly expressed genes, and needing to know the sequence ].<ref>{{cite journal | vauthors = Kukurba KR, Montgomery SB | title = RNA Sequencing and Analysis | journal = Cold Spring Harbor Protocols | volume = 2015 | issue = 11 | pages = 951–69 | date = April 2015 | pmid = 25870306 | pmc = 4863231 | doi = 10.1101/pdb.top084970 }}</ref> Because of these technical issues, ] transitioned to sequencing-based methods. These progressed from ] of ] libraries, to chemical tag-based methods (e.g., ]), and finally to the current technology, ] of ] (cDNA), notably RNA-Seq. | ||
] | |||
==Methods== | ==Methods== | ||
Line 13: | Line 18: | ||
] | ] | ||
The general steps to prepare a ] (cDNA) library for sequencing are described below, but often vary between platforms.<ref name="ReferenceA">{{cite journal | vauthors = Griffith M, Walker JR, Spies NC, Ainscough BJ, Griffith OL | title = Informatics for RNA Sequencing: A Web Resource for Analysis on the Cloud | journal = PLOS Computational Biology | volume = 11 | issue = 8 | pages = e1004393 | date = August 2015 | pmid = 26248053 | pmc = 4527835 | doi = 10.1371/journal.pcbi.1004393 | bibcode = 2015PLSCB..11E4393G }}</ref><ref name="wang2009">{{cite journal | vauthors = Wang Z, Gerstein M, Snyder M | title = RNA-Seq: a revolutionary tool for transcriptomics | journal = Nature Reviews. Genetics | volume = 10 | issue = 1 | pages = 57–63 | date = January 2009 | pmid = 19015660 | pmc = 2949280 | doi = 10.1038/nrg2484 }}</ref><ref>{{Cite web |url= http://rnaseq.uoregon.edu/ |title=RNA-seqlopedia |website=rnaseq.uoregon.edu |access-date=2017 |
The general steps to prepare a ] (cDNA) library for sequencing are described below, but often vary between platforms.<ref name="ReferenceA">{{cite journal | vauthors = Griffith M, Walker JR, Spies NC, Ainscough BJ, Griffith OL | title = Informatics for RNA Sequencing: A Web Resource for Analysis on the Cloud | journal = PLOS Computational Biology | volume = 11 | issue = 8 | pages = e1004393 | date = August 2015 | pmid = 26248053 | pmc = 4527835 | doi = 10.1371/journal.pcbi.1004393 | bibcode = 2015PLSCB..11E4393G | doi-access = free }}</ref><ref name="wang2009">{{cite journal | vauthors = Wang Z, Gerstein M, Snyder M | title = RNA-Seq: a revolutionary tool for transcriptomics | journal = Nature Reviews. Genetics | volume = 10 | issue = 1 | pages = 57–63 | date = January 2009 | pmid = 19015660 | pmc = 2949280 | doi = 10.1038/nrg2484 }}</ref><ref>{{Cite web |url= http://rnaseq.uoregon.edu/ |title=RNA-seqlopedia |website=rnaseq.uoregon.edu |access-date=8 February 2017}}</ref> | ||
⚫ | # ''RNA Isolation:'' ] from tissue and mixed with ]. DNase reduces the amount of genomic DNA. The amount of RNA degradation is checked with ] and ] and is used to assign an ] to the sample. This RNA quality and the total amount of starting RNA are taken into consideration during the subsequent library preparation, sequencing, and analysis steps. | ||
⚫ | # |
||
⚫ | # ''RNA Isolation:'' ] from tissue and mixed with ] (DNase). DNase reduces the amount of genomic DNA. The amount of RNA degradation is checked with ] and ] and is used to assign an ] to the sample. This RNA quality and the total amount of starting RNA are taken into consideration during the subsequent library preparation, sequencing, and analysis steps. | ||
⚫ | # |
||
⚫ | #''RNA selection/depletion:'' To analyze signals of interest, the isolated RNA can either be kept as is, enriched for RNA with ] tails to include only eukaryotic ], depleted of ], and/or filtered for RNA that binds specific sequences ('''RNA selection and depletion methods table''', below). RNA molecules having 3' poly(A) tails in eukaryotes are mainly composed of mature, processed, coding sequences. Poly(A) selection is performed by mixing RNA with {{not a typo|poly(T)}} oligomers covalently attached to a substrate, typically magnetic beads.<ref name="morin2008">{{cite journal | vauthors = Morin R, Bainbridge M, Fejes A, Hirst M, Krzywinski M, Pugh T, McDonald H, Varhol R, Jones S, Marra M | title = Profiling the HeLa S3 transcriptome using randomly primed cDNA and massively parallel short-read sequencing | journal = BioTechniques | volume = 45 | issue = 1 | pages = 81–94 | date = July 2008 | pmid = 18611170 | doi = 10.2144/000112900 | doi-access = free }}</ref><ref name="mortazavi2008">{{cite journal | vauthors = Mortazavi A, Williams BA, McCue K, Schaeffer L, Wold B | title = Mapping and quantifying mammalian transcriptomes by RNA-Seq | journal = Nature Methods | volume = 5 | issue = 7 | pages = 621–8 | date = July 2008 | pmid = 18516045 | doi = 10.1038/nmeth.1226 | s2cid = 205418589 }}</ref> Poly(A) selection has important limitations in RNA biotype detection. Many RNA biotypes are not polyadenylated, including many noncoding RNA and histone-core protein transcripts, or are regulated via their poly(A) tail length (e.g., cytokines) and thus might not be detected after poly(A) selection.<ref>{{cite journal | vauthors = Sun Q, Hao Q, Prasanth KV | title = Nuclear Long Noncoding RNAs: Key Regulators of Gene Expression | journal = Trends in Genetics | volume = 34 | issue = 2 | pages = 142–157 | date = February 2018 | pmid = 29249332 | pmc = 6002860 | doi = 10.1016/j.tig.2017.11.005 }}</ref> Furthermore, poly(A) selection may display increased 3' bias, especially with lower quality RNA.<ref>{{cite journal | vauthors = Sigurgeirsson B, Emanuelsson O, Lundeberg J | title = Sequencing degraded RNA addressed by 3' tag counting | journal = PLOS ONE | volume = 9 | issue = 3 | pages = e91851 | date = 2014 | pmid = 24632678 | pmc = 3954844 | doi = 10.1371/journal.pone.0091851 | bibcode = 2014PLoSO...991851S | doi-access = free }}</ref><ref>{{cite journal | vauthors = Chen EA, Souaiaia T, Herstein JS, Evgrafov OV, Spitsyna VN, Rebolini DF, Knowles JA | title = Effect of RNA integrity on uniquely mapped reads in RNA-Seq | journal = BMC Research Notes | volume = 7 | pages = 753 | date = October 2014 | pmid = 25339126 | pmc = 4213542 | doi = 10.1186/1756-0500-7-753 | doi-access = free }}</ref> These limitations can be avoided with ribosomal depletion, removing rRNA that typically represents over 90% of the RNA in a cell. Both poly(A) enrichment and ribosomal depletion steps are labor intensive and could introduce biases, so more simple approaches have been developed to omit these steps.<ref>{{Cite journal| vauthors = Moll P, Ante M, Seitz A, Reda T |date=December 2014|title=QuantSeq 3′ mRNA sequencing for RNA quantification |journal=Nature Methods|language=en|volume=11|issue=12|pages=i–iii|doi=10.1038/nmeth.f.376|s2cid=83424788 |issn=1548-7105}}</ref> Small RNA targets, such as ], can be further isolated through size selection with exclusion gels, magnetic beads, or commercial kits. | ||
⚫ | #''cDNA synthesis:'' RNA is ] to cDNA because DNA is more stable and to allow for amplification (which uses ]) and leverage more mature DNA sequencing technology. Amplification subsequent to reverse transcription results in loss of ], which can be avoided with chemical labeling or single molecule sequencing. Fragmentation and size selection are performed to purify sequences that are the appropriate length for the sequencing machine. The RNA, cDNA, or both are fragmented with enzymes, ], divalent ions, or nebulizers. Fragmentation of the RNA reduces 5' bias of randomly primed-reverse transcription and the influence of ] binding sites,<ref name="mortazavi2008" /> with the downside that the 5' and 3' ends are converted to DNA less efficiently. Fragmentation is followed by size selection, where either small sequences are removed or a tight range of sequence lengths are selected. Because small RNAs like ] are lost, these are analyzed independently. The cDNA for each experiment can be indexed with a hexamer or octamer barcode, so that these experiments can be pooled into a single lane for multiplexed sequencing. | ||
{| class="wikitable" | {| class="wikitable" | ||
|+ style="text-align: left;" | RNA selection and depletion methods:<ref name="ReferenceA"/> | |+ style="text-align: left;" | RNA selection and depletion methods:<ref name="ReferenceA"/> | ||
Line 37: | Line 40: | ||
{{See also|w:DNA sequencing}} | {{See also|w:DNA sequencing}} | ||
The cDNA library derived from RNA biotypes is then sequenced into a computer-readable format. There are many high-throughput sequencing technologies for cDNA sequencing including platforms developed by ], ], ], ], and ].<ref>{{cite journal | vauthors = Oikonomopoulos S, Bayega A, Fahiminiya S, Djambazian H, Berube P, Ragoussis J | title = Methodologies for Transcript Profiling Using Long-Read Technologies | language = English | journal = Frontiers in Genetics | volume = 11 | pages = 606 | date = 2020 | pmid = 32733532 | doi = 10.3389/fgene.2020.00606 | pmc = 7358353 | doi-access = free }}</ref> For Illumina short-read sequencing, a common technology for cDNA sequencing, adapters are ligated to the cDNA, DNA is attached to a flow cell, clusters are generated through cycles of bridge amplification and denaturing, and sequence-by-synthesis is performed in cycles of complementary strand synthesis and laser excitation of bases with reversible terminators. Sequencing platform choice and parameters are guided by experimental design and cost. Common experimental design considerations include deciding on the sequencing length, sequencing depth, use of single versus paired-end sequencing, number of replicates, multiplexing, randomization, and spike-ins.<ref name="A survey of best practices for RNA">{{cite journal | vauthors = Conesa A, Madrigal P, Tarazona S, Gomez-Cabrero D, Cervera A, McPherson A, Szcześniak MW, Gaffney DJ, Elo LL, Zhang X, Mortazavi A |
The cDNA library derived from RNA biotypes is then sequenced into a computer-readable format. There are many high-throughput sequencing technologies for cDNA sequencing including platforms developed by ], ], ], ], and ].<ref>{{cite journal | vauthors = Oikonomopoulos S, Bayega A, Fahiminiya S, Djambazian H, Berube P, Ragoussis J | title = Methodologies for Transcript Profiling Using Long-Read Technologies | language = English | journal = Frontiers in Genetics | volume = 11 | pages = 606 | date = 2020 | pmid = 32733532 | doi = 10.3389/fgene.2020.00606 | pmc = 7358353 | doi-access = free }}</ref> For Illumina short-read sequencing, a common technology for cDNA sequencing, adapters are ligated to the cDNA, DNA is attached to a flow cell, clusters are generated through cycles of bridge amplification and denaturing, and sequence-by-synthesis is performed in cycles of complementary strand synthesis and laser excitation of bases with reversible terminators. Sequencing platform choice and parameters are guided by experimental design and cost. Common experimental design considerations include deciding on the sequencing length, sequencing depth, use of single versus paired-end sequencing, number of replicates, multiplexing, randomization, and spike-ins.<ref name="A survey of best practices for RNA">{{cite journal | vauthors = Conesa A, Madrigal P, Tarazona S, Gomez-Cabrero D, Cervera A, McPherson A, Szcześniak MW, Gaffney DJ, Elo LL, Zhang X, Mortazavi A | title = A survey of best practices for RNA-seq data analysis | journal = Genome Biology | volume = 17 | issue = 1 | pages = 13 | date = January 2016 | pmid = 26813401 | pmc = 4728800 | doi = 10.1186/s13059-016-0881-8 | doi-access = free }}</ref> | ||
===Small RNA/non-coding RNA sequencing=== | ===Small RNA/non-coding RNA sequencing=== | ||
Line 44: | Line 47: | ||
===Direct RNA sequencing=== | ===Direct RNA sequencing=== | ||
] | ] | ||
Because converting RNA into ], ligation, amplification, and other sample manipulations have been shown to introduce biases and artifacts that may interfere with both the proper characterization and quantification of transcripts,<ref>{{cite journal | vauthors = Liu D, Graber JH | title = Quantitative comparison of EST libraries requires compensation for systematic biases in cDNA generation | journal = BMC Bioinformatics | volume = 7 | pages = 77 | date = February 2006 | pmid = 16503995 | pmc = 1431573 | doi = 10.1186/1471-2105-7-77 }}</ref> single molecule direct RNA sequencing has been explored by companies including ] (bankrupt), ],<ref name = "Garalde_2018">{{cite journal | vauthors = Garalde DR, Snell EA, Jachimowicz D, Sipos B, Lloyd JH, Bruce M, Pantic N, Admassu T, James P, Warland A, Jordan M, Ciccone J, Serra S, Keenan J, Martin S, McNeill L, Wallace EJ, Jayasinghe L, Wright C, Blasco J, Young S, Brocklebank D, Juul S, Clarke J, Heron AJ, Turner DJ |
Because converting RNA into ], ligation, amplification, and other sample manipulations have been shown to introduce biases and artifacts that may interfere with both the proper characterization and quantification of transcripts,<ref>{{cite journal | vauthors = Liu D, Graber JH | title = Quantitative comparison of EST libraries requires compensation for systematic biases in cDNA generation | journal = BMC Bioinformatics | volume = 7 | pages = 77 | date = February 2006 | pmid = 16503995 | pmc = 1431573 | doi = 10.1186/1471-2105-7-77 | doi-access = free }}</ref> single molecule direct RNA sequencing has been explored by companies including ] (bankrupt), ],<ref name = "Garalde_2018">{{cite journal | vauthors = Garalde DR, Snell EA, Jachimowicz D, Sipos B, Lloyd JH, Bruce M, Pantic N, Admassu T, James P, Warland A, Jordan M, Ciccone J, Serra S, Keenan J, Martin S, McNeill L, Wallace EJ, Jayasinghe L, Wright C, Blasco J, Young S, Brocklebank D, Juul S, Clarke J, Heron AJ, Turner DJ | title = Highly parallel direct RNA sequencing on an array of nanopores | journal = Nature Methods | volume = 15 | issue = 3 | pages = 201–206 | date = March 2018 | pmid = 29334379 | doi = 10.1038/nmeth.4577 | s2cid = 3589823 }}</ref> and others. This technology sequences RNA molecules directly in a massively-parallel manner. | ||
===Single-molecule real-time RNA sequencing=== | ===Single-molecule real-time RNA sequencing=== | ||
{{See also|Single-molecule real-time sequencing}} | {{See also|Single-molecule real-time sequencing}} | ||
Massively parallel single molecule direct RNA-Seq has been explored as an alternative to traditional RNA-Seq, in which RNA-to-] conversion, ligation, |
Massively parallel single molecule direct RNA-Seq has been explored as an alternative to traditional RNA-Seq, in which RNA-to-] conversion, ligation, amplification, and other sample manipulation steps may introduce biases and artifacts.<ref>{{cite journal | vauthors = Liu D, Graber JH | title = Quantitative comparison of EST libraries requires compensation for systematic biases in cDNA generation | journal = BMC Bioinformatics | volume = 7 | issue = | pages = 77 | date = February 2006 | pmid = 16503995 | pmc = 1431573 | doi = 10.1186/1471-2105-7-77 | doi-access = free }}</ref> Technology platforms that perform single-molecule real-time RNA-Seq include ] ],<ref name = "Garalde_2018" /> ] IsoSeq, and ] (bankrupt). Sequencing RNA in its native form preserves modifications like methylation, allowing them to be investigated directly and simultaneously.<ref name = "Garalde_2018" /> Another benefit of single-molecule RNA-Seq is that transcripts can be covered in full length, allowing for higher confidence isoform detection and quantification compared to short-read sequencing. Traditionally, single-molecule RNA-Seq methods have higher error rates compared to short-read sequencing, but newer methods like ONT direct RNA-Seq limit errors by avoiding fragmentation and cDNA conversion. Recent uses of ONT direct RNA-Seq for differential expression in human cell populations have demonstrated that this technology can overcome many limitations of short and long cDNA sequencing.<ref>{{Cite journal| vauthors = Gleeson J, Lane TA, Harrison PJ, Haerty W, Clark MB |date=3 August 2020|title=Nanopore direct RNA sequencing detects differential expression between human cell populations |journal=bioRxiv|language=en|pages=2020.08.02.232785|doi=10.1101/2020.08.02.232785|s2cid=220975367|doi-access=free}}</ref> | ||
=== Single-cell RNA sequencing (scRNA-Seq) === | === Single-cell RNA sequencing (scRNA-Seq) === | ||
{{Main|Single-cell transcriptomics}} | |||
{{See also| Single cell sequencing}} | {{See also| Single cell sequencing}} | ||
Standard methods such as ]s and standard bulk RNA-Seq analysis analyze the expression of RNAs from large populations of cells. In mixed cell populations, these measurements may obscure critical differences between individual cells within these populations.<ref name=Shapiro>"{{cite journal | vauthors = Shapiro E, Biezuner T, Linnarsson S | title = Single-cell sequencing-based technologies will revolutionize whole-organism science | journal = Nature Reviews. Genetics | volume = 14 | issue = 9 | pages = 618–30 | date = September 2013 | pmid = 23897237 | doi = 10.1038/nrg3542 | s2cid = 500845 }}"</ref><ref name="Kolodziejczyk">{{cite journal | vauthors = Kolodziejczyk AA, Kim JK, Svensson V, Marioni JC, Teichmann SA | title = The technology and biology of single-cell RNA sequencing | journal = Molecular Cell | volume = 58 | issue = 4 | pages = 610–20 | date = May 2015 | pmid = 26000846 | doi = 10.1016/j.molcel.2015.04.005 | doi-access = free }}</ref> | Standard methods such as ]s and standard bulk RNA-Seq analysis analyze the expression of RNAs from large populations of cells. In mixed cell populations, these measurements may obscure critical differences between individual cells within these populations.<ref name=Shapiro>"{{cite journal | vauthors = Shapiro E, Biezuner T, Linnarsson S | title = Single-cell sequencing-based technologies will revolutionize whole-organism science | journal = Nature Reviews. Genetics | volume = 14 | issue = 9 | pages = 618–30 | date = September 2013 | pmid = 23897237 | doi = 10.1038/nrg3542 | s2cid = 500845 }}"</ref><ref name="Kolodziejczyk">{{cite journal | vauthors = Kolodziejczyk AA, Kim JK, Svensson V, Marioni JC, Teichmann SA | title = The technology and biology of single-cell RNA sequencing | journal = Molecular Cell | volume = 58 | issue = 4 | pages = 610–20 | date = May 2015 | pmid = 26000846 | doi = 10.1016/j.molcel.2015.04.005 | doi-access = free }}</ref> | ||
Single-cell RNA sequencing (scRNA-Seq) provides the ] of individual cells. Although it is not possible to obtain complete information on every RNA expressed by each cell, due to the small amount of material available, patterns of gene expression can be identified through gene ]. This can uncover the existence of rare cell types within a cell population that may never have been seen before. For example, rare specialized cells in the lung called ] that express the ] were identified in 2018 by two groups performing scRNA-Seq on lung airway epithelia.<ref>{{cite journal | vauthors = Montoro DT, Haber AL, Biton M, Vinarsky V, Lin B, Birket SE, Yuan F, Chen S, Leung HM, Villoria J, Rogel N, Burgin G, Tsankov AM, Waghray A, Slyper M, Waldman J, Nguyen L, Dionne D, Rozenblatt-Rosen O, Tata PR, Mou H, Shivaraju M, Bihler H, Mense M, Tearney GJ, Rowe SM, Engelhardt JF, Regev A, Rajagopal J |
Single-cell RNA sequencing (scRNA-Seq) provides the ] of individual cells. Although it is not possible to obtain complete information on every RNA expressed by each cell, due to the small amount of material available, patterns of gene expression can be identified through gene ]. This can uncover the existence of rare cell types within a cell population that may never have been seen before. For example, rare specialized cells in the lung called ] that express the ] were identified in 2018 by two groups performing scRNA-Seq on lung airway epithelia.<ref>{{cite journal | vauthors = Montoro DT, Haber AL, Biton M, Vinarsky V, Lin B, Birket SE, Yuan F, Chen S, Leung HM, Villoria J, Rogel N, Burgin G, Tsankov AM, Waghray A, Slyper M, Waldman J, Nguyen L, Dionne D, Rozenblatt-Rosen O, Tata PR, Mou H, Shivaraju M, Bihler H, Mense M, Tearney GJ, Rowe SM, Engelhardt JF, Regev A, Rajagopal J | title = A revised airway epithelial hierarchy includes CFTR-expressing ionocytes | journal = Nature | volume = 560 | issue = 7718 | pages = 319–324 | date = August 2018 | pmid = 30069044 | pmc = 6295155 | doi = 10.1038/s41586-018-0393-7 | bibcode = 2018Natur.560..319M }}</ref><ref>{{cite journal | vauthors = Plasschaert LW, Žilionis R, Choo-Wing R, Savova V, Knehr J, Roma G, Klein AM, Jaffe AB | title = A single-cell atlas of the airway epithelium reveals the CFTR-rich pulmonary ionocyte | journal = Nature | volume = 560 | issue = 7718 | pages = 377–381 | date = August 2018 | pmid = 30069046 | pmc = 6108322 | doi = 10.1038/s41586-018-0394-6 | bibcode = 2018Natur.560..377P }}</ref> | ||
==== Experimental procedures ==== | ==== Experimental procedures ==== | ||
] | ] | ||
Current scRNA-Seq protocols involve the following steps: isolation of single cell and RNA, ] (RT), amplification, library generation and sequencing. Single cells are either mechanically separated into microwells (e.g., BD Rhapsody, Takara ICELL8, Vycap Puncher Platform, or CellMicrosystems CellRaft) or encapsulated in droplets (e.g., 10x Genomics Chromium, Illumina Bio-Rad ddSEQ, 1CellBio InDrop, Dolomite Bio Nadia).<ref>{{cite journal | vauthors = Valihrach L, Androvic P, Kubista M | title = Platforms for Single-Cell Collection and Analysis | journal = International Journal of Molecular Sciences | volume = 19 | issue = 3 | date = March 2018 | page = 807 | pmid = 29534489 | pmc = 5877668 | doi = 10.3390/ijms19030807 | doi-access = free }}</ref> Single cells are labeled by adding beads with barcoded oligonucleotides; both cells and beads are supplied in limited amounts such that co-occupancy with multiple cells and beads is a very rare event. Once reverse transcription is complete, the cDNAs from many cells can be mixed together for sequencing; transcripts from a particular cell are identified by each cell's unique barcode.<ref>{{cite journal | vauthors = Klein AM, Mazutis L, Akartuna I, Tallapragada N, Veres A, Li V, Peshkin L, Weitz DA, Kirschner MW |
Current scRNA-Seq protocols involve the following steps: isolation of single cell and RNA, ] (RT), amplification, library generation and sequencing. Single cells are either mechanically separated into microwells (e.g., BD Rhapsody, Takara ICELL8, Vycap Puncher Platform, or CellMicrosystems CellRaft) or encapsulated in droplets (e.g., 10x Genomics Chromium, Illumina Bio-Rad ddSEQ, 1CellBio InDrop, Dolomite Bio Nadia).<ref>{{cite journal | vauthors = Valihrach L, Androvic P, Kubista M | title = Platforms for Single-Cell Collection and Analysis | journal = International Journal of Molecular Sciences | volume = 19 | issue = 3 | date = March 2018 | page = 807 | pmid = 29534489 | pmc = 5877668 | doi = 10.3390/ijms19030807 | doi-access = free }}</ref> Single cells are labeled by adding beads with barcoded oligonucleotides; both cells and beads are supplied in limited amounts such that co-occupancy with multiple cells and beads is a very rare event. Once reverse transcription is complete, the cDNAs from many cells can be mixed together for sequencing; transcripts from a particular cell are identified by each cell's unique barcode.<ref>{{cite journal | vauthors = Klein AM, Mazutis L, Akartuna I, Tallapragada N, Veres A, Li V, Peshkin L, Weitz DA, Kirschner MW | title = Droplet barcoding for single-cell transcriptomics applied to embryonic stem cells | journal = Cell | volume = 161 | issue = 5 | pages = 1187–1201 | date = May 2015 | pmid = 26000487 | pmc = 4441768 | doi = 10.1016/j.cell.2015.04.044 }}</ref><ref>{{cite journal | vauthors = Macosko EZ, Basu A, Satija R, Nemesh J, Shekhar K, Goldman M, Tirosh I, Bialas AR, Kamitaki N, Martersteck EM, Trombetta JJ, Weitz DA, Sanes JR, Shalek AK, Regev A, McCarroll SA | title = Highly Parallel Genome-wide Expression Profiling of Individual Cells Using Nanoliter Droplets | journal = Cell | volume = 161 | issue = 5 | pages = 1202–1214 | date = May 2015 | pmid = 26000488 | pmc = 4481139 | doi = 10.1016/j.cell.2015.05.002 }}</ref> ] can be attached to mRNA/cDNA target sequences to help identify artifacts during library preparation.<ref>{{cite journal | vauthors = Islam S, Zeisel A, Joost S, La Manno G, Zajac P, Kasper M, Lönnerberg P, Linnarsson S | title = Quantitative single-cell RNA-seq with unique molecular identifiers | journal = Nature Methods | volume = 11 | issue = 2 | pages = 163–6 | date = February 2014 | pmid = 24363023 | doi = 10.1038/nmeth.2772 | s2cid = 6765530 }}</ref> | ||
Challenges for scRNA-Seq include preserving the initial relative abundance of mRNA in a cell and identifying rare transcripts.<ref name="Hebenstreit">"{{cite journal | vauthors = Hebenstreit D | title = Methods, Challenges and Potentials of Single Cell RNA-seq | journal = Biology | volume = 1 | issue = 3 | pages = 658–67 | date = November 2012 | pmid = 24832513 | pmc = 4009822 | doi = 10.3390/biology1030658 | doi-access = free }}"</ref> The reverse transcription step is critical as the efficiency of the RT reaction determines how much of the cell's RNA population will be eventually analyzed by the sequencer. The processivity of reverse transcriptases and the priming strategies used may affect full-length cDNA production and the generation of libraries biased toward the 3’ or 5' end of genes. | Challenges for scRNA-Seq include preserving the initial relative abundance of mRNA in a cell and identifying rare transcripts.<ref name="Hebenstreit">"{{cite journal | vauthors = Hebenstreit D | title = Methods, Challenges and Potentials of Single Cell RNA-seq | journal = Biology | volume = 1 | issue = 3 | pages = 658–67 | date = November 2012 | pmid = 24832513 | pmc = 4009822 | doi = 10.3390/biology1030658 | doi-access = free }}"</ref> The reverse transcription step is critical as the efficiency of the RT reaction determines how much of the cell's RNA population will be eventually analyzed by the sequencer. The processivity of reverse transcriptases and the priming strategies used may affect full-length cDNA production and the generation of libraries biased toward the 3’ or 5' end of genes. | ||
Line 64: | Line 68: | ||
In the amplification step, either PCR or ] transcription (IVT) is currently used to amplify cDNA. One of the advantages of PCR-based methods is the ability to generate full-length cDNA. However, different PCR efficiency on particular sequences (for instance, GC content and snapback structure) may also be exponentially amplified, producing libraries with uneven coverage. On the other hand, while libraries generated by IVT can avoid PCR-induced sequence bias, specific sequences may be transcribed inefficiently, thus causing sequence drop-out or generating incomplete sequences.<ref name=Eberwine>{{cite journal | vauthors = Eberwine J, Sul JY, Bartfai T, Kim J | title = The promise of single-cell sequencing | journal = Nature Methods | volume = 11 | issue = 1 | pages = 25–7 | date = January 2014 | pmid = 24524134 | doi = 10.1038/nmeth.2769 | s2cid = 11575439 }}</ref><ref name="Shapiro" /> | In the amplification step, either PCR or ] transcription (IVT) is currently used to amplify cDNA. One of the advantages of PCR-based methods is the ability to generate full-length cDNA. However, different PCR efficiency on particular sequences (for instance, GC content and snapback structure) may also be exponentially amplified, producing libraries with uneven coverage. On the other hand, while libraries generated by IVT can avoid PCR-induced sequence bias, specific sequences may be transcribed inefficiently, thus causing sequence drop-out or generating incomplete sequences.<ref name=Eberwine>{{cite journal | vauthors = Eberwine J, Sul JY, Bartfai T, Kim J | title = The promise of single-cell sequencing | journal = Nature Methods | volume = 11 | issue = 1 | pages = 25–7 | date = January 2014 | pmid = 24524134 | doi = 10.1038/nmeth.2769 | s2cid = 11575439 }}</ref><ref name="Shapiro" /> | ||
Several scRNA-Seq protocols have been published: | Several scRNA-Seq protocols have been published: | ||
Tang et al.,<ref>{{cite journal | vauthors = Tang F, Barbacioru C, Wang Y, Nordman E, Lee C, Xu N, Wang X, Bodeau J, Tuch BB, Siddiqui A, Lao K, Surani MA |
Tang et al.,<ref>{{cite journal | vauthors = Tang F, Barbacioru C, Wang Y, Nordman E, Lee C, Xu N, Wang X, Bodeau J, Tuch BB, Siddiqui A, Lao K, Surani MA | title = mRNA-Seq whole-transcriptome analysis of a single cell | journal = Nature Methods | volume = 6 | issue = 5 | pages = 377–82 | date = May 2009 | pmid = 19349980 | doi = 10.1038/NMETH.1315 | s2cid = 16570747 }}</ref> | ||
STRT,<ref>{{cite journal | vauthors = Islam S, Kjällquist U, Moliner A, Zajac P, Fan JB, Lönnerberg P, Linnarsson S | title = Characterization of the single-cell transcriptional landscape by highly multiplex RNA-seq | journal = Genome Research | volume = 21 | issue = 7 | pages = 1160–7 | date = July 2011 | pmid = 21543516 | pmc = 3129258 | doi = 10.1101/gr.110882.110 }}</ref> | STRT,<ref>{{cite journal | vauthors = Islam S, Kjällquist U, Moliner A, Zajac P, Fan JB, Lönnerberg P, Linnarsson S | title = Characterization of the single-cell transcriptional landscape by highly multiplex RNA-seq | journal = Genome Research | volume = 21 | issue = 7 | pages = 1160–7 | date = July 2011 | pmid = 21543516 | pmc = 3129258 | doi = 10.1101/gr.110882.110 }}</ref> | ||
SMART-seq,<ref>{{cite journal | vauthors = Ramsköld D, Luo S, Wang YC, Li R, Deng Q, Faridani OR, Daniels GA, Khrebtukova I, Loring JF, Laurent LC, Schroth GP, Sandberg R |
SMART-seq,<ref>{{cite journal | vauthors = Ramsköld D, Luo S, Wang YC, Li R, Deng Q, Faridani OR, Daniels GA, Khrebtukova I, Loring JF, Laurent LC, Schroth GP, Sandberg R | title = Full-length mRNA-Seq from single-cell levels of RNA and individual circulating tumor cells | journal = Nature Biotechnology | volume = 30 | issue = 8 | pages = 777–82 | date = August 2012 | pmid = 22820318 | pmc = 3467340 | doi = 10.1038/nbt.2282 }}</ref> | ||
CEL-seq,<ref>{{cite journal | vauthors = Hashimshony T, Wagner F, Sher N, Yanai I | title = CEL-Seq: single-cell RNA-Seq by multiplexed linear amplification | journal = Cell Reports | volume = 2 | issue = 3 | pages = 666–73 | date = September 2012 | pmid = 22939981 | doi = 10.1016/j.celrep.2012.08.003 | doi-access = free }}</ref> | CEL-seq,<ref>{{cite journal | vauthors = Hashimshony T, Wagner F, Sher N, Yanai I | title = CEL-Seq: single-cell RNA-Seq by multiplexed linear amplification | journal = Cell Reports | volume = 2 | issue = 3 | pages = 666–73 | date = September 2012 | pmid = 22939981 | doi = 10.1016/j.celrep.2012.08.003 | doi-access = free }}</ref> | ||
RAGE-seq,<ref>{{cite journal| vauthors = Singh M, Al-Eryani G, Carswell S, Ferguson JM, Blackburn J, Barton K, Roden D, Luciani F, Phan T, Junankar S, Jackson K, Goodnow CC, Smith MA, Swarbrick A | title=High-throughput targeted long-read single cell sequencing reveals the clonal and transcriptional landscape of lymphocytes |journal=bioRxiv|year=2018 | volume=10 | issue=1 | page=3120 |doi=10.1101/424945 | pmid=31311926 | pmc=6635368 |doi-access=free }}</ref> Quartz-seq<ref>{{cite journal | vauthors = Sasagawa Y, Nikaido I, Hayashi T, Danno H, Uno KD, Imai T, Ueda HR | title = Quartz-Seq: a highly reproducible and sensitive single-cell RNA sequencing method, reveals non-genetic gene-expression heterogeneity | journal = Genome Biology | volume = 14 | issue = 4 | pages = R31 | date = April 2013 | pmid = 23594475 | pmc = 4054835 | doi = 10.1186/gb-2013-14-4-r31 }}</ref> and C1-CAGE.<ref>{{cite journal | vauthors = Kouno T, Moody J, Kwon AT, Shibayama Y, Kato S, Huang Y, Böttcher M, Motakis E, Mendez M, Severin J, Luginbühl J, Abugessaisa I, Hasegawa A, Takizawa S, Arakawa T, Furuno M, Ramalingam N, West J, Suzuki H, Kasukawa T, Lassmann T, Hon CC, Arner E, Carninci P, Plessy C, Shin JW |
RAGE-seq,<ref>{{cite journal| vauthors = Singh M, Al-Eryani G, Carswell S, Ferguson JM, Blackburn J, Barton K, Roden D, Luciani F, Phan T, Junankar S, Jackson K, Goodnow CC, Smith MA, Swarbrick A | title=High-throughput targeted long-read single cell sequencing reveals the clonal and transcriptional landscape of lymphocytes |journal=bioRxiv|year=2018 | volume=10 | issue=1 | page=3120 |doi=10.1101/424945 | pmid=31311926 | pmc=6635368 |doi-access=free }}</ref> Quartz-seq<ref>{{cite journal | vauthors = Sasagawa Y, Nikaido I, Hayashi T, Danno H, Uno KD, Imai T, Ueda HR | title = Quartz-Seq: a highly reproducible and sensitive single-cell RNA sequencing method, reveals non-genetic gene-expression heterogeneity | journal = Genome Biology | volume = 14 | issue = 4 | pages = R31 | date = April 2013 | pmid = 23594475 | pmc = 4054835 | doi = 10.1186/gb-2013-14-4-r31 | doi-access = free }}</ref> and C1-CAGE.<ref>{{cite journal | vauthors = Kouno T, Moody J, Kwon AT, Shibayama Y, Kato S, Huang Y, Böttcher M, Motakis E, Mendez M, Severin J, Luginbühl J, Abugessaisa I, Hasegawa A, Takizawa S, Arakawa T, Furuno M, Ramalingam N, West J, Suzuki H, Kasukawa T, Lassmann T, Hon CC, Arner E, Carninci P, Plessy C, Shin JW | title = C1 CAGE detects transcription start sites and enhancer activity at single-cell resolution | journal = Nature Communications | volume = 10 | issue = 1 | pages = 360 | date = January 2019 | pmid = 30664627 | pmc = 6341120 | doi = 10.1038/s41467-018-08126-5 | bibcode = 2019NatCo..10..360K }}</ref> These protocols differ in terms of strategies for reverse transcription, cDNA synthesis and amplification, and the possibility to accommodate sequence-specific barcodes (i.e. ]) or the ability to process pooled samples.<ref>{{cite journal | vauthors = Dal Molin A, Di Camillo B | title = How to design a single-cell RNA-sequencing experiment: pitfalls, challenges and perspectives | journal = Briefings in Bioinformatics | volume = 20| issue = 4| pages = 1384–1394 | pmid = 29394315 | doi = 10.1093/bib/bby007 | year = 2019 }}</ref> | ||
In 2017, two approaches were introduced to simultaneously measure single-cell mRNA and protein expression through oligonucleotide-labeled antibodies known as REAP-seq,<ref>{{cite journal | vauthors = Peterson VM, Zhang KX, Kumar N, Wong J, Li L, Wilson DC, Moore R, McClanahan TK, Sadekova S, Klappenbach JA |
In 2017, two approaches were introduced to simultaneously measure single-cell mRNA and protein expression through oligonucleotide-labeled antibodies known as REAP-seq,<ref>{{cite journal | vauthors = Peterson VM, Zhang KX, Kumar N, Wong J, Li L, Wilson DC, Moore R, McClanahan TK, Sadekova S, Klappenbach JA | title = Multiplexed quantification of proteins and transcripts in single cells | journal = Nature Biotechnology | volume = 35 | issue = 10 | pages = 936–939 | date = October 2017 | pmid = 28854175 | doi = 10.1038/nbt.3973 | first8 = Namit | first9 = Kelvin Xi | first6 = Lixia | first7 = Jerelyn | s2cid = 205285357 }}</ref> and CITE-seq.<ref>{{cite journal | vauthors = Stoeckius M, Hafemeister C, Stephenson W, Houck-Loomis B, Chattopadhyay PK, Swerdlow H, Satija R, Smibert P | title = Simultaneous epitope and transcriptome measurement in single cells | journal = Nature Methods | volume = 14 | issue = 9 | pages = 865–868 | date = September 2017 | pmid = 28759029 | pmc = 5669064 | doi = 10.1038/nmeth.4380 | first8 = Marlon | first5 = Brian | first6 = William | first7 = Christoph }}</ref> | ||
==== Applications ==== | ==== Applications ==== | ||
scRNA-Seq is becoming widely used across biological disciplines including Development, ],<ref>{{cite journal | vauthors = Raj B, Wagner DE, McKenna A, Pandey S, Klein AM, Shendure J, Gagnon JA, Schier AF |
scRNA-Seq is becoming widely used across biological disciplines including Development, ],<ref>{{cite journal | vauthors = Raj B, Wagner DE, McKenna A, Pandey S, Klein AM, Shendure J, Gagnon JA, Schier AF | title = Simultaneous single-cell profiling of lineages and cell types in the vertebrate brain | journal = Nature Biotechnology | volume = 36 | issue = 5 | pages = 442–450 | date = June 2018 | pmid = 29608178 | pmc = 5938111 | doi = 10.1038/nbt.4103 }}</ref> ],<ref>{{cite journal | vauthors = Olmos D, Arkenau HT, Ang JE, Ledaki I, Attard G, Carden CP, Reid AH, A'Hern R, Fong PC, Oomen NB, Molife R, Dearnaley D, Parker C, Terstappen LW, de Bono JS | title = Circulating tumour cell (CTC) counts as intermediate end points in castration-resistant prostate cancer (CRPC): a single-centre experience | journal = Annals of Oncology | volume = 20 | issue = 1 | pages = 27–33 | date = January 2009 | pmid = 18695026 | doi = 10.1093/annonc/mdn544 | doi-access = free }}</ref><ref>{{cite journal | vauthors = Levitin HM, Yuan J, Sims PA | title = Single-Cell Transcriptomic Analysis of Tumor Heterogeneity | language = en | journal = Trends in Cancer | volume = 4 | issue = 4 | pages = 264–268 | date = April 2018 | pmid = 29606308 | pmc = 5993208 | doi = 10.1016/j.trecan.2018.02.003 | url = }}</ref><ref>{{cite journal | vauthors = Jerby-Arnon L, Shah P, Cuoco MS, Rodman C, Su MJ, Melms JC, Leeson R, Kanodia A, Mei S, Lin JR, Wang S, Rabasha B, Liu D, Zhang G, Margolais C, Ashenberg O, Ott PA, Buchbinder EI, Haq R, Hodi FS, Boland GM, Sullivan RJ, Frederick DT, Miao B, Moll T, Flaherty KT, Herlyn M, Jenkins RW, Thummalapalli R, Kowalczyk MS, Cañadas I, Schilling B, Cartwright AN, Luoma AM, Malu S, Hwu P, Bernatchez C, Forget MA, Barbie DA, Shalek AK, Tirosh I, Sorger PK, Wucherpfennig K, Van Allen EM, Schadendorf D, Johnson BE, Rotem A, Rozenblatt-Rosen O, Garraway LA, Yoon CH, Izar B, Regev A | title = A Cancer Cell Program Promotes T Cell Exclusion and Resistance to Checkpoint Blockade | language = en | journal = Cell | volume = 175 | issue = 4 | pages = 984–997.e24 | date = November 2018 | pmid = 30388455 | pmc = 6410377 | doi = 10.1016/j.cell.2018.09.006 | url = }}</ref> ],<ref>{{cite journal | vauthors = Stephenson W, Donlin LT, Butler A, Rozo C, Bracken B, Rashidfarrokhi A, Goodman SM, Ivashkiv LB, Bykerk VP, Orange DE, Darnell RB, Swerdlow HP, Satija R | title = Single-cell RNA-seq of rheumatoid arthritis synovial tissue using low-cost microfluidic instrumentation | journal = Nature Communications | volume = 9 | issue = 1 | pages = 791 | date = February 2018 | pmid = 29476078 | pmc = 5824814 | doi = 10.1038/s41467-017-02659-x | bibcode = 2018NatCo...9..791S }}</ref> and ].<ref>{{cite journal | vauthors = Avraham R, Haseley N, Brown D, Penaranda C, Jijon HB, Trombetta JJ, Satija R, Shalek AK, Xavier RJ, Regev A, Hung DT | title = Pathogen Cell-to-Cell Variability Drives Heterogeneity in Host Immune Responses | journal = Cell | volume = 162 | issue = 6 | pages = 1309–21 | date = September 2015 | pmid = 26343579 | pmc = 4578813 | doi = 10.1016/j.cell.2015.08.027 }}</ref> | ||
scRNA-Seq has provided considerable insight into the development of embryos and organisms, including the worm '']'',<ref>{{cite journal | vauthors = Cao J, Packer JS, Ramani V, Cusanovich DA, Huynh C, Daza R, Qiu X, Lee C, Furlan SN, Steemers FJ, Adey A, Waterston RH, Trapnell C, Shendure J |
scRNA-Seq has provided considerable insight into the development of embryos and organisms, including the worm '']'',<ref>{{cite journal | vauthors = Cao J, Packer JS, Ramani V, Cusanovich DA, Huynh C, Daza R, Qiu X, Lee C, Furlan SN, Steemers FJ, Adey A, Waterston RH, Trapnell C, Shendure J | title = Comprehensive single-cell transcriptional profiling of a multicellular organism | journal = Science | volume = 357 | issue = 6352 | pages = 661–667 | date = August 2017 | pmid = 28818938 | pmc = 5894354 | doi = 10.1126/science.aam8940 | bibcode = 2017Sci...357..661C }}</ref> and the regenerative planarian '']''.<ref>{{cite journal | vauthors = Plass M, Solana J, Wolf FA, Ayoub S, Misios A, Glažar P, Obermayer B, Theis FJ, Kocks C, Rajewsky N | title = Cell type atlas and lineage tree of a whole complex animal by single-cell transcriptomics | journal = Science | volume = 360 | issue = 6391 | pages = eaaq1723 | date = May 2018 | pmid = 29674432 | doi = 10.1126/science.aaq1723 | doi-access = free }}</ref><ref>{{cite journal | vauthors = Fincher CT, Wurtzel O, de Hoog T, Kravarik KM, Reddien PW | title = Schmidtea mediterranea | journal = Science | volume = 360 | issue = 6391 | pages = eaaq1736 | date = May 2018 | pmid = 29674431 | pmc = 6563842 | doi = 10.1126/science.aaq1736 }}</ref> The first vertebrate animals to be mapped in this way were ]<ref>{{cite journal | vauthors = Wagner DE, Weinreb C, Collins ZM, Briggs JA, Megason SG, Klein AM | title = Single-cell mapping of gene expression landscapes and lineage in the zebrafish embryo | journal = Science | volume = 360 | issue = 6392 | pages = 981–987 | date = June 2018 | pmid = 29700229 | pmc = 6083445 | doi = 10.1126/science.aar4362 | bibcode = 2018Sci...360..981W }}</ref><ref>{{cite journal | vauthors = Farrell JA, Wang Y, Riesenfeld SJ, Shekhar K, Regev A, Schier AF | title = Single-cell reconstruction of developmental trajectories during zebrafish embryogenesis | journal = Science | volume = 360 | issue = 6392 | pages = eaar3131 | date = June 2018 | pmid = 29700225 | pmc = 6247916 | doi = 10.1126/science.aar3131 }}</ref> and '']''.<ref>{{cite journal | vauthors = Briggs JA, Weinreb C, Wagner DE, Megason S, Peshkin L, Kirschner MW, Klein AM | title = The dynamics of gene expression in vertebrate embryogenesis at single-cell resolution | journal = Science | volume = 360 | issue = 6392 | pages = eaar5780 | date = June 2018 | pmid = 29700227 | pmc = 6038144 | doi = 10.1126/science.aar5780 }}</ref> In each case multiple stages of the embryo were studied, allowing the entire process of development to be mapped on a cell-by-cell basis.<ref name="ReferenceA" /> ] recognized these advances as the 2018 ].<ref>{{cite web |url= https://vis.sciencemag.org/breakthrough2018/finalists/ |title=Science's 2018 Breakthrough of the Year: tracking development cell by cell| vauthors = You J | work = Science Magazine | publisher = American Association for the Advancement of Science }}</ref> | ||
===Experimental considerations=== | ===Experimental considerations=== | ||
Line 81: | Line 85: | ||
A variety of ] are considered when designing and conducting RNA-Seq experiments: | A variety of ] are considered when designing and conducting RNA-Seq experiments: | ||
* ''Tissue specificity:'' Gene expression varies within and between tissues, and RNA-Seq measures this mix of cell types. This may make it difficult to isolate the biological mechanism of interest. ] can be used to study each cell individually, mitigating this issue. | * ''Tissue specificity:'' Gene expression varies within and between tissues, and RNA-Seq measures this mix of cell types. This may make it difficult to isolate the biological mechanism of interest. ] can be used to study each cell individually, mitigating this issue. | ||
* ''Time dependence:'' Gene expression changes over time, and RNA-Seq only takes a snapshot. Time course experiments can be performed to observe changes in the transcriptome. | * ''Time dependence:'' Gene expression changes over time, and RNA-Seq only takes a snapshot. Time course experiments can be performed to observe changes in the transcriptome. | ||
* ''Coverage (also known as depth):'' RNA harbors the same mutations observed in DNA, and detection requires deeper coverage. With high enough coverage, RNA-Seq can be used to estimate the expression of each allele. This may provide insight into phenomena such as ] or ]. The depth of sequencing required for specific applications can be extrapolated from a pilot experiment.<ref name="li2008" /> | * ''Coverage (also known as depth):'' RNA harbors the same mutations observed in DNA, and detection requires deeper coverage. With high enough coverage, RNA-Seq can be used to estimate the expression of each allele. This may provide insight into phenomena such as ] or ]. The depth of sequencing required for specific applications can be extrapolated from a pilot experiment.<ref name="li2008" /> | ||
Line 89: | Line 93: | ||
==Analysis== | ==Analysis== | ||
{{See also|List of RNA-Seq bioinformatics tools}} | {{See also|List of RNA-Seq bioinformatics tools}} | ||
] | ] | ||
===Transcriptome assembly=== | ===Transcriptome assembly=== | ||
Line 97: | Line 101: | ||
Two methods are used to assign raw sequence reads to genomic features (i.e., assemble the transcriptome): | Two methods are used to assign raw sequence reads to genomic features (i.e., assemble the transcriptome): | ||
* ''De novo:'' This approach does not require a ] to reconstruct the transcriptome, and is typically used if the genome is unknown, incomplete, or substantially altered compared to the reference.<ref name="ReferenceB">{{cite journal | vauthors = Grabherr MG, Haas BJ, Yassour M, Levin JZ, Thompson DA, Amit I, Adiconis X, Fan L, Raychowdhury R, Zeng Q, Chen Z, Mauceli E, Hacohen N, Gnirke A, Rhind N, di Palma F, Birren BW, Nusbaum C, Lindblad-Toh K, Friedman N, Regev A |
* ''De novo:'' This approach does not require a ] to reconstruct the transcriptome, and is typically used if the genome is unknown, incomplete, or substantially altered compared to the reference.<ref name="ReferenceB">{{cite journal | vauthors = Grabherr MG, Haas BJ, Yassour M, Levin JZ, Thompson DA, Amit I, Adiconis X, Fan L, Raychowdhury R, Zeng Q, Chen Z, Mauceli E, Hacohen N, Gnirke A, Rhind N, di Palma F, Birren BW, Nusbaum C, Lindblad-Toh K, Friedman N, Regev A | title = Full-length transcriptome assembly from RNA-Seq data without a reference genome | journal = Nature Biotechnology | volume = 29 | issue = 7 | pages = 644–52 | date = May 2011 | pmid = 21572440 | pmc = 3571712 | doi = 10.1038/nbt.1883 }}</ref> Challenges when using short reads for de novo assembly include 1) determining which reads should be joined together into contiguous sequences (]s), 2) robustness to sequencing errors and other artifacts, and 3) computational efficiency. The primary algorithm used for de novo assembly transitioned from overlap graphs, which identify all pair-wise overlaps between reads, to ]s, which break reads into sequences of length k and collapse all k-mers into a hash table.<ref>{{cite web|title=De Novo Assembly Using Illumina Reads |url= http://www.illumina.com/Documents/products/technotes/technote_denovo_assembly_ecoli.pdf |access-date=22 October 2016 }}</ref> Overlap graphs were used with Sanger sequencing, but do not scale well to the millions of reads generated with RNA-Seq. Examples of assemblers that use de Bruijn graphs are Trinity,<ref name="ReferenceB"/> Oases<ref></ref> (derived from the genome assembler ]<ref>{{cite journal | vauthors = Zerbino DR, Birney E | title = Velvet: algorithms for de novo short read assembly using de Bruijn graphs | journal = Genome Research | volume = 18 | issue = 5 | pages = 821–9 | date = May 2008 | pmid = 18349386 | pmc = 2336801 | doi = 10.1101/gr.074492.107 }}</ref>), Bridger,<ref>{{cite journal | vauthors = Chang Z, Li G, Liu J, Zhang Y, Ashby C, Liu D, Cramer CL, Huang X | title = Bridger: a new framework for de novo transcriptome assembly using RNA-seq data | journal = Genome Biology | volume = 16 | issue = 1 | pages = 30 | date = February 2015 | pmid = 25723335 | pmc = 4342890 | doi = 10.1186/s13059-015-0596-2 | doi-access = free }}</ref> and rnaSPAdes.<ref>{{cite journal | vauthors = Bushmanova E, Antipov D, Lapidus A, Prjibelski AD | title = rnaSPAdes: a de novo transcriptome assembler and its application to RNA-Seq data | journal = GigaScience | volume = 8 | issue = 9 | date = September 2019 | pmid = 31494669 | pmc = 6736328 | doi = 10.1093/gigascience/giz100 }}</ref> Paired-end and long-read sequencing of the same sample can mitigate the deficits in short read sequencing by serving as a template or skeleton. Metrics to assess the quality of a de novo assembly include median contig length, number of contigs and ].<ref name="ReferenceC">{{cite journal | vauthors = Li B, Fillmore N, Bai Y, Collins M, Thomson JA, Stewart R, Dewey CN | title = Evaluation of de novo transcriptome assemblies from RNA-Seq data | journal = Genome Biology | volume = 15 | issue = 12 | pages = 553 | date = December 2014 | pmid = 25608678 | pmc = 4298084 | doi = 10.1186/s13059-014-0553-5 | doi-access = free }}</ref> | ||
] | ] | ||
* ''Genome guided:'' This approach relies on the same methods used for DNA alignment, with the additional complexity of aligning reads that cover non-continuous portions of the reference genome.<ref name="2012 STAR aligner">{{cite journal | vauthors = Dobin A, Davis CA, Schlesinger F, Drenkow J, Zaleski C, Jha S, Batut P, Chaisson M, Gingeras TR |
* ''Genome guided:'' This approach relies on the same methods used for DNA alignment, with the additional complexity of aligning reads that cover non-continuous portions of the reference genome.<ref name="2012 STAR aligner">{{cite journal | vauthors = Dobin A, Davis CA, Schlesinger F, Drenkow J, Zaleski C, Jha S, Batut P, Chaisson M, Gingeras TR | title = STAR: ultrafast universal RNA-seq aligner | journal = Bioinformatics | volume = 29 | issue = 1 | pages = 15–21 | date = January 2013 | pmid = 23104886 | pmc = 3530905 | doi = 10.1093/bioinformatics/bts635 }}</ref> These non-continuous reads are the result of sequencing spliced transcripts (see figure). Typically, alignment algorithms have two steps: 1) align short portions of the read (i.e., seed the genome), and 2) use ] to find an optimal alignment, sometimes in combination with known annotations. Software tools that use genome-guided alignment include ],<ref>{{cite journal | vauthors = ], Trapnell C, Pop M, Salzberg SL | title = Ultrafast and memory-efficient alignment of short DNA sequences to the human genome | journal = Genome Biology | volume = 10 | issue = 3 | pages = R25 | date = 2009 | pmid = 19261174 | pmc = 2690996 | doi = 10.1186/gb-2009-10-3-r25 | doi-access = free }}</ref> ] (which builds on BowTie results to align splice junctions),<ref>{{cite journal | vauthors = Trapnell C, ], ] | title = TopHat: discovering splice junctions with RNA-Seq | journal = Bioinformatics | volume = 25 | issue = 9 | pages = 1105–11 | date = May 2009 | pmid = 19289445 | pmc = 2672628 | doi = 10.1093/bioinformatics/btp120 }}</ref><ref name="Differential gene and transcript ex">{{cite journal | vauthors = Trapnell C, Roberts A, Goff L, Pertea G, Kim D, Kelley DR, Pimentel H, Salzberg SL, Rinn JL, Pachter L | title = Differential gene and transcript expression analysis of RNA-seq experiments with TopHat and Cufflinks | journal = Nature Protocols | volume = 7 | issue = 3 | pages = 562–78 | date = March 2012 | pmid = 22383036 | pmc = 3334321 | doi = 10.1038/nprot.2012.016 }}</ref> Subread,<ref>{{cite journal | vauthors = Liao Y, Smyth GK, Shi W | title = The Subread aligner: fast, accurate and scalable read mapping by seed-and-vote | journal = Nucleic Acids Research | volume = 41 | issue = 10 | pages = e108 | date = May 2013 | pmid = 23558742 | pmc = 3664803 | doi = 10.1093/nar/gkt214 }}</ref> STAR,<ref name="2012 STAR aligner" /> HISAT2,<ref>{{cite journal | vauthors = Kim D, Langmead B, Salzberg SL | title = HISAT: a fast spliced aligner with low memory requirements | journal = Nature Methods | volume = 12 | issue = 4 | pages = 357–60 | date = April 2015 | pmid = 25751142 | pmc = 4655817 | doi = 10.1038/nmeth.3317 }}</ref> and GMAP.<ref>{{cite journal | vauthors = Wu TD, Watanabe CK | title = GMAP: a genomic mapping and alignment program for mRNA and EST sequences | journal = Bioinformatics | volume = 21 | issue = 9 | pages = 1859–75 | date = May 2005 | pmid = 15728110 | doi = 10.1093/bioinformatics/bti310 | doi-access = free }}</ref> The output of genome guided alignment (mapping) tools can be further used by tools such as Cufflinks<ref name="Differential gene and transcript ex"/> or StringTie<ref>{{cite journal | vauthors = Pertea M, Pertea GM, Antonescu CM, Chang TC, Mendell JT, Salzberg SL | title = StringTie enables improved reconstruction of a transcriptome from RNA-seq reads | journal = Nature Biotechnology | volume = 33 | issue = 3 | pages = 290–5 | date = March 2015 | pmid = 25690850 | pmc = 4643835 | doi = 10.1038/nbt.3122 }}</ref> to reconstruct contiguous transcript sequences (''i.e.'', a FASTA file). The quality of a genome guided assembly can be measured with both 1) de novo assembly metrics (e.g., N50) and 2) comparisons to known transcript, splice junction, genome, and protein sequences using ], or their combination (e.g., F1 score).<ref name="ReferenceC"/> In addition, ''in silico'' assessment could be performed using simulated reads.<ref>{{cite journal | vauthors = Baruzzo G, Hayer KE, Kim EJ, Di Camillo B, FitzGerald GA, Grant GR | title = Simulation-based comprehensive benchmarking of RNA-seq aligners | language = En | journal = Nature Methods | volume = 14 | issue = 2 | pages = 135–139 | date = February 2017 | pmid = 27941783 | pmc = 5792058 | doi = 10.1038/nmeth.4106 }}</ref><ref>{{cite journal | vauthors = Engström PG, Steijger T, Sipos B, Grant GR, Kahles A, Rätsch G, Goldman N, Hubbard TJ, Harrow J, Guigó R, Bertone P | title = Systematic evaluation of spliced alignment programs for RNA-seq data | language = En | journal = Nature Methods | volume = 10 | issue = 12 | pages = 1185–91 | date = December 2013 | pmid = 24185836 | pmc = 4018468 | doi = 10.1038/nmeth.2722 }}</ref> | ||
''A note on assembly quality:'' The current consensus is that 1) assembly quality can vary depending on which metric is used, 2) assembly tools that scored well in one species do not necessarily perform well in the other species, and 3) combining different approaches might be the most reliable.<ref>{{cite journal | vauthors = Lu B, Zeng Z, Shi T | title = Comparative study of de novo assembly and genome-guided assembly strategies for transcriptome reconstruction based on RNA-Seq | journal = Science China Life Sciences | volume = 56 | issue = 2 | pages = 143–55 | date = February 2013 | pmid = 23393030 | doi = 10.1007/s11427-013-4442-z | doi-access = free }}</ref><ref>{{cite journal | vauthors = Bradnam KR, Fass JN, Alexandrov A, Baranay P, Bechner M, Birol I, Boisvert S, Chapman JA, Chapuis G, Chikhi R, Chitsaz H, Chou WC, Corbeil J, Del Fabbro C, Docking TR, Durbin R, Earl D, Emrich S, Fedotov P, Fonseca NA, Ganapathy G, Gibbs RA, Gnerre S, Godzaridis E, Goldstein S, Haimel M, Hall G, Haussler D, Hiatt JB, Ho IY, Howard J, Hunt M, Jackman SD, Jaffe DB, Jarvis ED, Jiang H, Kazakov S, Kersey PJ, Kitzman JO, Knight JR, Koren S, Lam TW, Lavenier D, Laviolette F, Li Y, Li Z, Liu B, Liu Y, Luo R, Maccallum I, Macmanes MD, Maillet N, Melnikov S, Naquin D, Ning Z, Otto TD, Paten B, Paulo OS, Phillippy AM, Pina-Martins F, Place M, Przybylski D, Qin X, Qu C, Ribeiro FJ, Richards S, Rokhsar DS, Ruby JG, Scalabrin S, Schatz MC, Schwartz DC, Sergushichev A, Sharpe T, Shaw TI, Shendure J, Shi Y, Simpson JT, Song H, Tsarev F, Vezzi F, Vicedomini R, Vieira BM, Wang J, Worley KC, Yin S, Yiu SM, Yuan J, Zhang G, Zhang H, Zhou S, Korf IF |
''A note on assembly quality:'' The current consensus is that 1) assembly quality can vary depending on which metric is used, 2) assembly tools that scored well in one species do not necessarily perform well in the other species, and 3) combining different approaches might be the most reliable.<ref>{{cite journal | vauthors = Lu B, Zeng Z, Shi T | title = Comparative study of de novo assembly and genome-guided assembly strategies for transcriptome reconstruction based on RNA-Seq | journal = Science China Life Sciences | volume = 56 | issue = 2 | pages = 143–55 | date = February 2013 | pmid = 23393030 | doi = 10.1007/s11427-013-4442-z | doi-access = free }}</ref><ref>{{cite journal | vauthors = Bradnam KR, Fass JN, Alexandrov A, Baranay P, Bechner M, Birol I, Boisvert S, Chapman JA, Chapuis G, Chikhi R, Chitsaz H, Chou WC, Corbeil J, Del Fabbro C, Docking TR, Durbin R, Earl D, Emrich S, Fedotov P, Fonseca NA, Ganapathy G, Gibbs RA, Gnerre S, Godzaridis E, Goldstein S, Haimel M, Hall G, Haussler D, Hiatt JB, Ho IY, Howard J, Hunt M, Jackman SD, Jaffe DB, Jarvis ED, Jiang H, Kazakov S, Kersey PJ, Kitzman JO, Knight JR, Koren S, Lam TW, Lavenier D, Laviolette F, Li Y, Li Z, Liu B, Liu Y, Luo R, Maccallum I, Macmanes MD, Maillet N, Melnikov S, Naquin D, Ning Z, Otto TD, Paten B, Paulo OS, Phillippy AM, Pina-Martins F, Place M, Przybylski D, Qin X, Qu C, Ribeiro FJ, Richards S, Rokhsar DS, Ruby JG, Scalabrin S, Schatz MC, Schwartz DC, Sergushichev A, Sharpe T, Shaw TI, Shendure J, Shi Y, Simpson JT, Song H, Tsarev F, Vezzi F, Vicedomini R, Vieira BM, Wang J, Worley KC, Yin S, Yiu SM, Yuan J, Zhang G, Zhang H, Zhou S, Korf IF | title = Assemblathon 2: evaluating de novo methods of genome assembly in three vertebrate species | journal = GigaScience | volume = 2 | issue = 1 | pages = 10 | date = July 2013 | pmid = 23870653 | pmc = 3844414 | doi = 10.1186/2047-217X-2-10 | bibcode = 2013arXiv1301.5406B | arxiv = 1301.5406 | doi-access = free }}</ref><ref>{{cite journal | vauthors = Hölzer M, Marz M | title = De novo transcriptome assembly: A comprehensive cross-species comparison of short-read RNA-Seq assemblers | journal = GigaScience | volume = 8 | issue = 5 | date = May 2019 | pmid = 31077315 | pmc = 6511074 | doi = 10.1093/gigascience/giz039 }}</ref> | ||
===Gene expression quantification=== | ===Gene expression quantification=== | ||
Expression is quantified to study cellular changes in response to external stimuli, differences between healthy and ] states, and other research questions. Transcript levels are often used as a proxy for protein abundance, but these are often not equivalent due to post transcriptional events such as ] and ].<ref name=greenbaum2003 >{{cite journal | vauthors = Greenbaum D, Colangelo C, Williams K, Gerstein M | title = Comparing protein abundance and mRNA expression levels on a genomic scale | journal = Genome Biology | volume = 4 | issue = 9 | pages = 117 | year = 2003 | pmid = 12952525 | pmc = 193646 | doi = 10.1186/gb-2003-4-9-117 }}</ref> | Expression is quantified to study cellular changes in response to external stimuli, differences between healthy and ] states, and other research questions. Transcript levels are often used as a proxy for protein abundance, but these are often not equivalent due to post transcriptional events such as ] and ].<ref name=greenbaum2003 >{{cite journal | vauthors = Greenbaum D, Colangelo C, Williams K, Gerstein M | title = Comparing protein abundance and mRNA expression levels on a genomic scale | journal = Genome Biology | volume = 4 | issue = 9 | pages = 117 | year = 2003 | pmid = 12952525 | pmc = 193646 | doi = 10.1186/gb-2003-4-9-117 | doi-access = free }}</ref> | ||
Expression is quantified by counting the number of reads that mapped to each locus in the ] step. Expression can be quantified for exons or genes using contigs or reference transcript annotations.<ref name="ReferenceA"/> These observed RNA-Seq read counts have been robustly validated against older technologies, including expression microarrays and ].<ref name=li2008>{{cite journal | vauthors = Li H, Lovci MT, Kwon YS, Rosenfeld MG, Fu XD, Yeo GW | title = Determination of tag density required for digital transcriptome analysis: application to an androgen-sensitive prostate cancer model | journal = Proceedings of the National Academy of Sciences of the United States of America | volume = 105 | issue = 51 | pages = 20179–84 | date = December 2008 | pmid = 19088194 | pmc = 2603435 | doi = 10.1073/pnas.0807121105 | bibcode = 2008PNAS..10520179L }}</ref><ref>{{cite journal | vauthors = Zhang ZH, Jhaveri DJ, Marshall VM, Bauer DC, Edson J, Narayanan RK, Robinson GJ, Lundberg AE, Bartlett PF, Wray NR, Zhao QY |
Expression is quantified by counting the number of reads that mapped to each locus in the ] step. Expression can be quantified for exons or genes using contigs or reference transcript annotations.<ref name="ReferenceA"/> These observed RNA-Seq read counts have been robustly validated against older technologies, including expression microarrays and ].<ref name=li2008>{{cite journal | vauthors = Li H, Lovci MT, Kwon YS, Rosenfeld MG, Fu XD, Yeo GW | title = Determination of tag density required for digital transcriptome analysis: application to an androgen-sensitive prostate cancer model | journal = Proceedings of the National Academy of Sciences of the United States of America | volume = 105 | issue = 51 | pages = 20179–84 | date = December 2008 | pmid = 19088194 | pmc = 2603435 | doi = 10.1073/pnas.0807121105 | bibcode = 2008PNAS..10520179L | doi-access = free }}</ref><ref>{{cite journal | vauthors = Zhang ZH, Jhaveri DJ, Marshall VM, Bauer DC, Edson J, Narayanan RK, Robinson GJ, Lundberg AE, Bartlett PF, Wray NR, Zhao QY | title = A comparative study of techniques for differential expression analysis on RNA-Seq data | journal = PLOS ONE | volume = 9 | issue = 8 | pages = e103207 | date = August 2014 | pmid = 25119138 | doi = 10.1371/journal.pone.0103207 | pmc=4132098| bibcode = 2014PLoSO...9j3207Z | doi-access = free }}</ref> Tools that quantify counts are HTSeq,<ref>{{cite journal | vauthors = Anders S, Pyl PT, Huber W | title = HTSeq--a Python framework to work with high-throughput sequencing data | journal = Bioinformatics | volume = 31 | issue = 2 | pages = 166–9 | date = January 2015 | pmid = 25260700 | pmc = 4287950 | doi = 10.1093/bioinformatics/btu638 }}</ref> FeatureCounts,<ref>{{cite journal | vauthors = Liao Y, Smyth GK, Shi W | title = featureCounts: an efficient general purpose program for assigning sequence reads to genomic features | journal = Bioinformatics | volume = 30 | issue = 7 | pages = 923–30 | date = April 2014 | pmid = 24227677 | doi = 10.1093/bioinformatics/btt656 | arxiv = 1305.3347 }}</ref> Rcount,<ref>{{cite journal | vauthors = Schmid MW, Grossniklaus U | title = Rcount: simple and flexible RNA-Seq read counting | journal = Bioinformatics | volume = 31 | issue = 3 | pages = 436–7 | date = February 2015 | pmid = 25322836 | doi = 10.1093/bioinformatics/btu680 | doi-access = free }}</ref> maxcounts,<ref>{{cite journal | vauthors = Finotello F, Lavezzo E, Bianco L, Barzon L, Mazzon P, Fontana P, Toppo S, Di Camillo B | title = Reducing bias in RNA sequencing data: a novel approach to compute counts | journal = BMC Bioinformatics | volume = 15 | issue = Suppl 1 | pages = S7 | date = 2014 | pmid = 24564404 | pmc = 4016203 | doi = 10.1186/1471-2105-15-s1-s7 | doi-access = free }}</ref> FIXSEQ,<ref>{{cite journal | vauthors = Hashimoto TB, Edwards MD, Gifford DK | title = Universal count correction for high-throughput sequencing | journal = PLOS Computational Biology | volume = 10 | issue = 3 | pages = e1003494 | date = March 2014 | pmid = 24603409 | pmc = 3945112 | doi = 10.1371/journal.pcbi.1003494 | bibcode = 2014PLSCB..10E3494H | doi-access = free }}</ref> and Cuffquant. These tools determine read counts from aligned RNA-Seq data, but alignment-free counts can also be obtained with Sailfish<ref>{{cite journal|date=May 2014|title=Sailfish enables alignment-free isoform quantification from RNA-seq reads using lightweight algorithms|journal=Nature Biotechnology|volume=32|issue=5|pages=462–4|arxiv=1308.3700|doi=10.1038/nbt.2862|pmc=4077321|pmid=24752080|vauthors=Patro R, Mount SM, Kingsford C}}</ref> and Kallisto.<ref>{{cite journal|date=May 2016|title=Near-optimal probabilistic RNA-seq quantification|journal=Nature Biotechnology|volume=34|issue=5|pages=525–7|doi=10.1038/nbt.3519|pmid=27043002|vauthors=Bray NL, Pimentel H, Melsted P, Pachter L|s2cid=205282743|url=https://resolver.caltech.edu/CaltechAUTHORS:20190506-110012992 }}</ref> The read counts are then converted into appropriate metrics for hypothesis testing, regressions, and other analyses. Parameters for this conversion are: | ||
* '']:'' Although depth is pre-specified when conducting multiple RNA-Seq experiments, it will still vary widely between experiments.<ref name="ReferenceD">{{cite journal | |
* '']:'' Although depth is pre-specified when conducting multiple RNA-Seq experiments, it will still vary widely between experiments.<ref name="ReferenceD">{{cite journal | author1 = Robinson MD| author2 = Oshlack A | author-link2 = Alicia Oshlack | title = A scaling normalization method for differential expression analysis of RNA-seq data | journal = Genome Biology | volume = 11 | issue = 3 | pages = R25 | date = 2010 | pmid = 20196867 | pmc = 2864565 | doi = 10.1186/gb-2010-11-3-r25 | doi-access = free }}</ref> Therefore, the total number of reads generated in a single experiment is typically normalized by converting counts to fragments, reads, or counts per million mapped reads (FPM, RPM, or CPM). The difference between RPM and FPM was historically derived during the evolution from single-end sequencing of fragments to paired-end sequencing. In single-end sequencing, there is only one read per fragment (''i.e.'', RPM = FPM). In paired-end sequencing, there are two reads per fragment (''i.e.'', RPM = 2 x FPM). Sequencing depth is sometimes referred to as ], the number of intermediary cDNA molecules in the experiment. | ||
* ''Gene length:'' Longer genes will have more fragments/reads/counts than shorter genes if transcript expression is the same. This is adjusted by dividing the FPM by the length of a feature (which can be a gene, transcript, or exon), resulting in the metric fragments per kilobase of feature per million mapped reads (FPKM).<ref name="trapnell2010">{{cite journal | vauthors = Trapnell C, Williams BA, Pertea G, Mortazavi A, Kwan G, van Baren MJ, Salzberg SL, Wold BJ, Pachter L | title = Transcript assembly and quantification by RNA-Seq reveals unannotated transcripts and isoform switching during cell differentiation | journal = Nature Biotechnology | volume = 28 | issue = 5 | pages = 511–5 | date = May 2010 | pmid = 20436464 | pmc = 3146043 | doi = 10.1038/nbt.1621 | author9-link = Lior Pachter }}</ref> When looking at groups of features across samples, FPKM is converted to transcripts per million (TPM) by dividing each FPKM by the sum of FPKMs within a sample.<ref name = "Pachter_2011">{{cite |
* ''Gene length:'' Longer genes will have more fragments/reads/counts than shorter genes if transcript expression is the same. This is adjusted by dividing the FPM by the length of a feature (which can be a gene, transcript, or exon), resulting in the metric fragments per kilobase of feature per million mapped reads (FPKM).<ref name="trapnell2010">{{cite journal | vauthors = Trapnell C, Williams BA, Pertea G, Mortazavi A, Kwan G, van Baren MJ, Salzberg SL, Wold BJ, Pachter L | title = Transcript assembly and quantification by RNA-Seq reveals unannotated transcripts and isoform switching during cell differentiation | journal = Nature Biotechnology | volume = 28 | issue = 5 | pages = 511–5 | date = May 2010 | pmid = 20436464 | pmc = 3146043 | doi = 10.1038/nbt.1621 | author9-link = Lior Pachter }}</ref> When looking at groups of features across samples, FPKM is converted to transcripts per million (TPM) by dividing each FPKM by the sum of FPKMs within a sample.<ref name = "Pachter_2011">{{cite arXiv| vauthors = Pachter L |title=Models for transcript quantification from RNA-Seq|eprint=1104.3889|date=19 April 2011|class=q-bio.GN}}</ref><ref>{{cite web|title=What the FPKM? A review of RNA-Seq expression units|url=https://haroldpimentel.wordpress.com/2014/05/08/what-the-fpkm-a-review-rna-seq-expression-units/|website=The farrago|access-date=28 March 2018|date=8 May 2014}}</ref><ref>{{cite journal | vauthors = Wagner GP, Kin K, Lynch VJ | title = Measurement of mRNA abundance using RNA-seq data: RPKM measure is inconsistent among samples | journal = Theory in Biosciences | volume = 131 | issue = 4 | pages = 281–5 | date = December 2012 | pmid = 22872506 | doi = 10.1007/s12064-012-0162-3 | s2cid = 16752581 }}</ref> | ||
* ''Total sample RNA output:'' Because the same amount of RNA is extracted from each sample, samples with more total RNA will have less RNA per gene. These genes appear to have decreased expression, resulting in false positives in downstream analyses.<ref name="ReferenceD"/> Normalization strategies including quantile, DESeq2, TMM and Median Ratio attempt to account for this difference by comparing a set of non-differentially expressed genes between samples and scaling accordingly.<ref>{{cite journal | vauthors = Evans C, Hardin J, Stoebel DM | title = Selecting between-sample RNA-Seq normalization methods from the perspective of their assumptions | journal = Briefings in Bioinformatics | volume = 19 | issue = 5 | pages = 776–792 | date = September 2018 | pmid = 28334202 | pmc = 6171491 | doi = 10.1093/bib/bbx008 }}</ref> | * ''Total sample RNA output:'' Because the same amount of RNA is extracted from each sample, samples with more total RNA will have less RNA per gene. These genes appear to have decreased expression, resulting in false positives in downstream analyses.<ref name="ReferenceD"/> Normalization strategies including quantile, DESeq2, TMM and Median Ratio attempt to account for this difference by comparing a set of non-differentially expressed genes between samples and scaling accordingly.<ref>{{cite journal | vauthors = Evans C, Hardin J, Stoebel DM | title = Selecting between-sample RNA-Seq normalization methods from the perspective of their assumptions | journal = Briefings in Bioinformatics | volume = 19 | issue = 5 | pages = 776–792 | date = September 2018 | pmid = 28334202 | pmc = 6171491 | doi = 10.1093/bib/bbx008 }}</ref> | ||
* ''] for each gene's expression:'' is modeled to account for ] (important for genes with low read counts), increase power, and decrease false positives. Variance can be estimated as a ], ], or ] distribution<ref name="voom: Precision weights unlock line">{{cite journal | vauthors = Law CW, Chen Y, Shi W, Smyth GK | title = voom: Precision weights unlock linear model analysis tools for RNA-seq read counts | journal = Genome Biology | volume = 15 | issue = 2 | pages = R29 | date = February 2014 | pmid = 24485249 | pmc = 4053721 | doi = 10.1186/gb-2014-15-2-r29 }}</ref><ref name="Differential expression analysis fo">{{cite journal | vauthors = Anders S, Huber W | title = Differential expression analysis for sequence count data | journal = Genome Biology | volume = 11 | issue = 10 | pages = R106 | date = 2010 | pmid = 20979621 | pmc = 3218662 | doi = 10.1186/gb-2010-11-10-r106 }}</ref><ref name="edgeR: a Bioconductor package for d">{{cite journal | vauthors = Robinson MD, McCarthy DJ, Smyth GK | title = edgeR: a Bioconductor package for differential expression analysis of digital gene expression data | journal = Bioinformatics | volume = 26 | issue = 1 | pages = 139–40 | date = January 2010 | pmid = 19910308 | pmc = 2796818 | doi = 10.1093/bioinformatics/btp616 }}</ref> and is frequently decomposed into technical and biological variance. | * ''] for each gene's expression:'' is modeled to account for ] (important for genes with low read counts), increase power, and decrease false positives. Variance can be estimated as a ], ], or ] distribution<ref name="voom: Precision weights unlock line">{{cite journal | vauthors = Law CW, Chen Y, Shi W, Smyth GK | title = voom: Precision weights unlock linear model analysis tools for RNA-seq read counts | journal = Genome Biology | volume = 15 | issue = 2 | pages = R29 | date = February 2014 | pmid = 24485249 | pmc = 4053721 | doi = 10.1186/gb-2014-15-2-r29 | doi-access = free }}</ref><ref name="Differential expression analysis fo">{{cite journal | vauthors = Anders S, Huber W | title = Differential expression analysis for sequence count data | journal = Genome Biology | volume = 11 | issue = 10 | pages = R106 | date = 2010 | pmid = 20979621 | pmc = 3218662 | doi = 10.1186/gb-2010-11-10-r106 | doi-access = free }}</ref><ref name="edgeR: a Bioconductor package for d">{{cite journal | vauthors = Robinson MD, McCarthy DJ, Smyth GK | title = edgeR: a Bioconductor package for differential expression analysis of digital gene expression data | journal = Bioinformatics | volume = 26 | issue = 1 | pages = 139–40 | date = January 2010 | pmid = 19910308 | pmc = 2796818 | doi = 10.1093/bioinformatics/btp616 }}</ref> and is frequently decomposed into technical and biological variance. | ||
==== Spike-ins for absolute quantification and detection of genome-wide effects ==== | ==== Spike-ins for absolute quantification and detection of genome-wide effects ==== | ||
] are samples of RNA at known concentrations that can be used as gold standards in experimental design and during downstream analyses for absolute quantification and detection of genome-wide effects. | ] are samples of RNA at known concentrations that can be used as gold standards in experimental design and during downstream analyses for absolute quantification and detection of genome-wide effects. | ||
* ''Absolute quantification:'' Absolute quantification of gene expression is not possible with most RNA-Seq experiments, which quantify expression relative to all transcripts. It is possible by performing RNA-Seq with spike-ins, samples of RNA at known concentrations. After sequencing, read counts of spike-in sequences are used to determine the relationship between each gene's read counts and absolute quantities of biological fragments<ref name="mortazavi2008"/><ref>{{cite journal | vauthors = Marguerat S, Schmidt A, Codlin S, Chen W, Aebersold R, Bähler J | title = Quantitative analysis of fission yeast transcriptomes and proteomes in proliferating and quiescent cells | journal = Cell | volume = 151 | issue = 3 | pages = 671–83 | date = October 2012 | pmid = 23101633 | pmc = 3482660 | doi = 10.1016/j.cell.2012.09.019 }}</ref> In one example, this technique was used in '']'' embryos to determine transcription kinetics.<ref>{{cite journal | vauthors = Owens ND, Blitz IL, Lane MA, Patrushev I, Overton JD, Gilchrist MJ, Cho KW, Khokha MK | title = Measuring Absolute RNA Copy Numbers at High Temporal Resolution Reveals Transcriptome Kinetics in Development | journal = Cell Reports | volume = 14 | issue = 3 | pages = 632–647 | date = January 2016 | pmid = 26774488 | pmc = 4731879 | doi = 10.1016/j.celrep.2015.12.050 }}</ref> | * ''Absolute quantification:'' Absolute quantification of gene expression is not possible with most RNA-Seq experiments, which quantify expression relative to all transcripts. It is possible by performing RNA-Seq with spike-ins, samples of RNA at known concentrations. After sequencing, read counts of spike-in sequences are used to determine the relationship between each gene's read counts and absolute quantities of biological fragments.<ref name="mortazavi2008"/><ref>{{cite journal | vauthors = Marguerat S, Schmidt A, Codlin S, Chen W, Aebersold R, Bähler J | title = Quantitative analysis of fission yeast transcriptomes and proteomes in proliferating and quiescent cells | journal = Cell | volume = 151 | issue = 3 | pages = 671–83 | date = October 2012 | pmid = 23101633 | pmc = 3482660 | doi = 10.1016/j.cell.2012.09.019 }}</ref> In one example, this technique was used in '']'' embryos to determine transcription kinetics.<ref>{{cite journal | vauthors = Owens ND, Blitz IL, Lane MA, Patrushev I, Overton JD, Gilchrist MJ, Cho KW, Khokha MK | title = Measuring Absolute RNA Copy Numbers at High Temporal Resolution Reveals Transcriptome Kinetics in Development | journal = Cell Reports | volume = 14 | issue = 3 | pages = 632–647 | date = January 2016 | pmid = 26774488 | pmc = 4731879 | doi = 10.1016/j.celrep.2015.12.050 }}</ref> | ||
* ''Detection of genome-wide effects:'' Changes in global regulators including chromatin remodelers, transcription factors (e.g., MYC), acetyltransferase complexes, and nucleosome positioning are not congruent with normalization assumptions and spike-in controls can offer precise interpretation.<ref>{{cite journal | vauthors = Chen K, Hu Z, Xia Z, Zhao D, Li W, Tyler JK | title = The Overlooked Fact: Fundamental Need for Spike-In Control for Virtually All Genome-Wide Analyses | journal = Molecular and Cellular Biology | volume = 36 | issue = 5 | pages = 662–7 | date = December 2015 | pmid = 26711261 | pmc = 4760223 | doi = 10.1128/MCB.00970-14 }}</ref><ref>{{cite journal | vauthors = Lovén J, Orlando DA, Sigova AA, Lin CY, Rahl PB, Burge CB, Levens DL, Lee TI, Young RA |
* ''Detection of genome-wide effects:'' Changes in global regulators including ], ] (e.g., ]), ] complexes, and nucleosome positioning are not congruent with normalization assumptions and spike-in controls can offer precise interpretation.<ref>{{cite journal | vauthors = Chen K, Hu Z, Xia Z, Zhao D, Li W, Tyler JK | title = The Overlooked Fact: Fundamental Need for Spike-In Control for Virtually All Genome-Wide Analyses | journal = Molecular and Cellular Biology | volume = 36 | issue = 5 | pages = 662–7 | date = December 2015 | pmid = 26711261 | pmc = 4760223 | doi = 10.1128/MCB.00970-14 }}</ref><ref>{{cite journal | vauthors = Lovén J, Orlando DA, Sigova AA, Lin CY, Rahl PB, Burge CB, Levens DL, Lee TI, Young RA | title = Revisiting global gene expression analysis | journal = Cell | volume = 151 | issue = 3 | pages = 476–82 | date = October 2012 | pmid = 23101621 | pmc = 3505597 | doi = 10.1016/j.cell.2012.10.012 }}</ref> | ||
=== Differential expression === | === Differential expression === | ||
The simplest but often most powerful use of RNA-Seq is finding differences in gene expression between two or more conditions (''e.g.'', treated vs not treated); this process is called differential expression. The outputs are frequently referred to as differentially expressed genes (DEGs) and these genes can either be up- or down-regulated (''i.e.'', higher or lower in the condition of interest). There are many ]. Most are run in ], ], or the ] command line. Commonly used tools include DESeq,<ref name="Differential expression analysis fo"/> edgeR,<ref name="edgeR: a Bioconductor package for d"/> and voom+limma,<ref name="voom: Precision weights unlock line"/><ref>{{cite journal | vauthors = Ritchie ME, Phipson B, Wu D, Hu Y, Law CW, Shi W, Smyth GK | title = limma powers differential expression analyses for RNA-sequencing and microarray studies | journal = Nucleic Acids Research | volume = 43 | issue = 7 | pages = e47 | date = April 2015 | pmid = 25605792 | pmc = 4402510 | doi = 10.1093/nar/gkv007 }}</ref> all of which are available through R/].<ref>{{cite web | url = http://www.bioconductor.org | title = Bioconductor - Open source software for bioinformatics}}</ref><ref>{{cite journal | vauthors = Huber W, Carey VJ, Gentleman R, Anders S, Carlson M, Carvalho BS, Bravo HC, Davis S, Gatto L, Girke T, Gottardo R, Hahne F, Hansen KD, Irizarry RA, Lawrence M, Love MI, MacDonald J, Obenchain V, Oleś AK, Pagès H, Reyes A, Shannon P, Smyth GK, Tenenbaum D, Waldron L, Morgan M |
The simplest but often most powerful use of RNA-Seq is finding differences in gene expression between two or more conditions (''e.g.'', treated vs not treated); this process is called differential expression. The outputs are frequently referred to as differentially expressed genes (DEGs) and these genes can either be up- or down-regulated (''i.e.'', higher or lower in the condition of interest). There are many ]. Most are run in ], ], or the ] command line. Commonly used tools include DESeq,<ref name="Differential expression analysis fo"/> edgeR,<ref name="edgeR: a Bioconductor package for d"/> and voom+limma,<ref name="voom: Precision weights unlock line"/><ref>{{cite journal | vauthors = Ritchie ME, Phipson B, Wu D, Hu Y, Law CW, Shi W, Smyth GK | title = limma powers differential expression analyses for RNA-sequencing and microarray studies | journal = Nucleic Acids Research | volume = 43 | issue = 7 | pages = e47 | date = April 2015 | pmid = 25605792 | pmc = 4402510 | doi = 10.1093/nar/gkv007 }}</ref> all of which are available through R/].<ref>{{cite web | url = http://www.bioconductor.org | title = Bioconductor - Open source software for bioinformatics}}</ref><ref>{{cite journal | vauthors = Huber W, Carey VJ, Gentleman R, Anders S, Carlson M, Carvalho BS, Bravo HC, Davis S, Gatto L, Girke T, Gottardo R, Hahne F, Hansen KD, Irizarry RA, Lawrence M, Love MI, MacDonald J, Obenchain V, Oleś AK, Pagès H, Reyes A, Shannon P, Smyth GK, Tenenbaum D, Waldron L, Morgan M | title = Orchestrating high-throughput genomic analysis with Bioconductor | journal = Nature Methods | volume = 12 | issue = 2 | pages = 115–21 | date = February 2015 | pmid = 25633503 | pmc = 4509590 | doi = 10.1038/nmeth.3252 }}</ref> These are the common considerations when performing differential expression: | ||
* ''Inputs:'' Differential expression inputs include (1) an RNA-Seq expression matrix (M genes x N samples) and (2) a ] containing experimental conditions for N samples. The simplest design matrix contains one column, corresponding to labels for the condition being tested. Other covariates (also referred to as factors, features, labels, or parameters) can include ]s, known artifacts, and any metadata that might confound or mediate gene expression. In addition to known covariates, unknown covariates can also be estimated through ] approaches including ], surrogate variable,<ref>{{cite journal | vauthors = Leek JT, Storey JD | title = Capturing heterogeneity in gene expression studies by surrogate variable analysis | journal = PLOS Genetics | volume = 3 | issue = 9 | pages = 1724–35 | date = September 2007 | pmid = 17907809 | pmc = 1994707 | doi = 10.1371/journal.pgen.0030161 }}</ref> and PEER<ref name="Using probabilistic estimation of e"/> analyses. Hidden variable analyses are often employed for human tissue RNA-Seq data, which typically have additional artifacts not captured in the metadata (''e.g.'', ischemic time, sourcing from multiple institutions, underlying clinical traits, collecting data across many years with many personnel). | * ''Inputs:'' Differential expression inputs include (1) an RNA-Seq expression matrix (M genes x N samples) and (2) a ] containing experimental conditions for N samples. The simplest design matrix contains one column, corresponding to labels for the condition being tested. Other covariates (also referred to as factors, features, labels, or parameters) can include ]s, known artifacts, and any metadata that might confound or mediate gene expression. In addition to known covariates, unknown covariates can also be estimated through ] approaches including ], surrogate variable,<ref>{{cite journal | vauthors = Leek JT, Storey JD | title = Capturing heterogeneity in gene expression studies by surrogate variable analysis | journal = PLOS Genetics | volume = 3 | issue = 9 | pages = 1724–35 | date = September 2007 | pmid = 17907809 | pmc = 1994707 | doi = 10.1371/journal.pgen.0030161 | doi-access = free }}</ref> and PEER<ref name="Using probabilistic estimation of e"/> analyses. Hidden variable analyses are often employed for human tissue RNA-Seq data, which typically have additional artifacts not captured in the metadata (''e.g.'', ischemic time, sourcing from multiple institutions, underlying clinical traits, collecting data across many years with many personnel). | ||
* ''Methods:'' Most tools use ] or ] to identify differentially expressed genes, and are either based on read counts mapped to a reference genome (DESeq2, limma, edgeR) or based on read counts derived from alignment-free quantification (sleuth,<ref>{{cite journal | vauthors = Pimentel H, Bray NL, Puente S, Melsted P, Pachter L | title = Differential analysis of RNA-seq incorporating quantification uncertainty | journal = Nature Methods | volume = 14 | issue = 7 | pages = 687–690 | date = July 2017 | pmid = 28581496 | doi = 10.1038/nmeth.4324 | s2cid = 15063247 | url = https://resolver.caltech.edu/CaltechAUTHORS:20170612-084553487 }}</ref> Cuffdiff,<ref>{{cite journal | vauthors = Trapnell C, Hendrickson DG, Sauvageau M, Goff L, Rinn JL, Pachter L | title = Differential analysis of gene regulation at transcript resolution with RNA-seq | journal = Nature Biotechnology | volume = 31 | issue = 1 | pages = 46–53 | date = January 2013 | pmid = 23222703 | doi = 10.1038/nbt.2450 | pmc = 3869392 }}</ref> Ballgown<ref>{{cite journal | vauthors = Frazee AC, Pertea G, Jaffe AE, Langmead B, Salzberg SL, Leek JT | title = Ballgown bridges the gap between transcriptome assembly and expression analysis | journal = Nature Biotechnology | volume = 33 | issue = 3 | pages = 243–6 | date = March 2015 | pmid = 25748911 | doi = 10.1038/nbt.3172 | pmc = 4792117 }}</ref>).<ref name = "Sahraeian_2017">{{cite journal | vauthors = Sahraeian SM, Mohiyuddin M, Sebra R, Tilgner H, Afshar PT, Au KF, Bani Asadi N, Gerstein MB, Wong WH, Snyder MP, Schadt E, Lam HY |
* ''Methods:'' Most tools use ] or ] to identify differentially expressed genes, and are either based on read counts mapped to a reference genome (DESeq2, limma, edgeR) or based on read counts derived from alignment-free quantification (sleuth,<ref>{{cite journal | vauthors = Pimentel H, Bray NL, Puente S, Melsted P, Pachter L | title = Differential analysis of RNA-seq incorporating quantification uncertainty | journal = Nature Methods | volume = 14 | issue = 7 | pages = 687–690 | date = July 2017 | pmid = 28581496 | doi = 10.1038/nmeth.4324 | s2cid = 15063247 | url = https://resolver.caltech.edu/CaltechAUTHORS:20170612-084553487 }}</ref> Cuffdiff,<ref>{{cite journal | vauthors = Trapnell C, Hendrickson DG, Sauvageau M, Goff L, Rinn JL, Pachter L | title = Differential analysis of gene regulation at transcript resolution with RNA-seq | journal = Nature Biotechnology | volume = 31 | issue = 1 | pages = 46–53 | date = January 2013 | pmid = 23222703 | doi = 10.1038/nbt.2450 | pmc = 3869392 }}</ref> Ballgown<ref>{{cite journal | vauthors = Frazee AC, Pertea G, Jaffe AE, Langmead B, Salzberg SL, Leek JT | title = Ballgown bridges the gap between transcriptome assembly and expression analysis | journal = Nature Biotechnology | volume = 33 | issue = 3 | pages = 243–6 | date = March 2015 | pmid = 25748911 | doi = 10.1038/nbt.3172 | pmc = 4792117 }}</ref>).<ref name = "Sahraeian_2017">{{cite journal | vauthors = Sahraeian SM, Mohiyuddin M, Sebra R, Tilgner H, Afshar PT, Au KF, Bani Asadi N, Gerstein MB, Wong WH, Snyder MP, Schadt E, Lam HY | title = Gaining comprehensive biological insight into the transcriptome by performing a broad-spectrum RNA-seq analysis | journal = Nature Communications | volume = 8 | issue = 1 | pages = 59 | date = July 2017 | pmid = 28680106 | doi = 10.1038/s41467-017-00050-4 | pmc = 5498581 | bibcode = 2017NatCo...8...59S }}</ref> Following regression, most tools employ either ] or ] p-value adjustments to account for ] (in human studies, ~20,000 protein-coding genes or ~50,000 biotypes). | ||
* ''Outputs:'' A typical output consists of rows corresponding to the number of genes and at least three columns, each gene's log ] (] of the ratio in expression between conditions, a measure of ]), ], and p-value adjusted for ]. Genes are defined as biologically meaningful if they pass cut-offs for effect size (log fold change) and ]. These cut-offs should ideally be specified ''a priori'', but the nature of RNA-Seq experiments is often exploratory so it is difficult to predict effect sizes and pertinent cut-offs ahead of time. | * ''Outputs:'' A typical output consists of rows corresponding to the number of genes and at least three columns, each gene's log ] (] of the ratio in expression between conditions, a measure of ]), ], and p-value adjusted for ]. Genes are defined as biologically meaningful if they pass cut-offs for effect size (log fold change) and ]. These cut-offs should ideally be specified ''a priori'', but the nature of RNA-Seq experiments is often exploratory so it is difficult to predict effect sizes and pertinent cut-offs ahead of time. | ||
* ''Pitfalls:'' The raison d'etre for these complex methods is to avoid the myriad of pitfalls that can lead to ] and misleading interpretations. Pitfalls include increased false positive rates (due to multiple comparisons), sample preparation artifacts, sample heterogeneity (like mixed genetic backgrounds), highly correlated samples, unaccounted for ], and poor ]. One notable pitfall is viewing results in Microsoft Excel without |
* ''Pitfalls:'' The raison d'etre for these complex methods is to avoid the myriad of pitfalls that can lead to ] and misleading interpretations. Pitfalls include increased false positive rates (due to multiple comparisons), sample preparation artifacts, sample heterogeneity (like mixed genetic backgrounds), highly correlated samples, unaccounted for ], and poor ]. One notable pitfall is viewing results in Microsoft Excel without using the import feature to ensure that the gene names remain text.<ref>{{cite journal | vauthors = Ziemann M, Eren Y, El-Osta A | title = Gene name errors are widespread in the scientific literature | journal = Genome Biology | volume = 17 | issue = 1 | pages = 177 | date = August 2016 | pmid = 27552985 | pmc = 4994289 | doi = 10.1186/s13059-016-1044-7 | doi-access = free }}</ref> Although convenient, Excel automatically converts some gene names (''], ], ]'') into dates or floating point numbers. | ||
* ''Choice of tools and benchmarking:'' There are numerous efforts that compare the results of these tools, with DESeq2 tending to moderately outperform other methods.<ref>{{cite journal | vauthors = Soneson C, Delorenzi M | title = A comparison of methods for differential expression analysis of RNA-seq data | journal = BMC Bioinformatics | volume = 14 | pages = 91 | date = March 2013 | pmid = 23497356 | pmc = 3608160 | doi = 10.1186/1471-2105-14-91 }}</ref><ref>{{cite journal | vauthors = Fonseca NA, Marioni J, Brazma A | title = RNA-Seq gene profiling--a systematic empirical comparison | journal = PLOS ONE | volume = 9 | issue = 9 | pages = e107026 | date = 30 September 2014 | pmid = 25268973 | pmc = 4182317 | doi = 10.1371/journal.pone.0107026 | bibcode = 2014PLoSO...9j7026F }}</ref><ref>{{cite journal | vauthors = Seyednasrollah F, Laiho A, Elo LL | title = Comparison of software packages for detecting differential expression in RNA-seq studies | journal = Briefings in Bioinformatics | volume = 16 | issue = 1 | pages = 59–70 | date = January 2015 | pmid = 24300110 | pmc = 4293378 | doi = 10.1093/bib/bbt086 }}</ref><ref>{{cite journal | vauthors = Rapaport F, Khanin R, Liang Y, Pirun M, Krek A, Zumbo P, Mason CE, Socci ND, Betel D |
* ''Choice of tools and benchmarking:'' There are numerous efforts that compare the results of these tools, with DESeq2 tending to moderately outperform other methods.<ref>{{cite journal | vauthors = Soneson C, Delorenzi M | title = A comparison of methods for differential expression analysis of RNA-seq data | journal = BMC Bioinformatics | volume = 14 | pages = 91 | date = March 2013 | pmid = 23497356 | pmc = 3608160 | doi = 10.1186/1471-2105-14-91 | doi-access = free }}</ref><ref>{{cite journal | vauthors = Fonseca NA, Marioni J, Brazma A | title = RNA-Seq gene profiling--a systematic empirical comparison | journal = PLOS ONE | volume = 9 | issue = 9 | pages = e107026 | date = 30 September 2014 | pmid = 25268973 | pmc = 4182317 | doi = 10.1371/journal.pone.0107026 | bibcode = 2014PLoSO...9j7026F | doi-access = free }}</ref><ref>{{cite journal | vauthors = Seyednasrollah F, Laiho A, Elo LL | title = Comparison of software packages for detecting differential expression in RNA-seq studies | journal = Briefings in Bioinformatics | volume = 16 | issue = 1 | pages = 59–70 | date = January 2015 | pmid = 24300110 | pmc = 4293378 | doi = 10.1093/bib/bbt086 }}</ref><ref>{{cite journal | vauthors = Rapaport F, Khanin R, Liang Y, Pirun M, Krek A, Zumbo P, Mason CE, Socci ND, Betel D | title = Comprehensive evaluation of differential gene expression analysis methods for RNA-seq data | journal = Genome Biology | volume = 14 | issue = 9 | pages = R95 | date = 2013 | pmid = 24020486 | pmc = 4054597 | doi = 10.1186/gb-2013-14-9-r95 | doi-access = free }}</ref><ref name="A survey of best practices for RNA"/><ref name = "Sahraeian_2017" /><ref>{{cite journal | vauthors = Costa-Silva J, Domingues D, Lopes FM | title = RNA-Seq differential expression analysis: An extended review and a software tool | journal = PLOS ONE | volume = 12 | issue = 12 | pages = e0190152 | date = 21 December 2017 | pmid = 29267363 | pmc = 5739479 | doi = 10.1371/journal.pone.0190152 | bibcode = 2017PLoSO..1290152C | doi-access = free }}</ref><ref>{{cite journal | vauthors = Corchete LA, Rojas EA, Alonso-López D, De Las Rivas J, Gutiérrez NC, Burguillo FJ | title = Systematic comparison and assessment of RNA-seq procedures for gene expression quantitative analysis | journal = Scientific Reports | volume = 12 | issue = 10 | pages = 19737 | date = 12 November 2020 | pmid = 33184454 | pmc = 7665074 | doi = 10.1038/s41598-020-76881-x | bibcode = 2020NatSR..1019737C | doi-access = free }}</ref> As with other methods, benchmarking consists of comparing tool outputs to each other and known ]. | ||
Downstream analyses for a list of differentially expressed genes come in two flavors, validating observations and making biological inferences. Owing to the pitfalls of differential expression and RNA-Seq, important observations are replicated with (1) an orthogonal method in the same samples (like ]) or (2) another, sometimes ], experiment in a new cohort. The latter helps ensure generalizability and can typically be followed up with a meta-analysis of all the pooled cohorts. The most common method for obtaining higher-level biological understanding of the results is ], although sometimes candidate gene approaches are employed. Gene set enrichment determines if the overlap between two gene sets is statistically significant, in this case the overlap between differentially expressed genes and gene sets from known pathways/databases (''e.g.'', ], ], ]) or from complementary analyses in the same data (like co-expression networks). Common tools for gene set enrichment include web interfaces (''e.g.'', |
Downstream analyses for a list of differentially expressed genes come in two flavors, validating observations and making biological inferences. Owing to the pitfalls of differential expression and RNA-Seq, important observations are replicated with (1) an orthogonal method in the same samples (like ]) or (2) another, sometimes ], experiment in a new cohort. The latter helps ensure generalizability and can typically be followed up with a meta-analysis of all the pooled cohorts. The most common method for obtaining higher-level biological understanding of the results is ], although sometimes candidate gene approaches are employed. Gene set enrichment determines if the overlap between two gene sets is statistically significant, in this case the overlap between differentially expressed genes and gene sets from known pathways/databases (''e.g.'', ], ], ]) or from complementary analyses in the same data (like co-expression networks). Common tools for gene set enrichment include web interfaces (''e.g.'', ENRICHR, g:profiler, WEBGESTALT)<ref>{{cite journal | vauthors = Liao Y, Wang J, Jaehnig EJ, Shi Z, Zhang B | title = WebGestalt 2019: gene set analysis toolkit with revamped UIs and APIs | journal = Nucleic Acids Research | volume = 47 | issue = W1 | pages = W199–W205 | date = July 2019 | pmid = 31114916 | pmc = 6602449 | doi = 10.1093/nar/gkz401 }}</ref> and software packages. When evaluating enrichment results, one heuristic is to first look for enrichment of known biology as a sanity check and then expand the scope to look for novel biology. | ||
[[file:Alt splicing bestiary2.jpg|thumb|Examples of alternative RNA splicing modes. Exons are represented as blue and yellow blocks, spliced introns as horizontal black lines connecting two exons, and exon-exon junctions as thin grey connecting lines between two exons. | [[file:Alt splicing bestiary2.jpg|thumb|Examples of alternative RNA splicing modes. Exons are represented as blue and yellow blocks, spliced introns as horizontal black lines connecting two exons, and exon-exon junctions as thin grey connecting lines between two exons. | ||
Line 139: | Line 143: | ||
===Alternative splicing=== | ===Alternative splicing=== | ||
] is integral to eukaryotes and contributes significantly to protein regulation and diversity, occurring in >90% of human genes.<ref name="Alternative splicing and evolution">{{cite journal | vauthors = Keren H, Lev-Maor G, Ast G | title = Alternative splicing and evolution: diversification, exon definition and function | journal = Nature Reviews. Genetics | volume = 11 | issue = 5 | pages = 345–55 | date = May 2010 | pmid = 20376054 | doi = 10.1038/nrg2776 | s2cid = 5184582 }}</ref> There are multiple ]: exon skipping (most common splicing mode in humans and higher eukaryotes), mutually exclusive exons, alternative donor or acceptor sites, intron retention (most common splicing mode in plants, fungi, and protozoa), alternative transcription start site (promoter), and alternative polyadenylation.<ref name="Alternative splicing and evolution"/> One goal of RNA-Seq is to identify alternative splicing events and test if they differ between conditions. Long-read sequencing captures the full transcript and thus minimizes many of issues in estimating isoform abundance, like ambiguous read mapping. For short-read RNA-Seq, there are multiple methods to detect alternative splicing that can be classified into three main groups:<ref>{{cite journal | vauthors = Liu R, Loraine AE, Dickerson JA | title = Comparisons of computational methods for differential alternative splicing detection using RNA-seq in plant systems | journal = BMC Bioinformatics | volume = 15 | issue = 1 | pages = 364 | date = December 2014 | pmid = 25511303 | pmc = 4271460 | doi = 10.1186/s12859-014-0364-4 }}</ref><ref name = "Pachter_2011" /><ref name="Annotation-free quantification of R">{{cite journal | vauthors = Li YI, Knowles DA, Humphrey J, Barbeira AN, Dickinson SP, Im HK, Pritchard JK | title = Annotation-free quantification of RNA splicing using LeafCutter | journal = Nature Genetics | volume = 50 | issue = 1 | pages = 151–158 | date = January 2018 | pmid = 29229983 | pmc = 5742080 | doi = 10.1038/s41588-017-0004-9 }}</ref> | ] is integral to eukaryotes and contributes significantly to protein regulation and diversity, occurring in >90% of human genes.<ref name="Alternative splicing and evolution">{{cite journal | vauthors = Keren H, Lev-Maor G, Ast G | title = Alternative splicing and evolution: diversification, exon definition and function | journal = Nature Reviews. Genetics | volume = 11 | issue = 5 | pages = 345–55 | date = May 2010 | pmid = 20376054 | doi = 10.1038/nrg2776 | s2cid = 5184582 }}</ref> There are multiple ]: exon skipping (most common splicing mode in humans and higher eukaryotes), mutually exclusive exons, alternative donor or acceptor sites, intron retention (most common splicing mode in plants, fungi, and protozoa), alternative transcription start site (promoter), and alternative polyadenylation.<ref name="Alternative splicing and evolution"/> One goal of RNA-Seq is to identify alternative splicing events and test if they differ between conditions. Long-read sequencing captures the full transcript and thus minimizes many of issues in estimating isoform abundance, like ambiguous read mapping. For short-read RNA-Seq, there are multiple methods to detect alternative splicing that can be classified into three main groups:<ref>{{cite journal | vauthors = Liu R, Loraine AE, Dickerson JA | title = Comparisons of computational methods for differential alternative splicing detection using RNA-seq in plant systems | journal = BMC Bioinformatics | volume = 15 | issue = 1 | pages = 364 | date = December 2014 | pmid = 25511303 | pmc = 4271460 | doi = 10.1186/s12859-014-0364-4 | doi-access = free }}</ref><ref name = "Pachter_2011" /><ref name="Annotation-free quantification of R">{{cite journal | vauthors = Li YI, Knowles DA, Humphrey J, Barbeira AN, Dickinson SP, Im HK, Pritchard JK | title = Annotation-free quantification of RNA splicing using LeafCutter | journal = Nature Genetics | volume = 50 | issue = 1 | pages = 151–158 | date = January 2018 | pmid = 29229983 | pmc = 5742080 | doi = 10.1038/s41588-017-0004-9 }}</ref> | ||
* ''Count-based (also event-based, differential splicing):'' estimate exon retention. Examples are DEXSeq,<ref>{{cite journal | vauthors = Anders S, Reyes A, Huber W | title = Detecting differential usage of exons from RNA-seq data | journal = Genome Research | volume = 22 | issue = 10 | pages = 2008–17 | date = October 2012 | pmid = 22722343 | pmc = 3460195 | doi = 10.1101/gr.133744.111 }}</ref> MATS,<ref>{{cite journal | vauthors = Shen S, Park JW, Huang J, Dittmar KA, Lu ZX, Zhou Q, Carstens RP, Xing Y |
* ''Count-based (also event-based, differential splicing):'' estimate exon retention. Examples are DEXSeq,<ref>{{cite journal | vauthors = Anders S, Reyes A, Huber W | title = Detecting differential usage of exons from RNA-seq data | journal = Genome Research | volume = 22 | issue = 10 | pages = 2008–17 | date = October 2012 | pmid = 22722343 | pmc = 3460195 | doi = 10.1101/gr.133744.111 }}</ref> MATS,<ref>{{cite journal | vauthors = Shen S, Park JW, Huang J, Dittmar KA, Lu ZX, Zhou Q, Carstens RP, Xing Y | title = MATS: a Bayesian framework for flexible detection of differential alternative splicing from RNA-Seq data | journal = Nucleic Acids Research | volume = 40 | issue = 8 | pages = e61 | date = April 2012 | pmid = 22266656 | pmc = 3333886 | doi = 10.1093/nar/gkr1291 }}</ref> and SeqGSEA.<ref>{{cite journal | vauthors = Wang X, Cairns MJ | title = SeqGSEA: a Bioconductor package for gene set enrichment analysis of RNA-Seq data integrating differential expression and splicing | journal = Bioinformatics | volume = 30 | issue = 12 | pages = 1777–9 | date = June 2014 | pmid = 24535097 | doi = 10.1093/bioinformatics/btu090 | doi-access = free }}</ref> | ||
* ''Isoform-based (also multi-read modules, differential isoform expression)'': estimate isoform abundance first, and then relative abundance between conditions. Examples are Cufflinks 2<ref>{{cite journal | vauthors = Trapnell C, Hendrickson DG, Sauvageau M, Goff L, Rinn JL, Pachter L | title = Differential analysis of gene regulation at transcript resolution with RNA-seq | journal = Nature Biotechnology | volume = 31 | issue = 1 | pages = 46–53 | date = January 2013 | pmid = 23222703 | pmc = 3869392 | doi = 10.1038/nbt.2450 }}</ref> and DiffSplice.<ref>{{cite journal | vauthors = Hu Y, Huang Y, Du Y, Orellana CF, Singh D, Johnson AR, Monroy A, Kuan PF, Hammond SM, Makowski L, Randell SH, Chiang DY, Hayes DN, Jones C, Liu Y, Prins JF, Liu J |
* ''Isoform-based (also multi-read modules, differential isoform expression)'': estimate isoform abundance first, and then relative abundance between conditions. Examples are Cufflinks 2<ref>{{cite journal | vauthors = Trapnell C, Hendrickson DG, Sauvageau M, Goff L, Rinn JL, Pachter L | title = Differential analysis of gene regulation at transcript resolution with RNA-seq | journal = Nature Biotechnology | volume = 31 | issue = 1 | pages = 46–53 | date = January 2013 | pmid = 23222703 | pmc = 3869392 | doi = 10.1038/nbt.2450 }}</ref> and DiffSplice.<ref>{{cite journal | vauthors = Hu Y, Huang Y, Du Y, Orellana CF, Singh D, Johnson AR, Monroy A, Kuan PF, Hammond SM, Makowski L, Randell SH, Chiang DY, Hayes DN, Jones C, Liu Y, Prins JF, Liu J | title = DiffSplice: the genome-wide detection of differential splicing events with RNA-seq | journal = Nucleic Acids Research | volume = 41 | issue = 2 | pages = e39 | date = January 2013 | pmid = 23155066 | pmc = 3553996 | doi = 10.1093/nar/gks1026 }}</ref> | ||
* ''Intron excision based:'' calculate alternative splicing using split reads. Examples are MAJIQ<ref>{{cite journal | vauthors = Vaquero-Garcia J, Barrera A, Gazzara MR, González-Vallinas J, Lahens NF, Hogenesch JB, Lynch KW, Barash Y |
* ''Intron excision based:'' calculate alternative splicing using split reads. Examples are MAJIQ<ref>{{cite journal | vauthors = Vaquero-Garcia J, Barrera A, Gazzara MR, González-Vallinas J, Lahens NF, Hogenesch JB, Lynch KW, Barash Y | title = A new view of transcriptome complexity and regulation through the lens of local splicing variations | journal = eLife | volume = 5 | pages = e11752 | date = February 2016 | pmid = 26829591 | pmc = 4801060 | doi = 10.7554/eLife.11752 | doi-access = free }}</ref> and Leafcutter.<ref name="Annotation-free quantification of R"/> | ||
Differential gene expression tools can also be used for differential isoform expression if isoforms are quantified ahead of time with other tools like RSEM.<ref>{{cite journal | vauthors = Merino GA, Conesa A, Fernández EA | title = A benchmarking of workflows for detecting differential splicing and differential expression at isoform level in human RNA-seq studies | journal = Briefings in Bioinformatics | volume = 20 | issue = 2 | pages = 471–481 | date = March 2019 | pmid = 29040385 | doi = 10.1093/bib/bbx122 | s2cid = 22706028 }}</ref> | Differential gene expression tools can also be used for differential isoform expression if isoforms are quantified ahead of time with other tools like RSEM.<ref>{{cite journal | vauthors = Merino GA, Conesa A, Fernández EA | title = A benchmarking of workflows for detecting differential splicing and differential expression at isoform level in human RNA-seq studies | journal = Briefings in Bioinformatics | volume = 20 | issue = 2 | pages = 471–481 | date = March 2019 | pmid = 29040385 | doi = 10.1093/bib/bbx122 | s2cid = 22706028 | hdl = 11336/41247 | hdl-access = free }}</ref> | ||
===Coexpression networks=== | ===Coexpression networks=== | ||
Coexpression networks are data-derived representations of genes behaving in a similar way across tissues and experimental conditions.<ref name=marcotte1999>{{cite journal | vauthors = Marcotte EM, Pellegrini M, Thompson MJ, Yeates TO, Eisenberg D | title = A combined algorithm for genome-wide prediction of protein function | journal = Nature | volume = 402 | issue = 6757 | pages = 83–6 | date = November 1999 | pmid = 10573421 | doi = 10.1038/47048 | bibcode = 1999Natur.402...83M | s2cid = 144447 }}</ref> Their main purpose lies in hypothesis generation and guilt-by-association approaches for inferring functions of previously unknown genes.<ref name="marcotte1999"/> RNA-Seq data has been used to infer genes involved in specific pathways based on ], both in plants<ref name=giorgi2013>{{cite journal | vauthors = Giorgi FM, Del Fabbro C, Licausi F | title = Comparative study of RNA-seq- and microarray-derived coexpression networks in Arabidopsis thaliana | journal = Bioinformatics | volume = 29 | issue = 6 | pages = 717–24 | date = March 2013 | pmid = 23376351 | doi = 10.1093/bioinformatics/btt053 | doi-access = free }}</ref> and mammals.<ref name=iancu2012>{{cite journal | vauthors = Iancu OD, Kawane S, Bottomly D, Searles R, Hitzemann R, McWeeney S | title = Utilizing RNA-Seq data for de novo coexpression network inference | journal = Bioinformatics | volume = 28 | issue = 12 | pages = 1592–7 | date = June 2012 | pmid = 22556371 | pmc = 3493127 | doi = 10.1093/bioinformatics/bts245 }}</ref> The main advantage of RNA-Seq data in this kind of analysis over the microarray platforms is the capability to cover the entire transcriptome, therefore allowing the possibility to unravel more complete representations of the gene regulatory networks. Differential regulation of the splice isoforms of the same gene can be detected and used to predict their biological functions.<ref>{{cite journal | vauthors = Eksi R, Li HD, Menon R, Wen Y, Omenn GS, Kretzler M, Guan Y | title = Systematically differentiating functions for alternatively spliced isoforms through integrating RNA-seq data | journal = PLOS Computational Biology | volume = 9 | issue = 11 | pages = e1003314 | date = |
Coexpression networks are data-derived representations of genes behaving in a similar way across tissues and experimental conditions.<ref name=marcotte1999>{{cite journal | vauthors = Marcotte EM, Pellegrini M, Thompson MJ, Yeates TO, Eisenberg D | title = A combined algorithm for genome-wide prediction of protein function | journal = Nature | volume = 402 | issue = 6757 | pages = 83–6 | date = November 1999 | pmid = 10573421 | doi = 10.1038/47048 | bibcode = 1999Natur.402...83M | s2cid = 144447 }}</ref> Their main purpose lies in hypothesis generation and guilt-by-association approaches for inferring functions of previously unknown genes.<ref name="marcotte1999"/> RNA-Seq data has been used to infer genes involved in specific pathways based on ], both in plants<ref name=giorgi2013>{{cite journal | vauthors = Giorgi FM, Del Fabbro C, Licausi F | title = Comparative study of RNA-seq- and microarray-derived coexpression networks in Arabidopsis thaliana | journal = Bioinformatics | volume = 29 | issue = 6 | pages = 717–24 | date = March 2013 | pmid = 23376351 | doi = 10.1093/bioinformatics/btt053 | doi-access = free | hdl = 11390/990155 | hdl-access = free }}</ref> and mammals.<ref name=iancu2012>{{cite journal | vauthors = Iancu OD, Kawane S, Bottomly D, Searles R, Hitzemann R, McWeeney S | title = Utilizing RNA-Seq data for de novo coexpression network inference | journal = Bioinformatics | volume = 28 | issue = 12 | pages = 1592–7 | date = June 2012 | pmid = 22556371 | pmc = 3493127 | doi = 10.1093/bioinformatics/bts245 }}</ref> The main advantage of RNA-Seq data in this kind of analysis over the microarray platforms is the capability to cover the entire transcriptome, therefore allowing the possibility to unravel more complete representations of the gene regulatory networks. Differential regulation of the splice isoforms of the same gene can be detected and used to predict their biological functions.<ref>{{cite journal | vauthors = Eksi R, Li HD, Menon R, Wen Y, Omenn GS, Kretzler M, Guan Y | title = Systematically differentiating functions for alternatively spliced isoforms through integrating RNA-seq data | journal = PLOS Computational Biology | volume = 9 | issue = 11 | pages = e1003314 | date = November 2013 | pmid = 24244129 | pmc = 3820534 | doi = 10.1371/journal.pcbi.1003314 | bibcode = 2013PLSCB...9E3314E | doi-access = free }}</ref><ref>{{cite journal | vauthors = Li HD, Menon R, Omenn GS, Guan Y | title = The emerging era of genomic data integration for analyzing splice isoform function | journal = Trends in Genetics | volume = 30 | issue = 8 | pages = 340–7 | date = August 2014 | pmid = 24951248 | pmc = 4112133 | doi = 10.1016/j.tig.2014.05.005 }}</ref> | ||
] has been successfully used to identify co-expression modules and intramodular hub genes based on RNA seq data. Co-expression modules may correspond to cell types or pathways. Highly connected intramodular hubs can be interpreted as representatives of their respective module. An eigengene is a weighted sum of expression of all genes in a module. Eigengenes are useful biomarkers (features) for diagnosis and prognosis.<ref name="Pigegene">{{cite journal | vauthors = Foroushani A, Agrahari R, Docking R, Chang L, Duns G, Hudoba M, Karsan A, Zare H |
] has been successfully used to identify co-expression modules and intramodular hub genes based on RNA seq data. Co-expression modules may correspond to cell types or pathways. Highly connected intramodular hubs can be interpreted as representatives of their respective module. An eigengene is a weighted sum of expression of all genes in a module. Eigengenes are useful biomarkers (features) for diagnosis and prognosis.<ref name="Pigegene">{{cite journal | vauthors = Foroushani A, Agrahari R, Docking R, Chang L, Duns G, Hudoba M, Karsan A, Zare H | title = Large-scale gene network analysis reveals the significance of extracellular matrix pathway and homeobox genes in acute myeloid leukemia: an introduction to the Pigengene package and its applications | journal = BMC Medical Genomics | volume = 10 | issue = 1 | pages = 16 | date = March 2017 | pmid = 28298217 | pmc = 5353782 | doi = 10.1186/s12920-017-0253-6 | doi-access = free }}</ref> Variance-Stabilizing Transformation approaches for estimating correlation coefficients based on RNA seq data have been proposed.<ref name="giorgi2013"/> | ||
===Variant discovery=== | ===Variant discovery=== | ||
RNA-Seq captures DNA variation, including ], ]. and ]. ] in RNA-Seq is similar to DNA variant calling and often employs the same tools (including SAMtools mpileup<ref>{{cite journal | vauthors = Li H, Handsaker B, Wysoker A, Fennell T, Ruan J, Homer N, Marth G, Abecasis G, Durbin R |
RNA-Seq captures DNA variation, including ], ]. and ]. ] in RNA-Seq is similar to DNA variant calling and often employs the same tools (including SAMtools mpileup<ref>{{cite journal | vauthors = Li H, Handsaker B, Wysoker A, Fennell T, Ruan J, Homer N, Marth G, Abecasis G, Durbin R | title = The Sequence Alignment/Map format and SAMtools | journal = Bioinformatics | volume = 25 | issue = 16 | pages = 2078–9 | date = August 2009 | pmid = 19505943 | doi = 10.1093/bioinformatics/btp352 | pmc = 2723002 }}</ref> and GATK HaplotypeCaller<ref>{{cite journal | vauthors = DePristo MA, Banks E, Poplin R, Garimella KV, Maguire JR, Hartl C, Philippakis AA, del Angel G, Rivas MA, Hanna M, McKenna A, Fennell TJ, Kernytsky AM, Sivachenko AY, Cibulskis K, Gabriel SB, Altshuler D, Daly MJ | title = A framework for variation discovery and genotyping using next-generation DNA sequencing data | journal = Nature Genetics | volume = 43 | issue = 5 | pages = 491–8 | date = May 2011 | pmid = 21478889 | doi = 10.1038/ng.806 | pmc = 3083463 }}</ref>) with adjustments to account for splicing. One unique dimension for RNA variants is ]: the variants from only one haplotype might be preferentially expressed due to regulatory effects including ] and ], and noncoding ].<ref>{{cite journal | vauthors = Battle A, Brown CD, Engelhardt BE, Montgomery SB | title = Genetic effects on gene expression across human tissues | journal = Nature | volume = 550 | issue = 7675 | pages = 204–213 | date = October 2017 | pmid = 29022597 | doi = 10.1038/nature24277 | pmc = 5776756 | bibcode = 2017Natur.550..204A | hdl = 10230/34202 | hdl-access = free }}</ref><ref>{{cite journal | vauthors = Richter F, Hoffman GE, Manheimer KB, Patel N, Sharp AJ, McKean D, Morton SU, DePalma S, Gorham J, Kitaygorodksy A, Porter GA, Giardini A, Shen Y, Chung WK, Seidman JG, Seidman CE, Schadt EE, Gelb BD | title = ORE identifies extreme expression effects enriched for rare variants | journal = Bioinformatics | volume = 35 | issue = 20 | pages = 3906–3912 | date = October 2019 | pmid = 30903145 | doi = 10.1093/bioinformatics/btz202 | pmc = 6792115 }}</ref> Limitations of RNA variant identification include that it only reflects expressed regions (in humans, <5% of the genome), could be subject to biases introduced by data processing (e.g., de novo transcriptome assemblies underestimate heterozygosity<ref>{{cite journal | vauthors = Freedman AH, Clamp M, Sackton TB | title = Error, noise and bias in de novo transcriptome assemblies | journal = Molecular Ecology Resources | volume = 21 | issue = 1 | pages = 18–29 | date = January 2021 | pmid = 32180366 | doi = 10.1111/1755-0998.13156 | s2cid = 212739959 }}</ref>), and has lower quality when compared to direct DNA sequencing. | ||
====RNA editing (post-transcriptional alterations)==== | ====RNA editing (post-transcriptional alterations)==== | ||
Line 164: | Line 168: | ||
{{See also|Fusion gene}} | {{See also|Fusion gene}} | ||
Caused by different structural modifications in the genome, fusion genes have gained attention because of their relationship with cancer.<ref name=teixeira2006>{{cite journal | vauthors = Teixeira MR | title = Recurrent fusion oncogenes in carcinomas | journal = Critical Reviews in Oncogenesis | volume = 12 | issue = 3–4 | pages = 257–71 | date = December 2006 | pmid = 17425505 | doi = 10.1615/critrevoncog.v12.i3-4.40 }}</ref> The ability of RNA-Seq to analyze a sample's whole transcriptome in an unbiased fashion makes it an attractive tool to find these kinds of common events in cancer.<ref name="maher2009">{{cite journal | vauthors = Maher CA, Kumar-Sinha C, Cao X, Kalyana-Sundaram S, Han B, Jing X, Sam L, Barrette T, Palanisamy N, Chinnaiyan AM |
Caused by different structural modifications in the genome, fusion genes have gained attention because of their relationship with cancer.<ref name=teixeira2006>{{cite journal | vauthors = Teixeira MR | title = Recurrent fusion oncogenes in carcinomas | journal = Critical Reviews in Oncogenesis | volume = 12 | issue = 3–4 | pages = 257–71 | date = December 2006 | pmid = 17425505 | doi = 10.1615/critrevoncog.v12.i3-4.40 | s2cid = 40770452 }}</ref> The ability of RNA-Seq to analyze a sample's whole transcriptome in an unbiased fashion makes it an attractive tool to find these kinds of common events in cancer.<ref name="maher2009">{{cite journal | vauthors = Maher CA, Kumar-Sinha C, Cao X, Kalyana-Sundaram S, Han B, Jing X, Sam L, Barrette T, Palanisamy N, Chinnaiyan AM | title = Transcriptome sequencing to detect gene fusions in cancer | journal = Nature | volume = 458 | issue = 7234 | pages = 97–101 | date = March 2009 | pmid = 19136943 | pmc = 2725402 | doi = 10.1038/nature07638 | bibcode = 2009Natur.458...97M }}</ref> | ||
The idea follows from the process of aligning the short transcriptomic reads to a reference genome. Most of the short reads will fall within one complete exon, and a smaller but still large set would be expected to map to known exon-exon junctions. The remaining unmapped short reads would then be further analyzed to determine whether they match an exon-exon junction where the exons come from different genes. This would be evidence of a possible fusion event, however, because of the length of the reads, this could prove to be very noisy. An alternative approach is to use paired-end reads, when a potentially large number of paired reads would map each end to a different exon, giving better coverage of these events (see figure). Nonetheless, the end result consists of multiple and potentially novel combinations of genes providing an ideal starting point for further validation. | The idea follows from the process of aligning the short transcriptomic reads to a reference genome. Most of the short reads will fall within one complete exon, and a smaller but still large set would be expected to map to known exon-exon junctions. The remaining unmapped short reads would then be further analyzed to determine whether they match an exon-exon junction where the exons come from different genes. This would be evidence of a possible fusion event, however, because of the length of the reads, this could prove to be very noisy. An alternative approach is to use paired-end reads, when a potentially large number of paired reads would map each end to a different exon, giving better coverage of these events (see figure). Nonetheless, the end result consists of multiple and potentially novel combinations of genes providing an ideal starting point for further validation. | ||
=== Copy number alteration === | |||
] (CNA) analyses are commonly used in cancer studies. Gain and loss of the genes have signalling pathway implications and are a key biomarker of molecular dysfunction in oncology. Calling the CNA information from RNA-Seq data is not straightforward because of the differences in gene expression, which lead to the read depth variance of different magnitudes across genes. Due to these difficulties, most of these analyses are usually done using whole-genome sequencing / whole-exome sequencing (WGS/WES). But advanced bioinformatics tools can call CNA from RNA-Seq.<ref>{{cite journal | vauthors = Thind AS, Monga I, Thakur PK, Kumari P, Dindhoria K, Krzak M, Ranson M, Ashford B | title = Demystifying emerging bulk RNA-Seq applications: the application and utility of bioinformatic methodology | journal = Briefings in Bioinformatics | volume = 22 | issue = 6 | date = November 2021 | pmid = 34329375 | doi = 10.1093/bib/bbab259 }}</ref> | |||
=== Other emerging analysis and applications === | |||
The applications of RNA-Seq are growing day by day. Other new application of RNA-Seq includes detection of microbial contaminants,<ref>{{cite journal | vauthors = Sangiovanni M, Granata I, Thind AS, Guarracino MR | title = From trash to treasure: detecting unexpected contamination in unmapped NGS data | journal = BMC Bioinformatics | volume = 20 | issue = Suppl 4 | pages = 168 | date = April 2019 | pmid = 30999839 | pmc = 6472186 | doi = 10.1186/s12859-019-2684-x | doi-access = free }}</ref> determining cell type abundance (cell type deconvolution),<ref name="Thind_2021" /> measuring the expression of TEs and Neoantigen prediction etc.<ref name="Thind_2021" /> | |||
==History== | ==History== | ||
] | ] | ||
RNA-Seq was first developed in mid |
RNA-Seq was first developed in mid 2000s with the advent of next-generation sequencing technology.<ref>{{cite journal | vauthors = Weber AP | title = Discovering New Biology through Sequencing of RNA | journal = Plant Physiology | volume = 169 | issue = 3 | pages = 1524–31 | date = November 2015 | pmid = 26353759 | pmc = 4634082 | doi = 10.1104/pp.15.01081 }}</ref> The first manuscripts that used RNA-Seq even without using the term includes those of ] ]<ref>{{cite journal | vauthors = Bainbridge MN, Warren RL, Hirst M, Romanuik T, Zeng T, Go A, Delaney A, Griffith M, Hickenbotham M, Magrini V, Mardis ER, Sadar MD, Siddiqui AS, Marra MA, Jones SJ | title = Analysis of the prostate cancer cell line LNCaP transcriptome using a sequencing-by-synthesis approach | journal = BMC Genomics | volume = 7 | pages = 246 | date = September 2006 | pmid = 17010196 | pmc = 1592491 | doi = 10.1186/1471-2164-7-246 | doi-access = free }}</ref> (dated 2006), '']''<ref>{{cite journal | vauthors = Cheung F, Haas BJ, Goldberg SM, May GD, Xiao Y, Town CD | title = Sequencing Medicago truncatula expressed sequenced tags using 454 Life Sciences technology | journal = BMC Genomics | volume = 7 | pages = 272 | date = October 2006 | pmid = 17062153 | pmc = 1635983 | doi = 10.1186/1471-2164-7-272 | doi-access = free }}</ref> (2006), maize<ref>{{cite journal | vauthors = Emrich SJ, Barbazuk WB, Li L, Schnable PS | title = Gene discovery and annotation using LCM-454 transcriptome sequencing | journal = Genome Research | volume = 17 | issue = 1 | pages = 69–73 | date = January 2007 | pmid = 17095711 | pmc = 1716268 | doi = 10.1101/gr.5145806 }}</ref> (2007), and '']''<ref>{{cite journal | vauthors = Weber AP, Weber KL, Carr K, Wilkerson C, Ohlrogge JB | title = Sampling the Arabidopsis transcriptome with massively parallel pyrosequencing | journal = Plant Physiology | volume = 144 | issue = 1 | pages = 32–42 | date = May 2007 | pmid = 17351049 | pmc = 1913805 | doi = 10.1104/pp.107.096677 }}</ref> (2007), while the term "RNA-Seq" itself was first mentioned in 2008.<ref name="mortazavi2008" /><ref>{{cite journal | vauthors = Nagalakshmi U, Wang Z, Waern K, Shou C, Raha D, Gerstein M, Snyder M | title = The transcriptional landscape of the yeast genome defined by RNA sequencing | journal = Science | volume = 320 | issue = 5881 | pages = 1344–9 | date = June 2008 | pmid = 18451266 | pmc = 2951732 | doi = 10.1126/science.1158441 | bibcode = 2008Sci...320.1344N }}</ref> The number of manuscripts referring to RNA-Seq in the title or abstract (Figure, blue line) is continuously increasing with 6754 manuscripts published in 2018. The intersection of RNA-Seq and medicine (Figure, gold line) has similar celerity.<ref>{{Cite journal| vauthors = Richter F |date=2021|title=A broad introduction to RNA-Seq|url=https://en.wikiversity.org/WikiJournal_of_Science/A_broad_introduction_to_RNA-Seq|journal=WikiJournal of Science|volume=4|issue=1|pages=4|doi=10.15347/WJS/2021.004|doi-access=free}}</ref> | ||
===Applications to medicine=== | ===Applications to medicine=== | ||
RNA-Seq has the potential to identify new disease biology, profile biomarkers for clinical indications, infer druggable pathways, and make genetic diagnoses. These results could be further personalized for subgroups or even individual patients, potentially highlighting more effective prevention, diagnostics, and therapy. The feasibility of this approach is in part dictated by costs in money and time; a related limitation is the required team of specialists (bioinformaticians, physicians/clinicians, basic researchers, technicians) to fully interpret the huge amount of data generated by this analysis.<ref>{{cite journal | vauthors = Sandberg R | title = Entering the era of single-cell transcriptomics in biology and medicine | journal = Nature Methods | volume = 11 | issue = 1 | pages = 22–4 | date = January 2014 | pmid = 24524133 | doi = 10.1038/nmeth.2764 | s2cid = 27632439 | url = https://zenodo.org/record/890299 }}</ref> | RNA-Seq has the potential to identify new disease biology, profile biomarkers for clinical indications, infer druggable pathways, and make genetic diagnoses.<ref>{{cite journal |last1=Cummings |first1=Beryl B. |last2=Marshall |first2=Jamie L. |last3=Tukiainen |first3=Taru |last4=Lek |first4=Monkol |last5=Donkervoort |first5=Sandra |last6=Foley |first6=A. Reghan |last7=Bolduc |first7=Veronique |last8=Waddell |first8=Leigh B. |last9=Sandaradura |first9=Sarah A. |last10=O’Grady |first10=Gina L. |last11=Estrella |first11=Elicia |last12=Reddy |first12=Hemakumar M. |last13=Zhao |first13=Fengmei |last14=Weisburd |first14=Ben |last15=Karczewski |first15=Konrad J. |last16=O’Donnell-Luria |first16=Anne H. |last17=Birnbaum |first17=Daniel |last18=Sarkozy |first18=Anna |last19=Hu |first19=Ying |last20=Gonorazky |first20=Hernan |last21=Claeys |first21=Kristl |last22=Joshi |first22=Himanshu |last23=Bournazos |first23=Adam |last24=Oates |first24=Emily C. |last25=Ghaoui |first25=Roula |last26=Davis |first26=Mark R. |last27=Laing |first27=Nigel G. |last28=Topf |first28=Ana |last29=Kang |first29=Peter B. |last30=Beggs |first30=Alan H. |last31=North |first31=Kathryn N. |last32=Straub |first32=Volker |last33=Dowling |first33=James J. |last34=Muntoni |first34=Francesco |last35=Clarke |first35=Nigel F. |last36=Cooper |first36=Sandra T. |last37=Bönnemann |first37=Carsten G. |last38=MacArthur |first38=Daniel G. |title=Improving genetic diagnosis in Mendelian disease with transcriptome sequencing |journal=Science Translational Medicine |date=19 April 2017 |volume=9 |issue=386 |doi=10.1126/scitranslmed.aal5209|pmid=28424332 |pmc=5548421 |hdl=10230/35912 |hdl-access=free }}</ref><ref>{{cite journal |last1=Kremer |first1=Laura S. |last2=Bader |first2=Daniel M. |last3=Mertes |first3=Christian |last4=Kopajtich |first4=Robert |last5=Pichler |first5=Garwin |last6=Iuso |first6=Arcangela |last7=Haack |first7=Tobias B. |last8=Graf |first8=Elisabeth |last9=Schwarzmayr |first9=Thomas |last10=Terrile |first10=Caterina |last11=Koňaříková |first11=Eliška |last12=Repp |first12=Birgit |last13=Kastenmüller |first13=Gabi |last14=Adamski |first14=Jerzy |last15=Lichtner |first15=Peter |last16=Leonhardt |first16=Christoph |last17=Funalot |first17=Benoit |last18=Donati |first18=Alice |last19=Tiranti |first19=Valeria |last20=Lombes |first20=Anne |last21=Jardel |first21=Claude |last22=Gläser |first22=Dieter |last23=Taylor |first23=Robert W. |last24=Ghezzi |first24=Daniele |last25=Mayr |first25=Johannes A. |last26=Rötig |first26=Agnes |last27=Freisinger |first27=Peter |last28=Distelmaier |first28=Felix |last29=Strom |first29=Tim M. |last30=Meitinger |first30=Thomas |last31=Gagneur |first31=Julien |last32=Prokisch |first32=Holger |title=Genetic diagnosis of Mendelian disorders via RNA sequencing |journal=Nature Communications |date=12 June 2017 |volume=8 |issue=1 |page=15824 |doi=10.1038/ncomms15824|pmid=28604674 |pmc=5499207 |bibcode=2017NatCo...815824K }}</ref> These results could be further personalized for subgroups or even individual patients, potentially highlighting more effective prevention, diagnostics, and therapy. The feasibility of this approach is in part dictated by costs in money and time; a related limitation is the required team of specialists (bioinformaticians, physicians/clinicians, basic researchers, technicians) to fully interpret the huge amount of data generated by this analysis.<ref>{{cite journal | vauthors = Sandberg R | title = Entering the era of single-cell transcriptomics in biology and medicine | journal = Nature Methods | volume = 11 | issue = 1 | pages = 22–4 | date = January 2014 | pmid = 24524133 | doi = 10.1038/nmeth.2764 | s2cid = 27632439 | url = https://zenodo.org/record/890299 }}</ref> | ||
===Large-scale sequencing efforts=== | ===Large-scale sequencing efforts=== | ||
A lot of emphasis has been given to RNA-Seq data after the ] and ] projects have used this approach to characterize dozens of cell lines<ref>{{cite web |url= http://genome.ucsc.edu/ENCODE/dataMatrix/encodeDataMatrixHuman.html |title=ENCODE Data Matrix |access-date= |
A lot of emphasis has been given to RNA-Seq data after the ] and ] (TCGA) projects have used this approach to characterize dozens of cell lines<ref>{{cite web |url= http://genome.ucsc.edu/ENCODE/dataMatrix/encodeDataMatrixHuman.html |title=ENCODE Data Matrix |access-date=28 July 2013}}</ref> and thousands of primary tumor samples,<ref>{{cite web |url=https://tcga-data.nci.nih.gov/tcga/tcgaHome2.jsp |title=The Cancer Genome Atlas – Data Portal |access-date=28 July 2013}}</ref> respectively. ENCODE aimed to identify genome-wide regulatory regions in different cohort of cell lines and transcriptomic data are paramount to understand the downstream effect of those epigenetic and genetic regulatory layers. TCGA, instead, aimed to collect and analyze thousands of patient's samples from 30 different tumor types to understand the underlying mechanisms of malignant transformation and progression. In this context RNA-Seq data provide a unique snapshot of the transcriptomic status of the disease and look at an unbiased population of transcripts that allows the identification of novel transcripts, fusion transcripts and non-coding RNAs that could be undetected with different technologies. | ||
== See also == | == See also == | ||
* ] | * ] | ||
* ] | * ] | ||
* ] | * ] | ||
== References == | == References == | ||
{{Academic peer reviewed|Q=Q100146647}}{{Reflist|2}} | {{Academic peer reviewed|Q=Q100146647|doi-access=free}} | ||
{{Reflist|2}} | |||
== Further reading == | == Further reading == | ||
{{refbegin}} | {{refbegin}} | ||
* {{cite book |doi=10.1016/B978-0-12-809633-8.20163-5 |chapter=Comparative Transcriptomics Analysis |title=Encyclopedia of Bioinformatics and Computational Biology |pages=814–818 |year=2019 | vauthors = Taguchi Y |isbn= |
* {{cite book |doi=10.1016/B978-0-12-809633-8.20163-5 |chapter=Comparative Transcriptomics Analysis |title=Encyclopedia of Bioinformatics and Computational Biology |pages=814–818 |year=2019 | vauthors = Taguchi Y |isbn=978-0-12-811432-2 |s2cid=65302519 }} | ||
{{refend}} | {{refend}} | ||
Latest revision as of 05:04, 28 October 2024
Lab technique in cellular biology

RNA-Seq (named as an abbreviation of RNA sequencing) is a technique that uses next-generation sequencing to reveal the presence and quantity of RNA molecules in a biological sample, providing a snapshot of gene expression in the sample, also known as transcriptome.
Specifically, RNA-Seq facilitates the ability to look at alternative gene spliced transcripts, post-transcriptional modifications, gene fusion, mutations/SNPs and changes in gene expression over time, or differences in gene expression in different groups or treatments. In addition to mRNA transcripts, RNA-Seq can look at different populations of RNA to include total RNA, small RNA, such as miRNA, tRNA, and ribosomal profiling. RNA-Seq can also be used to determine exon/intron boundaries and verify or amend previously annotated 5' and 3' gene boundaries. Recent advances in RNA-Seq include single cell sequencing, bulk RNA sequencing, 3' mRNA-sequencing, in situ sequencing of fixed tissue, and native RNA molecule sequencing with single-molecule real-time sequencing. Other examples of emerging RNA-Seq applications due to the advancement of bioinformatics algorithms are copy number alteration, microbial contamination, transposable elements, cell type (deconvolution) and the presence of neoantigens.
Prior to RNA-Seq, gene expression studies were done with hybridization-based microarrays. Issues with microarrays include cross-hybridization artifacts, poor quantification of lowly and highly expressed genes, and needing to know the sequence a priori. Because of these technical issues, transcriptomics transitioned to sequencing-based methods. These progressed from Sanger sequencing of Expressed sequence tag libraries, to chemical tag-based methods (e.g., serial analysis of gene expression), and finally to the current technology, next-gen sequencing of complementary DNA (cDNA), notably RNA-Seq.

Methods
Library preparation
See also: Library (biology)
The general steps to prepare a complementary DNA (cDNA) library for sequencing are described below, but often vary between platforms.
- RNA Isolation: RNA is isolated from tissue and mixed with Deoxyribonuclease (DNase). DNase reduces the amount of genomic DNA. The amount of RNA degradation is checked with gel and capillary electrophoresis and is used to assign an RNA integrity number to the sample. This RNA quality and the total amount of starting RNA are taken into consideration during the subsequent library preparation, sequencing, and analysis steps.
- RNA selection/depletion: To analyze signals of interest, the isolated RNA can either be kept as is, enriched for RNA with 3' polyadenylated (poly(A)) tails to include only eukaryotic mRNA, depleted of ribosomal RNA (rRNA), and/or filtered for RNA that binds specific sequences (RNA selection and depletion methods table, below). RNA molecules having 3' poly(A) tails in eukaryotes are mainly composed of mature, processed, coding sequences. Poly(A) selection is performed by mixing RNA with poly(T) oligomers covalently attached to a substrate, typically magnetic beads. Poly(A) selection has important limitations in RNA biotype detection. Many RNA biotypes are not polyadenylated, including many noncoding RNA and histone-core protein transcripts, or are regulated via their poly(A) tail length (e.g., cytokines) and thus might not be detected after poly(A) selection. Furthermore, poly(A) selection may display increased 3' bias, especially with lower quality RNA. These limitations can be avoided with ribosomal depletion, removing rRNA that typically represents over 90% of the RNA in a cell. Both poly(A) enrichment and ribosomal depletion steps are labor intensive and could introduce biases, so more simple approaches have been developed to omit these steps. Small RNA targets, such as miRNA, can be further isolated through size selection with exclusion gels, magnetic beads, or commercial kits.
- cDNA synthesis: RNA is reverse transcribed to cDNA because DNA is more stable and to allow for amplification (which uses DNA polymerases) and leverage more mature DNA sequencing technology. Amplification subsequent to reverse transcription results in loss of strandedness, which can be avoided with chemical labeling or single molecule sequencing. Fragmentation and size selection are performed to purify sequences that are the appropriate length for the sequencing machine. The RNA, cDNA, or both are fragmented with enzymes, sonication, divalent ions, or nebulizers. Fragmentation of the RNA reduces 5' bias of randomly primed-reverse transcription and the influence of primer binding sites, with the downside that the 5' and 3' ends are converted to DNA less efficiently. Fragmentation is followed by size selection, where either small sequences are removed or a tight range of sequence lengths are selected. Because small RNAs like miRNAs are lost, these are analyzed independently. The cDNA for each experiment can be indexed with a hexamer or octamer barcode, so that these experiments can be pooled into a single lane for multiplexed sequencing.
Strategy | Predominant type of RNA | Ribosomal RNA content | Unprocessed RNA content | Isolation method |
---|---|---|---|---|
Total RNA | All | High | High | None |
PolyA selection | Coding | Low | Low | Hybridization with poly(dT) oligomers |
rRNA depletion | Coding, noncoding | Low | High | Removal of oligomers complementary to rRNA |
RNA capture | Targeted | Low | Moderate | Hybridization with probes complementary to desired transcripts |
Complementary DNA sequencing (cDNA-Seq)
See also: w:DNA sequencingThe cDNA library derived from RNA biotypes is then sequenced into a computer-readable format. There are many high-throughput sequencing technologies for cDNA sequencing including platforms developed by Illumina, Thermo Fisher, BGI/MGI, PacBio, and Oxford Nanopore Technologies. For Illumina short-read sequencing, a common technology for cDNA sequencing, adapters are ligated to the cDNA, DNA is attached to a flow cell, clusters are generated through cycles of bridge amplification and denaturing, and sequence-by-synthesis is performed in cycles of complementary strand synthesis and laser excitation of bases with reversible terminators. Sequencing platform choice and parameters are guided by experimental design and cost. Common experimental design considerations include deciding on the sequencing length, sequencing depth, use of single versus paired-end sequencing, number of replicates, multiplexing, randomization, and spike-ins.
Small RNA/non-coding RNA sequencing
When sequencing RNA other than mRNA, the library preparation is modified. The cellular RNA is selected based on the desired size range. For small RNA targets, such as miRNA, the RNA is isolated through size selection. This can be performed with a size exclusion gel, through size selection magnetic beads, or with a commercially developed kit. Once isolated, linkers are added to the 3' and 5' end then purified. The final step is cDNA generation through reverse transcription.
Direct RNA sequencing

Because converting RNA into cDNA, ligation, amplification, and other sample manipulations have been shown to introduce biases and artifacts that may interfere with both the proper characterization and quantification of transcripts, single molecule direct RNA sequencing has been explored by companies including Helicos (bankrupt), Oxford Nanopore Technologies, and others. This technology sequences RNA molecules directly in a massively-parallel manner.
Single-molecule real-time RNA sequencing
See also: Single-molecule real-time sequencingMassively parallel single molecule direct RNA-Seq has been explored as an alternative to traditional RNA-Seq, in which RNA-to-cDNA conversion, ligation, amplification, and other sample manipulation steps may introduce biases and artifacts. Technology platforms that perform single-molecule real-time RNA-Seq include Oxford Nanopore Technologies (ONT) Nanopore sequencing, PacBio IsoSeq, and Helicos (bankrupt). Sequencing RNA in its native form preserves modifications like methylation, allowing them to be investigated directly and simultaneously. Another benefit of single-molecule RNA-Seq is that transcripts can be covered in full length, allowing for higher confidence isoform detection and quantification compared to short-read sequencing. Traditionally, single-molecule RNA-Seq methods have higher error rates compared to short-read sequencing, but newer methods like ONT direct RNA-Seq limit errors by avoiding fragmentation and cDNA conversion. Recent uses of ONT direct RNA-Seq for differential expression in human cell populations have demonstrated that this technology can overcome many limitations of short and long cDNA sequencing.
Single-cell RNA sequencing (scRNA-Seq)
Main article: Single-cell transcriptomics See also: Single cell sequencingStandard methods such as microarrays and standard bulk RNA-Seq analysis analyze the expression of RNAs from large populations of cells. In mixed cell populations, these measurements may obscure critical differences between individual cells within these populations.
Single-cell RNA sequencing (scRNA-Seq) provides the expression profiles of individual cells. Although it is not possible to obtain complete information on every RNA expressed by each cell, due to the small amount of material available, patterns of gene expression can be identified through gene clustering analyses. This can uncover the existence of rare cell types within a cell population that may never have been seen before. For example, rare specialized cells in the lung called pulmonary ionocytes that express the Cystic fibrosis transmembrane conductance regulator were identified in 2018 by two groups performing scRNA-Seq on lung airway epithelia.
Experimental procedures

Current scRNA-Seq protocols involve the following steps: isolation of single cell and RNA, reverse transcription (RT), amplification, library generation and sequencing. Single cells are either mechanically separated into microwells (e.g., BD Rhapsody, Takara ICELL8, Vycap Puncher Platform, or CellMicrosystems CellRaft) or encapsulated in droplets (e.g., 10x Genomics Chromium, Illumina Bio-Rad ddSEQ, 1CellBio InDrop, Dolomite Bio Nadia). Single cells are labeled by adding beads with barcoded oligonucleotides; both cells and beads are supplied in limited amounts such that co-occupancy with multiple cells and beads is a very rare event. Once reverse transcription is complete, the cDNAs from many cells can be mixed together for sequencing; transcripts from a particular cell are identified by each cell's unique barcode. Unique molecular identifier (UMIs) can be attached to mRNA/cDNA target sequences to help identify artifacts during library preparation.
Challenges for scRNA-Seq include preserving the initial relative abundance of mRNA in a cell and identifying rare transcripts. The reverse transcription step is critical as the efficiency of the RT reaction determines how much of the cell's RNA population will be eventually analyzed by the sequencer. The processivity of reverse transcriptases and the priming strategies used may affect full-length cDNA production and the generation of libraries biased toward the 3’ or 5' end of genes.
In the amplification step, either PCR or in vitro transcription (IVT) is currently used to amplify cDNA. One of the advantages of PCR-based methods is the ability to generate full-length cDNA. However, different PCR efficiency on particular sequences (for instance, GC content and snapback structure) may also be exponentially amplified, producing libraries with uneven coverage. On the other hand, while libraries generated by IVT can avoid PCR-induced sequence bias, specific sequences may be transcribed inefficiently, thus causing sequence drop-out or generating incomplete sequences. Several scRNA-Seq protocols have been published: Tang et al., STRT, SMART-seq, CEL-seq, RAGE-seq, Quartz-seq and C1-CAGE. These protocols differ in terms of strategies for reverse transcription, cDNA synthesis and amplification, and the possibility to accommodate sequence-specific barcodes (i.e. UMIs) or the ability to process pooled samples.
In 2017, two approaches were introduced to simultaneously measure single-cell mRNA and protein expression through oligonucleotide-labeled antibodies known as REAP-seq, and CITE-seq.
Applications
scRNA-Seq is becoming widely used across biological disciplines including Development, Neurology, Oncology, Autoimmune disease, and Infectious disease.
scRNA-Seq has provided considerable insight into the development of embryos and organisms, including the worm Caenorhabditis elegans, and the regenerative planarian Schmidtea mediterranea. The first vertebrate animals to be mapped in this way were Zebrafish and Xenopus laevis. In each case multiple stages of the embryo were studied, allowing the entire process of development to be mapped on a cell-by-cell basis. Science recognized these advances as the 2018 Breakthrough of the Year.
Experimental considerations
A variety of parameters are considered when designing and conducting RNA-Seq experiments:
- Tissue specificity: Gene expression varies within and between tissues, and RNA-Seq measures this mix of cell types. This may make it difficult to isolate the biological mechanism of interest. Single cell sequencing can be used to study each cell individually, mitigating this issue.
- Time dependence: Gene expression changes over time, and RNA-Seq only takes a snapshot. Time course experiments can be performed to observe changes in the transcriptome.
- Coverage (also known as depth): RNA harbors the same mutations observed in DNA, and detection requires deeper coverage. With high enough coverage, RNA-Seq can be used to estimate the expression of each allele. This may provide insight into phenomena such as imprinting or cis-regulatory effects. The depth of sequencing required for specific applications can be extrapolated from a pilot experiment.
- Data generation artifacts (also known as technical variance): The reagents (e.g., library preparation kit), personnel involved, and type of sequencer (e.g., Illumina, Pacific Biosciences) can result in technical artifacts that might be mis-interpreted as meaningful results. As with any scientific experiment, it is prudent to conduct RNA-Seq in a well controlled setting. If this is not possible or the study is a meta-analysis, another solution is to detect technical artifacts by inferring latent variables (typically principal component analysis or factor analysis) and subsequently correcting for these variables.
- Data management: A single RNA-Seq experiment in humans is usually 1-5 Gb (compressed), or more when including intermediate files. This large volume of data can pose storage issues. One solution is compressing the data using multi-purpose computational schemas (e.g., gzip) or genomics-specific schemas. The latter can be based on reference sequences or de novo. Another solution is to perform microarray experiments, which may be sufficient for hypothesis-driven work or replication studies (as opposed to exploratory research).
Analysis
See also: List of RNA-Seq bioinformatics tools
Transcriptome assembly
See also: Sequence alignment software § Short-Read Sequence AlignmentTwo methods are used to assign raw sequence reads to genomic features (i.e., assemble the transcriptome):
- De novo: This approach does not require a reference genome to reconstruct the transcriptome, and is typically used if the genome is unknown, incomplete, or substantially altered compared to the reference. Challenges when using short reads for de novo assembly include 1) determining which reads should be joined together into contiguous sequences (contigs), 2) robustness to sequencing errors and other artifacts, and 3) computational efficiency. The primary algorithm used for de novo assembly transitioned from overlap graphs, which identify all pair-wise overlaps between reads, to de Bruijn graphs, which break reads into sequences of length k and collapse all k-mers into a hash table. Overlap graphs were used with Sanger sequencing, but do not scale well to the millions of reads generated with RNA-Seq. Examples of assemblers that use de Bruijn graphs are Trinity, Oases (derived from the genome assembler Velvet), Bridger, and rnaSPAdes. Paired-end and long-read sequencing of the same sample can mitigate the deficits in short read sequencing by serving as a template or skeleton. Metrics to assess the quality of a de novo assembly include median contig length, number of contigs and N50.
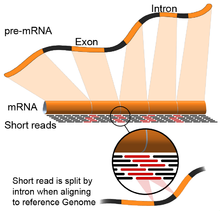
- Genome guided: This approach relies on the same methods used for DNA alignment, with the additional complexity of aligning reads that cover non-continuous portions of the reference genome. These non-continuous reads are the result of sequencing spliced transcripts (see figure). Typically, alignment algorithms have two steps: 1) align short portions of the read (i.e., seed the genome), and 2) use dynamic programming to find an optimal alignment, sometimes in combination with known annotations. Software tools that use genome-guided alignment include Bowtie, TopHat (which builds on BowTie results to align splice junctions), Subread, STAR, HISAT2, and GMAP. The output of genome guided alignment (mapping) tools can be further used by tools such as Cufflinks or StringTie to reconstruct contiguous transcript sequences (i.e., a FASTA file). The quality of a genome guided assembly can be measured with both 1) de novo assembly metrics (e.g., N50) and 2) comparisons to known transcript, splice junction, genome, and protein sequences using precision, recall, or their combination (e.g., F1 score). In addition, in silico assessment could be performed using simulated reads.
A note on assembly quality: The current consensus is that 1) assembly quality can vary depending on which metric is used, 2) assembly tools that scored well in one species do not necessarily perform well in the other species, and 3) combining different approaches might be the most reliable.
Gene expression quantification
Expression is quantified to study cellular changes in response to external stimuli, differences between healthy and diseased states, and other research questions. Transcript levels are often used as a proxy for protein abundance, but these are often not equivalent due to post transcriptional events such as RNA interference and nonsense-mediated decay.
Expression is quantified by counting the number of reads that mapped to each locus in the transcriptome assembly step. Expression can be quantified for exons or genes using contigs or reference transcript annotations. These observed RNA-Seq read counts have been robustly validated against older technologies, including expression microarrays and qPCR. Tools that quantify counts are HTSeq, FeatureCounts, Rcount, maxcounts, FIXSEQ, and Cuffquant. These tools determine read counts from aligned RNA-Seq data, but alignment-free counts can also be obtained with Sailfish and Kallisto. The read counts are then converted into appropriate metrics for hypothesis testing, regressions, and other analyses. Parameters for this conversion are:
- Sequencing depth/coverage: Although depth is pre-specified when conducting multiple RNA-Seq experiments, it will still vary widely between experiments. Therefore, the total number of reads generated in a single experiment is typically normalized by converting counts to fragments, reads, or counts per million mapped reads (FPM, RPM, or CPM). The difference between RPM and FPM was historically derived during the evolution from single-end sequencing of fragments to paired-end sequencing. In single-end sequencing, there is only one read per fragment (i.e., RPM = FPM). In paired-end sequencing, there are two reads per fragment (i.e., RPM = 2 x FPM). Sequencing depth is sometimes referred to as library size, the number of intermediary cDNA molecules in the experiment.
- Gene length: Longer genes will have more fragments/reads/counts than shorter genes if transcript expression is the same. This is adjusted by dividing the FPM by the length of a feature (which can be a gene, transcript, or exon), resulting in the metric fragments per kilobase of feature per million mapped reads (FPKM). When looking at groups of features across samples, FPKM is converted to transcripts per million (TPM) by dividing each FPKM by the sum of FPKMs within a sample.
- Total sample RNA output: Because the same amount of RNA is extracted from each sample, samples with more total RNA will have less RNA per gene. These genes appear to have decreased expression, resulting in false positives in downstream analyses. Normalization strategies including quantile, DESeq2, TMM and Median Ratio attempt to account for this difference by comparing a set of non-differentially expressed genes between samples and scaling accordingly.
- Variance for each gene's expression: is modeled to account for sampling error (important for genes with low read counts), increase power, and decrease false positives. Variance can be estimated as a normal, Poisson, or negative binomial distribution and is frequently decomposed into technical and biological variance.
Spike-ins for absolute quantification and detection of genome-wide effects
RNA spike-ins are samples of RNA at known concentrations that can be used as gold standards in experimental design and during downstream analyses for absolute quantification and detection of genome-wide effects.
- Absolute quantification: Absolute quantification of gene expression is not possible with most RNA-Seq experiments, which quantify expression relative to all transcripts. It is possible by performing RNA-Seq with spike-ins, samples of RNA at known concentrations. After sequencing, read counts of spike-in sequences are used to determine the relationship between each gene's read counts and absolute quantities of biological fragments. In one example, this technique was used in Xenopus tropicalis embryos to determine transcription kinetics.
- Detection of genome-wide effects: Changes in global regulators including chromatin remodelers, transcription factors (e.g., MYC), acetyltransferase complexes, and nucleosome positioning are not congruent with normalization assumptions and spike-in controls can offer precise interpretation.
Differential expression
The simplest but often most powerful use of RNA-Seq is finding differences in gene expression between two or more conditions (e.g., treated vs not treated); this process is called differential expression. The outputs are frequently referred to as differentially expressed genes (DEGs) and these genes can either be up- or down-regulated (i.e., higher or lower in the condition of interest). There are many tools that perform differential expression. Most are run in R, Python, or the Unix command line. Commonly used tools include DESeq, edgeR, and voom+limma, all of which are available through R/Bioconductor. These are the common considerations when performing differential expression:
- Inputs: Differential expression inputs include (1) an RNA-Seq expression matrix (M genes x N samples) and (2) a design matrix containing experimental conditions for N samples. The simplest design matrix contains one column, corresponding to labels for the condition being tested. Other covariates (also referred to as factors, features, labels, or parameters) can include batch effects, known artifacts, and any metadata that might confound or mediate gene expression. In addition to known covariates, unknown covariates can also be estimated through unsupervised machine learning approaches including principal component, surrogate variable, and PEER analyses. Hidden variable analyses are often employed for human tissue RNA-Seq data, which typically have additional artifacts not captured in the metadata (e.g., ischemic time, sourcing from multiple institutions, underlying clinical traits, collecting data across many years with many personnel).
- Methods: Most tools use regression or non-parametric statistics to identify differentially expressed genes, and are either based on read counts mapped to a reference genome (DESeq2, limma, edgeR) or based on read counts derived from alignment-free quantification (sleuth, Cuffdiff, Ballgown). Following regression, most tools employ either familywise error rate (FWER) or false discovery rate (FDR) p-value adjustments to account for multiple hypotheses (in human studies, ~20,000 protein-coding genes or ~50,000 biotypes).
- Outputs: A typical output consists of rows corresponding to the number of genes and at least three columns, each gene's log fold change (log-transform of the ratio in expression between conditions, a measure of effect size), p-value, and p-value adjusted for multiple comparisons. Genes are defined as biologically meaningful if they pass cut-offs for effect size (log fold change) and statistical significance. These cut-offs should ideally be specified a priori, but the nature of RNA-Seq experiments is often exploratory so it is difficult to predict effect sizes and pertinent cut-offs ahead of time.
- Pitfalls: The raison d'etre for these complex methods is to avoid the myriad of pitfalls that can lead to statistical errors and misleading interpretations. Pitfalls include increased false positive rates (due to multiple comparisons), sample preparation artifacts, sample heterogeneity (like mixed genetic backgrounds), highly correlated samples, unaccounted for multi-level experimental designs, and poor experimental design. One notable pitfall is viewing results in Microsoft Excel without using the import feature to ensure that the gene names remain text. Although convenient, Excel automatically converts some gene names (SEPT1, DEC1, MARCH2) into dates or floating point numbers.
- Choice of tools and benchmarking: There are numerous efforts that compare the results of these tools, with DESeq2 tending to moderately outperform other methods. As with other methods, benchmarking consists of comparing tool outputs to each other and known gold standards.
Downstream analyses for a list of differentially expressed genes come in two flavors, validating observations and making biological inferences. Owing to the pitfalls of differential expression and RNA-Seq, important observations are replicated with (1) an orthogonal method in the same samples (like real-time PCR) or (2) another, sometimes pre-registered, experiment in a new cohort. The latter helps ensure generalizability and can typically be followed up with a meta-analysis of all the pooled cohorts. The most common method for obtaining higher-level biological understanding of the results is gene set enrichment analysis, although sometimes candidate gene approaches are employed. Gene set enrichment determines if the overlap between two gene sets is statistically significant, in this case the overlap between differentially expressed genes and gene sets from known pathways/databases (e.g., Gene Ontology, KEGG, Human Phenotype Ontology) or from complementary analyses in the same data (like co-expression networks). Common tools for gene set enrichment include web interfaces (e.g., ENRICHR, g:profiler, WEBGESTALT) and software packages. When evaluating enrichment results, one heuristic is to first look for enrichment of known biology as a sanity check and then expand the scope to look for novel biology.

Alternative splicing
RNA splicing is integral to eukaryotes and contributes significantly to protein regulation and diversity, occurring in >90% of human genes. There are multiple alternative splicing modes: exon skipping (most common splicing mode in humans and higher eukaryotes), mutually exclusive exons, alternative donor or acceptor sites, intron retention (most common splicing mode in plants, fungi, and protozoa), alternative transcription start site (promoter), and alternative polyadenylation. One goal of RNA-Seq is to identify alternative splicing events and test if they differ between conditions. Long-read sequencing captures the full transcript and thus minimizes many of issues in estimating isoform abundance, like ambiguous read mapping. For short-read RNA-Seq, there are multiple methods to detect alternative splicing that can be classified into three main groups:
- Count-based (also event-based, differential splicing): estimate exon retention. Examples are DEXSeq, MATS, and SeqGSEA.
- Isoform-based (also multi-read modules, differential isoform expression): estimate isoform abundance first, and then relative abundance between conditions. Examples are Cufflinks 2 and DiffSplice.
- Intron excision based: calculate alternative splicing using split reads. Examples are MAJIQ and Leafcutter.
Differential gene expression tools can also be used for differential isoform expression if isoforms are quantified ahead of time with other tools like RSEM.
Coexpression networks
Coexpression networks are data-derived representations of genes behaving in a similar way across tissues and experimental conditions. Their main purpose lies in hypothesis generation and guilt-by-association approaches for inferring functions of previously unknown genes. RNA-Seq data has been used to infer genes involved in specific pathways based on Pearson correlation, both in plants and mammals. The main advantage of RNA-Seq data in this kind of analysis over the microarray platforms is the capability to cover the entire transcriptome, therefore allowing the possibility to unravel more complete representations of the gene regulatory networks. Differential regulation of the splice isoforms of the same gene can be detected and used to predict their biological functions. Weighted gene co-expression network analysis has been successfully used to identify co-expression modules and intramodular hub genes based on RNA seq data. Co-expression modules may correspond to cell types or pathways. Highly connected intramodular hubs can be interpreted as representatives of their respective module. An eigengene is a weighted sum of expression of all genes in a module. Eigengenes are useful biomarkers (features) for diagnosis and prognosis. Variance-Stabilizing Transformation approaches for estimating correlation coefficients based on RNA seq data have been proposed.
Variant discovery
RNA-Seq captures DNA variation, including single nucleotide variants, small insertions/deletions. and structural variation. Variant calling in RNA-Seq is similar to DNA variant calling and often employs the same tools (including SAMtools mpileup and GATK HaplotypeCaller) with adjustments to account for splicing. One unique dimension for RNA variants is allele-specific expression (ASE): the variants from only one haplotype might be preferentially expressed due to regulatory effects including imprinting and expression quantitative trait loci, and noncoding rare variants. Limitations of RNA variant identification include that it only reflects expressed regions (in humans, <5% of the genome), could be subject to biases introduced by data processing (e.g., de novo transcriptome assemblies underestimate heterozygosity), and has lower quality when compared to direct DNA sequencing.
RNA editing (post-transcriptional alterations)
See also: RNA editingHaving the matching genomic and transcriptomic sequences of an individual can help detect post-transcriptional edits (RNA editing). A post-transcriptional modification event is identified if the gene's transcript has an allele/variant not observed in the genomic data.
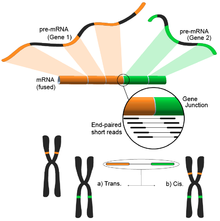
Fusion gene detection
See also: Fusion geneCaused by different structural modifications in the genome, fusion genes have gained attention because of their relationship with cancer. The ability of RNA-Seq to analyze a sample's whole transcriptome in an unbiased fashion makes it an attractive tool to find these kinds of common events in cancer.
The idea follows from the process of aligning the short transcriptomic reads to a reference genome. Most of the short reads will fall within one complete exon, and a smaller but still large set would be expected to map to known exon-exon junctions. The remaining unmapped short reads would then be further analyzed to determine whether they match an exon-exon junction where the exons come from different genes. This would be evidence of a possible fusion event, however, because of the length of the reads, this could prove to be very noisy. An alternative approach is to use paired-end reads, when a potentially large number of paired reads would map each end to a different exon, giving better coverage of these events (see figure). Nonetheless, the end result consists of multiple and potentially novel combinations of genes providing an ideal starting point for further validation.
Copy number alteration
Copy number alteration (CNA) analyses are commonly used in cancer studies. Gain and loss of the genes have signalling pathway implications and are a key biomarker of molecular dysfunction in oncology. Calling the CNA information from RNA-Seq data is not straightforward because of the differences in gene expression, which lead to the read depth variance of different magnitudes across genes. Due to these difficulties, most of these analyses are usually done using whole-genome sequencing / whole-exome sequencing (WGS/WES). But advanced bioinformatics tools can call CNA from RNA-Seq.
Other emerging analysis and applications
The applications of RNA-Seq are growing day by day. Other new application of RNA-Seq includes detection of microbial contaminants, determining cell type abundance (cell type deconvolution), measuring the expression of TEs and Neoantigen prediction etc.
History

RNA-Seq was first developed in mid 2000s with the advent of next-generation sequencing technology. The first manuscripts that used RNA-Seq even without using the term includes those of prostate cancer cell lines (dated 2006), Medicago truncatula (2006), maize (2007), and Arabidopsis thaliana (2007), while the term "RNA-Seq" itself was first mentioned in 2008. The number of manuscripts referring to RNA-Seq in the title or abstract (Figure, blue line) is continuously increasing with 6754 manuscripts published in 2018. The intersection of RNA-Seq and medicine (Figure, gold line) has similar celerity.
Applications to medicine
RNA-Seq has the potential to identify new disease biology, profile biomarkers for clinical indications, infer druggable pathways, and make genetic diagnoses. These results could be further personalized for subgroups or even individual patients, potentially highlighting more effective prevention, diagnostics, and therapy. The feasibility of this approach is in part dictated by costs in money and time; a related limitation is the required team of specialists (bioinformaticians, physicians/clinicians, basic researchers, technicians) to fully interpret the huge amount of data generated by this analysis.
Large-scale sequencing efforts
A lot of emphasis has been given to RNA-Seq data after the Encyclopedia of DNA Elements (ENCODE) and The Cancer Genome Atlas (TCGA) projects have used this approach to characterize dozens of cell lines and thousands of primary tumor samples, respectively. ENCODE aimed to identify genome-wide regulatory regions in different cohort of cell lines and transcriptomic data are paramount to understand the downstream effect of those epigenetic and genetic regulatory layers. TCGA, instead, aimed to collect and analyze thousands of patient's samples from 30 different tumor types to understand the underlying mechanisms of malignant transformation and progression. In this context RNA-Seq data provide a unique snapshot of the transcriptomic status of the disease and look at an unbiased population of transcripts that allows the identification of novel transcripts, fusion transcripts and non-coding RNAs that could be undetected with different technologies.
See also
References
This article was submitted to WikiJournal of Science for external academic peer review in 2019 (reviewer reports). The updated content was reintegrated into the Misplaced Pages page under a CC-BY-SA-3.0 license (2021). The version of record as reviewed is:
Felix Richter, et al. (17 May 2021). "A broad introduction to RNA-Seq" (PDF). WikiJournal of Science. 4 (2): 4. doi:10.15347/WJS/2021.004. ISSN 2470-6345. Wikidata Q100146647.
- Lowe R, Shirley N, Bleackley M, Dolan S, Shafee T (May 2017). "Transcriptomics technologies". PLOS Computational Biology. 13 (5): e1005457. Bibcode:2017PLSCB..13E5457L. doi:10.1371/journal.pcbi.1005457. PMC 5436640. PMID 28545146.
- Chu Y, Corey DR (August 2012). "RNA sequencing: platform selection, experimental design, and data interpretation". Nucleic Acid Therapeutics. 22 (4): 271–4. doi:10.1089/nat.2012.0367. PMC 3426205. PMID 22830413.
- ^ Wang Z, Gerstein M, Snyder M (January 2009). "RNA-Seq: a revolutionary tool for transcriptomics". Nature Reviews. Genetics. 10 (1): 57–63. doi:10.1038/nrg2484. PMC 2949280. PMID 19015660.
- ^ Maher CA, Kumar-Sinha C, Cao X, Kalyana-Sundaram S, Han B, Jing X, et al. (March 2009). "Transcriptome sequencing to detect gene fusions in cancer". Nature. 458 (7234): 97–101. Bibcode:2009Natur.458...97M. doi:10.1038/nature07638. PMC 2725402. PMID 19136943.
- Ingolia NT, Brar GA, Rouskin S, McGeachy AM, Weissman JS (July 2012). "The ribosome profiling strategy for monitoring translation in vivo by deep sequencing of ribosome-protected mRNA fragments". Nature Protocols. 7 (8): 1534–50. doi:10.1038/nprot.2012.086. PMC 3535016. PMID 22836135.
- Alpern D, Gardeux V, Russeil J, Mangeat B, Meireles-Filho AC, Breysse R, et al. (April 2019). "BRB-seq: ultra-affordable high-throughput transcriptomics enabled by bulk RNA barcoding and sequencing". Genome Biology. 20 (1): 71. doi:10.1186/s13059-019-1671-x. PMC 6474054. PMID 30999927.
- Lee JH, Daugharthy ER, Scheiman J, Kalhor R, Yang JL, Ferrante TC, et al. (March 2014). "Highly multiplexed subcellular RNA sequencing in situ". Science. 343 (6177): 1360–3. Bibcode:2014Sci...343.1360L. doi:10.1126/science.1250212. PMC 4140943. PMID 24578530.
- ^ Thind AS, Monga I, Thakur PK, Kumari P, Dindhoria K, Krzak M, et al. (November 2021). "Demystifying emerging bulk RNA-Seq applications: the application and utility of bioinformatic methodology". Briefings in Bioinformatics. 22 (6). doi:10.1093/bib/bbab259. PMID 34329375.
- Kukurba KR, Montgomery SB (April 2015). "RNA Sequencing and Analysis". Cold Spring Harbor Protocols. 2015 (11): 951–69. doi:10.1101/pdb.top084970. PMC 4863231. PMID 25870306.
- ^ Griffith M, Walker JR, Spies NC, Ainscough BJ, Griffith OL (August 2015). "Informatics for RNA Sequencing: A Web Resource for Analysis on the Cloud". PLOS Computational Biology. 11 (8): e1004393. Bibcode:2015PLSCB..11E4393G. doi:10.1371/journal.pcbi.1004393. PMC 4527835. PMID 26248053.
- "RNA-seqlopedia". rnaseq.uoregon.edu. Retrieved 8 February 2017.
- Morin R, Bainbridge M, Fejes A, Hirst M, Krzywinski M, Pugh T, et al. (July 2008). "Profiling the HeLa S3 transcriptome using randomly primed cDNA and massively parallel short-read sequencing". BioTechniques. 45 (1): 81–94. doi:10.2144/000112900. PMID 18611170.
- ^ Mortazavi A, Williams BA, McCue K, Schaeffer L, Wold B (July 2008). "Mapping and quantifying mammalian transcriptomes by RNA-Seq". Nature Methods. 5 (7): 621–8. doi:10.1038/nmeth.1226. PMID 18516045. S2CID 205418589.
- Sun Q, Hao Q, Prasanth KV (February 2018). "Nuclear Long Noncoding RNAs: Key Regulators of Gene Expression". Trends in Genetics. 34 (2): 142–157. doi:10.1016/j.tig.2017.11.005. PMC 6002860. PMID 29249332.
- Sigurgeirsson B, Emanuelsson O, Lundeberg J (2014). "Sequencing degraded RNA addressed by 3' tag counting". PLOS ONE. 9 (3): e91851. Bibcode:2014PLoSO...991851S. doi:10.1371/journal.pone.0091851. PMC 3954844. PMID 24632678.
- Chen EA, Souaiaia T, Herstein JS, Evgrafov OV, Spitsyna VN, Rebolini DF, et al. (October 2014). "Effect of RNA integrity on uniquely mapped reads in RNA-Seq". BMC Research Notes. 7: 753. doi:10.1186/1756-0500-7-753. PMC 4213542. PMID 25339126.
- Moll P, Ante M, Seitz A, Reda T (December 2014). "QuantSeq 3′ mRNA sequencing for RNA quantification". Nature Methods. 11 (12): i–iii. doi:10.1038/nmeth.f.376. ISSN 1548-7105. S2CID 83424788.
- Oikonomopoulos S, Bayega A, Fahiminiya S, Djambazian H, Berube P, Ragoussis J (2020). "Methodologies for Transcript Profiling Using Long-Read Technologies". Frontiers in Genetics. 11: 606. doi:10.3389/fgene.2020.00606. PMC 7358353. PMID 32733532.
- ^ Conesa A, Madrigal P, Tarazona S, Gomez-Cabrero D, Cervera A, McPherson A, et al. (January 2016). "A survey of best practices for RNA-seq data analysis". Genome Biology. 17 (1): 13. doi:10.1186/s13059-016-0881-8. PMC 4728800. PMID 26813401.
- Liu D, Graber JH (February 2006). "Quantitative comparison of EST libraries requires compensation for systematic biases in cDNA generation". BMC Bioinformatics. 7: 77. doi:10.1186/1471-2105-7-77. PMC 1431573. PMID 16503995.
- ^ Garalde DR, Snell EA, Jachimowicz D, Sipos B, Lloyd JH, Bruce M, et al. (March 2018). "Highly parallel direct RNA sequencing on an array of nanopores". Nature Methods. 15 (3): 201–206. doi:10.1038/nmeth.4577. PMID 29334379. S2CID 3589823.
- Liu D, Graber JH (February 2006). "Quantitative comparison of EST libraries requires compensation for systematic biases in cDNA generation". BMC Bioinformatics. 7: 77. doi:10.1186/1471-2105-7-77. PMC 1431573. PMID 16503995.
- Gleeson J, Lane TA, Harrison PJ, Haerty W, Clark MB (3 August 2020). "Nanopore direct RNA sequencing detects differential expression between human cell populations". bioRxiv: 2020.08.02.232785. doi:10.1101/2020.08.02.232785. S2CID 220975367.
- ^ "Shapiro E, Biezuner T, Linnarsson S (September 2013). "Single-cell sequencing-based technologies will revolutionize whole-organism science". Nature Reviews. Genetics. 14 (9): 618–30. doi:10.1038/nrg3542. PMID 23897237. S2CID 500845."
- Kolodziejczyk AA, Kim JK, Svensson V, Marioni JC, Teichmann SA (May 2015). "The technology and biology of single-cell RNA sequencing". Molecular Cell. 58 (4): 610–20. doi:10.1016/j.molcel.2015.04.005. PMID 26000846.
- Montoro DT, Haber AL, Biton M, Vinarsky V, Lin B, Birket SE, et al. (August 2018). "A revised airway epithelial hierarchy includes CFTR-expressing ionocytes". Nature. 560 (7718): 319–324. Bibcode:2018Natur.560..319M. doi:10.1038/s41586-018-0393-7. PMC 6295155. PMID 30069044.
- Plasschaert LW, Žilionis R, Choo-Wing R, Savova V, Knehr J, Roma G, et al. (August 2018). "A single-cell atlas of the airway epithelium reveals the CFTR-rich pulmonary ionocyte". Nature. 560 (7718): 377–381. Bibcode:2018Natur.560..377P. doi:10.1038/s41586-018-0394-6. PMC 6108322. PMID 30069046.
- Valihrach L, Androvic P, Kubista M (March 2018). "Platforms for Single-Cell Collection and Analysis". International Journal of Molecular Sciences. 19 (3): 807. doi:10.3390/ijms19030807. PMC 5877668. PMID 29534489.
- Klein AM, Mazutis L, Akartuna I, Tallapragada N, Veres A, Li V, et al. (May 2015). "Droplet barcoding for single-cell transcriptomics applied to embryonic stem cells". Cell. 161 (5): 1187–1201. doi:10.1016/j.cell.2015.04.044. PMC 4441768. PMID 26000487.
- Macosko EZ, Basu A, Satija R, Nemesh J, Shekhar K, Goldman M, et al. (May 2015). "Highly Parallel Genome-wide Expression Profiling of Individual Cells Using Nanoliter Droplets". Cell. 161 (5): 1202–1214. doi:10.1016/j.cell.2015.05.002. PMC 4481139. PMID 26000488.
- Islam S, Zeisel A, Joost S, La Manno G, Zajac P, Kasper M, et al. (February 2014). "Quantitative single-cell RNA-seq with unique molecular identifiers". Nature Methods. 11 (2): 163–6. doi:10.1038/nmeth.2772. PMID 24363023. S2CID 6765530.
- "Hebenstreit D (November 2012). "Methods, Challenges and Potentials of Single Cell RNA-seq". Biology. 1 (3): 658–67. doi:10.3390/biology1030658. PMC 4009822. PMID 24832513."
- Eberwine J, Sul JY, Bartfai T, Kim J (January 2014). "The promise of single-cell sequencing". Nature Methods. 11 (1): 25–7. doi:10.1038/nmeth.2769. PMID 24524134. S2CID 11575439.
- Tang F, Barbacioru C, Wang Y, Nordman E, Lee C, Xu N, et al. (May 2009). "mRNA-Seq whole-transcriptome analysis of a single cell". Nature Methods. 6 (5): 377–82. doi:10.1038/NMETH.1315. PMID 19349980. S2CID 16570747.
- Islam S, Kjällquist U, Moliner A, Zajac P, Fan JB, Lönnerberg P, et al. (July 2011). "Characterization of the single-cell transcriptional landscape by highly multiplex RNA-seq". Genome Research. 21 (7): 1160–7. doi:10.1101/gr.110882.110. PMC 3129258. PMID 21543516.
- Ramsköld D, Luo S, Wang YC, Li R, Deng Q, Faridani OR, et al. (August 2012). "Full-length mRNA-Seq from single-cell levels of RNA and individual circulating tumor cells". Nature Biotechnology. 30 (8): 777–82. doi:10.1038/nbt.2282. PMC 3467340. PMID 22820318.
- Hashimshony T, Wagner F, Sher N, Yanai I (September 2012). "CEL-Seq: single-cell RNA-Seq by multiplexed linear amplification". Cell Reports. 2 (3): 666–73. doi:10.1016/j.celrep.2012.08.003. PMID 22939981.
- Singh M, Al-Eryani G, Carswell S, Ferguson JM, Blackburn J, Barton K, et al. (2018). "High-throughput targeted long-read single cell sequencing reveals the clonal and transcriptional landscape of lymphocytes". bioRxiv. 10 (1): 3120. doi:10.1101/424945. PMC 6635368. PMID 31311926.
- Sasagawa Y, Nikaido I, Hayashi T, Danno H, Uno KD, Imai T, et al. (April 2013). "Quartz-Seq: a highly reproducible and sensitive single-cell RNA sequencing method, reveals non-genetic gene-expression heterogeneity". Genome Biology. 14 (4): R31. doi:10.1186/gb-2013-14-4-r31. PMC 4054835. PMID 23594475.
- Kouno T, Moody J, Kwon AT, Shibayama Y, Kato S, Huang Y, et al. (January 2019). "C1 CAGE detects transcription start sites and enhancer activity at single-cell resolution". Nature Communications. 10 (1): 360. Bibcode:2019NatCo..10..360K. doi:10.1038/s41467-018-08126-5. PMC 6341120. PMID 30664627.
- Dal Molin A, Di Camillo B (2019). "How to design a single-cell RNA-sequencing experiment: pitfalls, challenges and perspectives". Briefings in Bioinformatics. 20 (4): 1384–1394. doi:10.1093/bib/bby007. PMID 29394315.
- Peterson VM, Zhang KX, Kumar N, Wong J, Li L, Wilson DC, et al. (October 2017). "Multiplexed quantification of proteins and transcripts in single cells". Nature Biotechnology. 35 (10): 936–939. doi:10.1038/nbt.3973. PMID 28854175. S2CID 205285357.
- Stoeckius M, Hafemeister C, Stephenson W, Houck-Loomis B, Chattopadhyay PK, Swerdlow H, et al. (September 2017). "Simultaneous epitope and transcriptome measurement in single cells". Nature Methods. 14 (9): 865–868. doi:10.1038/nmeth.4380. PMC 5669064. PMID 28759029.
- Raj B, Wagner DE, McKenna A, Pandey S, Klein AM, Shendure J, et al. (June 2018). "Simultaneous single-cell profiling of lineages and cell types in the vertebrate brain". Nature Biotechnology. 36 (5): 442–450. doi:10.1038/nbt.4103. PMC 5938111. PMID 29608178.
- Olmos D, Arkenau HT, Ang JE, Ledaki I, Attard G, Carden CP, et al. (January 2009). "Circulating tumour cell (CTC) counts as intermediate end points in castration-resistant prostate cancer (CRPC): a single-centre experience". Annals of Oncology. 20 (1): 27–33. doi:10.1093/annonc/mdn544. PMID 18695026.
- Levitin HM, Yuan J, Sims PA (April 2018). "Single-Cell Transcriptomic Analysis of Tumor Heterogeneity". Trends in Cancer. 4 (4): 264–268. doi:10.1016/j.trecan.2018.02.003. PMC 5993208. PMID 29606308.
- Jerby-Arnon L, Shah P, Cuoco MS, Rodman C, Su MJ, Melms JC, et al. (November 2018). "A Cancer Cell Program Promotes T Cell Exclusion and Resistance to Checkpoint Blockade". Cell. 175 (4): 984–997.e24. doi:10.1016/j.cell.2018.09.006. PMC 6410377. PMID 30388455.
- Stephenson W, Donlin LT, Butler A, Rozo C, Bracken B, Rashidfarrokhi A, et al. (February 2018). "Single-cell RNA-seq of rheumatoid arthritis synovial tissue using low-cost microfluidic instrumentation". Nature Communications. 9 (1): 791. Bibcode:2018NatCo...9..791S. doi:10.1038/s41467-017-02659-x. PMC 5824814. PMID 29476078.
- Avraham R, Haseley N, Brown D, Penaranda C, Jijon HB, Trombetta JJ, et al. (September 2015). "Pathogen Cell-to-Cell Variability Drives Heterogeneity in Host Immune Responses". Cell. 162 (6): 1309–21. doi:10.1016/j.cell.2015.08.027. PMC 4578813. PMID 26343579.
- Cao J, Packer JS, Ramani V, Cusanovich DA, Huynh C, Daza R, et al. (August 2017). "Comprehensive single-cell transcriptional profiling of a multicellular organism". Science. 357 (6352): 661–667. Bibcode:2017Sci...357..661C. doi:10.1126/science.aam8940. PMC 5894354. PMID 28818938.
- Plass M, Solana J, Wolf FA, Ayoub S, Misios A, Glažar P, et al. (May 2018). "Cell type atlas and lineage tree of a whole complex animal by single-cell transcriptomics". Science. 360 (6391): eaaq1723. doi:10.1126/science.aaq1723. PMID 29674432.
- Fincher CT, Wurtzel O, de Hoog T, Kravarik KM, Reddien PW (May 2018). "Schmidtea mediterranea". Science. 360 (6391): eaaq1736. doi:10.1126/science.aaq1736. PMC 6563842. PMID 29674431.
- Wagner DE, Weinreb C, Collins ZM, Briggs JA, Megason SG, Klein AM (June 2018). "Single-cell mapping of gene expression landscapes and lineage in the zebrafish embryo". Science. 360 (6392): 981–987. Bibcode:2018Sci...360..981W. doi:10.1126/science.aar4362. PMC 6083445. PMID 29700229.
- Farrell JA, Wang Y, Riesenfeld SJ, Shekhar K, Regev A, Schier AF (June 2018). "Single-cell reconstruction of developmental trajectories during zebrafish embryogenesis". Science. 360 (6392): eaar3131. doi:10.1126/science.aar3131. PMC 6247916. PMID 29700225.
- Briggs JA, Weinreb C, Wagner DE, Megason S, Peshkin L, Kirschner MW, et al. (June 2018). "The dynamics of gene expression in vertebrate embryogenesis at single-cell resolution". Science. 360 (6392): eaar5780. doi:10.1126/science.aar5780. PMC 6038144. PMID 29700227.
- You J. "Science's 2018 Breakthrough of the Year: tracking development cell by cell". Science Magazine. American Association for the Advancement of Science.
- ^ Li H, Lovci MT, Kwon YS, Rosenfeld MG, Fu XD, Yeo GW (December 2008). "Determination of tag density required for digital transcriptome analysis: application to an androgen-sensitive prostate cancer model". Proceedings of the National Academy of Sciences of the United States of America. 105 (51): 20179–84. Bibcode:2008PNAS..10520179L. doi:10.1073/pnas.0807121105. PMC 2603435. PMID 19088194.
- ^ Stegle O, Parts L, Piipari M, Winn J, Durbin R (February 2012). "Using probabilistic estimation of expression residuals (PEER) to obtain increased power and interpretability of gene expression analyses". Nature Protocols. 7 (3): 500–7. doi:10.1038/nprot.2011.457. PMC 3398141. PMID 22343431.
- Kingsford C, Patro R (June 2015). "Reference-based compression of short-read sequences using path encoding". Bioinformatics. 31 (12): 1920–8. doi:10.1093/bioinformatics/btv071. PMC 4481695. PMID 25649622.
- ^ Grabherr MG, Haas BJ, Yassour M, Levin JZ, Thompson DA, Amit I, et al. (May 2011). "Full-length transcriptome assembly from RNA-Seq data without a reference genome". Nature Biotechnology. 29 (7): 644–52. doi:10.1038/nbt.1883. PMC 3571712. PMID 21572440.
- "De Novo Assembly Using Illumina Reads" (PDF). Retrieved 22 October 2016.
- Oases: a transcriptome assembler for very short reads
- Zerbino DR, Birney E (May 2008). "Velvet: algorithms for de novo short read assembly using de Bruijn graphs". Genome Research. 18 (5): 821–9. doi:10.1101/gr.074492.107. PMC 2336801. PMID 18349386.
- Chang Z, Li G, Liu J, Zhang Y, Ashby C, Liu D, et al. (February 2015). "Bridger: a new framework for de novo transcriptome assembly using RNA-seq data". Genome Biology. 16 (1): 30. doi:10.1186/s13059-015-0596-2. PMC 4342890. PMID 25723335.
- Bushmanova E, Antipov D, Lapidus A, Prjibelski AD (September 2019). "rnaSPAdes: a de novo transcriptome assembler and its application to RNA-Seq data". GigaScience. 8 (9). doi:10.1093/gigascience/giz100. PMC 6736328. PMID 31494669.
- ^ Li B, Fillmore N, Bai Y, Collins M, Thomson JA, Stewart R, et al. (December 2014). "Evaluation of de novo transcriptome assemblies from RNA-Seq data". Genome Biology. 15 (12): 553. doi:10.1186/s13059-014-0553-5. PMC 4298084. PMID 25608678.
- ^ Dobin A, Davis CA, Schlesinger F, Drenkow J, Zaleski C, Jha S, et al. (January 2013). "STAR: ultrafast universal RNA-seq aligner". Bioinformatics. 29 (1): 15–21. doi:10.1093/bioinformatics/bts635. PMC 3530905. PMID 23104886.
- Langmead B, Trapnell C, Pop M, Salzberg SL (2009). "Ultrafast and memory-efficient alignment of short DNA sequences to the human genome". Genome Biology. 10 (3): R25. doi:10.1186/gb-2009-10-3-r25. PMC 2690996. PMID 19261174.
- Trapnell C, Pachter L, Salzberg SL (May 2009). "TopHat: discovering splice junctions with RNA-Seq". Bioinformatics. 25 (9): 1105–11. doi:10.1093/bioinformatics/btp120. PMC 2672628. PMID 19289445.
- ^ Trapnell C, Roberts A, Goff L, Pertea G, Kim D, Kelley DR, et al. (March 2012). "Differential gene and transcript expression analysis of RNA-seq experiments with TopHat and Cufflinks". Nature Protocols. 7 (3): 562–78. doi:10.1038/nprot.2012.016. PMC 3334321. PMID 22383036.
- Liao Y, Smyth GK, Shi W (May 2013). "The Subread aligner: fast, accurate and scalable read mapping by seed-and-vote". Nucleic Acids Research. 41 (10): e108. doi:10.1093/nar/gkt214. PMC 3664803. PMID 23558742.
- Kim D, Langmead B, Salzberg SL (April 2015). "HISAT: a fast spliced aligner with low memory requirements". Nature Methods. 12 (4): 357–60. doi:10.1038/nmeth.3317. PMC 4655817. PMID 25751142.
- Wu TD, Watanabe CK (May 2005). "GMAP: a genomic mapping and alignment program for mRNA and EST sequences". Bioinformatics. 21 (9): 1859–75. doi:10.1093/bioinformatics/bti310. PMID 15728110.
- Pertea M, Pertea GM, Antonescu CM, Chang TC, Mendell JT, Salzberg SL (March 2015). "StringTie enables improved reconstruction of a transcriptome from RNA-seq reads". Nature Biotechnology. 33 (3): 290–5. doi:10.1038/nbt.3122. PMC 4643835. PMID 25690850.
- Baruzzo G, Hayer KE, Kim EJ, Di Camillo B, FitzGerald GA, Grant GR (February 2017). "Simulation-based comprehensive benchmarking of RNA-seq aligners". Nature Methods. 14 (2): 135–139. doi:10.1038/nmeth.4106. PMC 5792058. PMID 27941783.
- Engström PG, Steijger T, Sipos B, Grant GR, Kahles A, Rätsch G, et al. (December 2013). "Systematic evaluation of spliced alignment programs for RNA-seq data". Nature Methods. 10 (12): 1185–91. doi:10.1038/nmeth.2722. PMC 4018468. PMID 24185836.
- Lu B, Zeng Z, Shi T (February 2013). "Comparative study of de novo assembly and genome-guided assembly strategies for transcriptome reconstruction based on RNA-Seq". Science China Life Sciences. 56 (2): 143–55. doi:10.1007/s11427-013-4442-z. PMID 23393030.
- Bradnam KR, Fass JN, Alexandrov A, Baranay P, Bechner M, Birol I, et al. (July 2013). "Assemblathon 2: evaluating de novo methods of genome assembly in three vertebrate species". GigaScience. 2 (1): 10. arXiv:1301.5406. Bibcode:2013arXiv1301.5406B. doi:10.1186/2047-217X-2-10. PMC 3844414. PMID 23870653.
- Hölzer M, Marz M (May 2019). "De novo transcriptome assembly: A comprehensive cross-species comparison of short-read RNA-Seq assemblers". GigaScience. 8 (5). doi:10.1093/gigascience/giz039. PMC 6511074. PMID 31077315.
- Greenbaum D, Colangelo C, Williams K, Gerstein M (2003). "Comparing protein abundance and mRNA expression levels on a genomic scale". Genome Biology. 4 (9): 117. doi:10.1186/gb-2003-4-9-117. PMC 193646. PMID 12952525.
- Zhang ZH, Jhaveri DJ, Marshall VM, Bauer DC, Edson J, Narayanan RK, et al. (August 2014). "A comparative study of techniques for differential expression analysis on RNA-Seq data". PLOS ONE. 9 (8): e103207. Bibcode:2014PLoSO...9j3207Z. doi:10.1371/journal.pone.0103207. PMC 4132098. PMID 25119138.
- Anders S, Pyl PT, Huber W (January 2015). "HTSeq--a Python framework to work with high-throughput sequencing data". Bioinformatics. 31 (2): 166–9. doi:10.1093/bioinformatics/btu638. PMC 4287950. PMID 25260700.
- Liao Y, Smyth GK, Shi W (April 2014). "featureCounts: an efficient general purpose program for assigning sequence reads to genomic features". Bioinformatics. 30 (7): 923–30. arXiv:1305.3347. doi:10.1093/bioinformatics/btt656. PMID 24227677.
- Schmid MW, Grossniklaus U (February 2015). "Rcount: simple and flexible RNA-Seq read counting". Bioinformatics. 31 (3): 436–7. doi:10.1093/bioinformatics/btu680. PMID 25322836.
- Finotello F, Lavezzo E, Bianco L, Barzon L, Mazzon P, Fontana P, et al. (2014). "Reducing bias in RNA sequencing data: a novel approach to compute counts". BMC Bioinformatics. 15 (Suppl 1): S7. doi:10.1186/1471-2105-15-s1-s7. PMC 4016203. PMID 24564404.
- Hashimoto TB, Edwards MD, Gifford DK (March 2014). "Universal count correction for high-throughput sequencing". PLOS Computational Biology. 10 (3): e1003494. Bibcode:2014PLSCB..10E3494H. doi:10.1371/journal.pcbi.1003494. PMC 3945112. PMID 24603409.
- Patro R, Mount SM, Kingsford C (May 2014). "Sailfish enables alignment-free isoform quantification from RNA-seq reads using lightweight algorithms". Nature Biotechnology. 32 (5): 462–4. arXiv:1308.3700. doi:10.1038/nbt.2862. PMC 4077321. PMID 24752080.
- Bray NL, Pimentel H, Melsted P, Pachter L (May 2016). "Near-optimal probabilistic RNA-seq quantification". Nature Biotechnology. 34 (5): 525–7. doi:10.1038/nbt.3519. PMID 27043002. S2CID 205282743.
- ^ Robinson MD, Oshlack A (2010). "A scaling normalization method for differential expression analysis of RNA-seq data". Genome Biology. 11 (3): R25. doi:10.1186/gb-2010-11-3-r25. PMC 2864565. PMID 20196867.
- Trapnell C, Williams BA, Pertea G, Mortazavi A, Kwan G, van Baren MJ, et al. (May 2010). "Transcript assembly and quantification by RNA-Seq reveals unannotated transcripts and isoform switching during cell differentiation". Nature Biotechnology. 28 (5): 511–5. doi:10.1038/nbt.1621. PMC 3146043. PMID 20436464.
- ^ Pachter L (19 April 2011). "Models for transcript quantification from RNA-Seq". arXiv:1104.3889 .
- "What the FPKM? A review of RNA-Seq expression units". The farrago. 8 May 2014. Retrieved 28 March 2018.
- Wagner GP, Kin K, Lynch VJ (December 2012). "Measurement of mRNA abundance using RNA-seq data: RPKM measure is inconsistent among samples". Theory in Biosciences. 131 (4): 281–5. doi:10.1007/s12064-012-0162-3. PMID 22872506. S2CID 16752581.
- Evans C, Hardin J, Stoebel DM (September 2018). "Selecting between-sample RNA-Seq normalization methods from the perspective of their assumptions". Briefings in Bioinformatics. 19 (5): 776–792. doi:10.1093/bib/bbx008. PMC 6171491. PMID 28334202.
- ^ Law CW, Chen Y, Shi W, Smyth GK (February 2014). "voom: Precision weights unlock linear model analysis tools for RNA-seq read counts". Genome Biology. 15 (2): R29. doi:10.1186/gb-2014-15-2-r29. PMC 4053721. PMID 24485249.
- ^ Anders S, Huber W (2010). "Differential expression analysis for sequence count data". Genome Biology. 11 (10): R106. doi:10.1186/gb-2010-11-10-r106. PMC 3218662. PMID 20979621.
- ^ Robinson MD, McCarthy DJ, Smyth GK (January 2010). "edgeR: a Bioconductor package for differential expression analysis of digital gene expression data". Bioinformatics. 26 (1): 139–40. doi:10.1093/bioinformatics/btp616. PMC 2796818. PMID 19910308.
- Marguerat S, Schmidt A, Codlin S, Chen W, Aebersold R, Bähler J (October 2012). "Quantitative analysis of fission yeast transcriptomes and proteomes in proliferating and quiescent cells". Cell. 151 (3): 671–83. doi:10.1016/j.cell.2012.09.019. PMC 3482660. PMID 23101633.
- Owens ND, Blitz IL, Lane MA, Patrushev I, Overton JD, Gilchrist MJ, et al. (January 2016). "Measuring Absolute RNA Copy Numbers at High Temporal Resolution Reveals Transcriptome Kinetics in Development". Cell Reports. 14 (3): 632–647. doi:10.1016/j.celrep.2015.12.050. PMC 4731879. PMID 26774488.
- Chen K, Hu Z, Xia Z, Zhao D, Li W, Tyler JK (December 2015). "The Overlooked Fact: Fundamental Need for Spike-In Control for Virtually All Genome-Wide Analyses". Molecular and Cellular Biology. 36 (5): 662–7. doi:10.1128/MCB.00970-14. PMC 4760223. PMID 26711261.
- Lovén J, Orlando DA, Sigova AA, Lin CY, Rahl PB, Burge CB, et al. (October 2012). "Revisiting global gene expression analysis". Cell. 151 (3): 476–82. doi:10.1016/j.cell.2012.10.012. PMC 3505597. PMID 23101621.
- Ritchie ME, Phipson B, Wu D, Hu Y, Law CW, Shi W, et al. (April 2015). "limma powers differential expression analyses for RNA-sequencing and microarray studies". Nucleic Acids Research. 43 (7): e47. doi:10.1093/nar/gkv007. PMC 4402510. PMID 25605792.
- "Bioconductor - Open source software for bioinformatics".
- Huber W, Carey VJ, Gentleman R, Anders S, Carlson M, Carvalho BS, et al. (February 2015). "Orchestrating high-throughput genomic analysis with Bioconductor". Nature Methods. 12 (2): 115–21. doi:10.1038/nmeth.3252. PMC 4509590. PMID 25633503.
- Leek JT, Storey JD (September 2007). "Capturing heterogeneity in gene expression studies by surrogate variable analysis". PLOS Genetics. 3 (9): 1724–35. doi:10.1371/journal.pgen.0030161. PMC 1994707. PMID 17907809.
- Pimentel H, Bray NL, Puente S, Melsted P, Pachter L (July 2017). "Differential analysis of RNA-seq incorporating quantification uncertainty". Nature Methods. 14 (7): 687–690. doi:10.1038/nmeth.4324. PMID 28581496. S2CID 15063247.
- Trapnell C, Hendrickson DG, Sauvageau M, Goff L, Rinn JL, Pachter L (January 2013). "Differential analysis of gene regulation at transcript resolution with RNA-seq". Nature Biotechnology. 31 (1): 46–53. doi:10.1038/nbt.2450. PMC 3869392. PMID 23222703.
- Frazee AC, Pertea G, Jaffe AE, Langmead B, Salzberg SL, Leek JT (March 2015). "Ballgown bridges the gap between transcriptome assembly and expression analysis". Nature Biotechnology. 33 (3): 243–6. doi:10.1038/nbt.3172. PMC 4792117. PMID 25748911.
- ^ Sahraeian SM, Mohiyuddin M, Sebra R, Tilgner H, Afshar PT, Au KF, et al. (July 2017). "Gaining comprehensive biological insight into the transcriptome by performing a broad-spectrum RNA-seq analysis". Nature Communications. 8 (1): 59. Bibcode:2017NatCo...8...59S. doi:10.1038/s41467-017-00050-4. PMC 5498581. PMID 28680106.
- Ziemann M, Eren Y, El-Osta A (August 2016). "Gene name errors are widespread in the scientific literature". Genome Biology. 17 (1): 177. doi:10.1186/s13059-016-1044-7. PMC 4994289. PMID 27552985.
- Soneson C, Delorenzi M (March 2013). "A comparison of methods for differential expression analysis of RNA-seq data". BMC Bioinformatics. 14: 91. doi:10.1186/1471-2105-14-91. PMC 3608160. PMID 23497356.
- Fonseca NA, Marioni J, Brazma A (30 September 2014). "RNA-Seq gene profiling--a systematic empirical comparison". PLOS ONE. 9 (9): e107026. Bibcode:2014PLoSO...9j7026F. doi:10.1371/journal.pone.0107026. PMC 4182317. PMID 25268973.
- Seyednasrollah F, Laiho A, Elo LL (January 2015). "Comparison of software packages for detecting differential expression in RNA-seq studies". Briefings in Bioinformatics. 16 (1): 59–70. doi:10.1093/bib/bbt086. PMC 4293378. PMID 24300110.
- Rapaport F, Khanin R, Liang Y, Pirun M, Krek A, Zumbo P, et al. (2013). "Comprehensive evaluation of differential gene expression analysis methods for RNA-seq data". Genome Biology. 14 (9): R95. doi:10.1186/gb-2013-14-9-r95. PMC 4054597. PMID 24020486.
- Costa-Silva J, Domingues D, Lopes FM (21 December 2017). "RNA-Seq differential expression analysis: An extended review and a software tool". PLOS ONE. 12 (12): e0190152. Bibcode:2017PLoSO..1290152C. doi:10.1371/journal.pone.0190152. PMC 5739479. PMID 29267363.
- Corchete LA, Rojas EA, Alonso-López D, De Las Rivas J, Gutiérrez NC, Burguillo FJ (12 November 2020). "Systematic comparison and assessment of RNA-seq procedures for gene expression quantitative analysis". Scientific Reports. 12 (10): 19737. Bibcode:2020NatSR..1019737C. doi:10.1038/s41598-020-76881-x. PMC 7665074. PMID 33184454.
- Liao Y, Wang J, Jaehnig EJ, Shi Z, Zhang B (July 2019). "WebGestalt 2019: gene set analysis toolkit with revamped UIs and APIs". Nucleic Acids Research. 47 (W1): W199 – W205. doi:10.1093/nar/gkz401. PMC 6602449. PMID 31114916.
- ^ Keren H, Lev-Maor G, Ast G (May 2010). "Alternative splicing and evolution: diversification, exon definition and function". Nature Reviews. Genetics. 11 (5): 345–55. doi:10.1038/nrg2776. PMID 20376054. S2CID 5184582.
- Liu R, Loraine AE, Dickerson JA (December 2014). "Comparisons of computational methods for differential alternative splicing detection using RNA-seq in plant systems". BMC Bioinformatics. 15 (1): 364. doi:10.1186/s12859-014-0364-4. PMC 4271460. PMID 25511303.
- ^ Li YI, Knowles DA, Humphrey J, Barbeira AN, Dickinson SP, Im HK, et al. (January 2018). "Annotation-free quantification of RNA splicing using LeafCutter". Nature Genetics. 50 (1): 151–158. doi:10.1038/s41588-017-0004-9. PMC 5742080. PMID 29229983.
- Anders S, Reyes A, Huber W (October 2012). "Detecting differential usage of exons from RNA-seq data". Genome Research. 22 (10): 2008–17. doi:10.1101/gr.133744.111. PMC 3460195. PMID 22722343.
- Shen S, Park JW, Huang J, Dittmar KA, Lu ZX, Zhou Q, et al. (April 2012). "MATS: a Bayesian framework for flexible detection of differential alternative splicing from RNA-Seq data". Nucleic Acids Research. 40 (8): e61. doi:10.1093/nar/gkr1291. PMC 3333886. PMID 22266656.
- Wang X, Cairns MJ (June 2014). "SeqGSEA: a Bioconductor package for gene set enrichment analysis of RNA-Seq data integrating differential expression and splicing". Bioinformatics. 30 (12): 1777–9. doi:10.1093/bioinformatics/btu090. PMID 24535097.
- Trapnell C, Hendrickson DG, Sauvageau M, Goff L, Rinn JL, Pachter L (January 2013). "Differential analysis of gene regulation at transcript resolution with RNA-seq". Nature Biotechnology. 31 (1): 46–53. doi:10.1038/nbt.2450. PMC 3869392. PMID 23222703.
- Hu Y, Huang Y, Du Y, Orellana CF, Singh D, Johnson AR, et al. (January 2013). "DiffSplice: the genome-wide detection of differential splicing events with RNA-seq". Nucleic Acids Research. 41 (2): e39. doi:10.1093/nar/gks1026. PMC 3553996. PMID 23155066.
- Vaquero-Garcia J, Barrera A, Gazzara MR, González-Vallinas J, Lahens NF, Hogenesch JB, et al. (February 2016). "A new view of transcriptome complexity and regulation through the lens of local splicing variations". eLife. 5: e11752. doi:10.7554/eLife.11752. PMC 4801060. PMID 26829591.
- Merino GA, Conesa A, Fernández EA (March 2019). "A benchmarking of workflows for detecting differential splicing and differential expression at isoform level in human RNA-seq studies". Briefings in Bioinformatics. 20 (2): 471–481. doi:10.1093/bib/bbx122. hdl:11336/41247. PMID 29040385. S2CID 22706028.
- ^ Marcotte EM, Pellegrini M, Thompson MJ, Yeates TO, Eisenberg D (November 1999). "A combined algorithm for genome-wide prediction of protein function". Nature. 402 (6757): 83–6. Bibcode:1999Natur.402...83M. doi:10.1038/47048. PMID 10573421. S2CID 144447.
- ^ Giorgi FM, Del Fabbro C, Licausi F (March 2013). "Comparative study of RNA-seq- and microarray-derived coexpression networks in Arabidopsis thaliana". Bioinformatics. 29 (6): 717–24. doi:10.1093/bioinformatics/btt053. hdl:11390/990155. PMID 23376351.
- Iancu OD, Kawane S, Bottomly D, Searles R, Hitzemann R, McWeeney S (June 2012). "Utilizing RNA-Seq data for de novo coexpression network inference". Bioinformatics. 28 (12): 1592–7. doi:10.1093/bioinformatics/bts245. PMC 3493127. PMID 22556371.
- Eksi R, Li HD, Menon R, Wen Y, Omenn GS, Kretzler M, et al. (November 2013). "Systematically differentiating functions for alternatively spliced isoforms through integrating RNA-seq data". PLOS Computational Biology. 9 (11): e1003314. Bibcode:2013PLSCB...9E3314E. doi:10.1371/journal.pcbi.1003314. PMC 3820534. PMID 24244129.
- Li HD, Menon R, Omenn GS, Guan Y (August 2014). "The emerging era of genomic data integration for analyzing splice isoform function". Trends in Genetics. 30 (8): 340–7. doi:10.1016/j.tig.2014.05.005. PMC 4112133. PMID 24951248.
- Foroushani A, Agrahari R, Docking R, Chang L, Duns G, Hudoba M, et al. (March 2017). "Large-scale gene network analysis reveals the significance of extracellular matrix pathway and homeobox genes in acute myeloid leukemia: an introduction to the Pigengene package and its applications". BMC Medical Genomics. 10 (1): 16. doi:10.1186/s12920-017-0253-6. PMC 5353782. PMID 28298217.
- Li H, Handsaker B, Wysoker A, Fennell T, Ruan J, Homer N, et al. (August 2009). "The Sequence Alignment/Map format and SAMtools". Bioinformatics. 25 (16): 2078–9. doi:10.1093/bioinformatics/btp352. PMC 2723002. PMID 19505943.
- DePristo MA, Banks E, Poplin R, Garimella KV, Maguire JR, Hartl C, et al. (May 2011). "A framework for variation discovery and genotyping using next-generation DNA sequencing data". Nature Genetics. 43 (5): 491–8. doi:10.1038/ng.806. PMC 3083463. PMID 21478889.
- Battle A, Brown CD, Engelhardt BE, Montgomery SB (October 2017). "Genetic effects on gene expression across human tissues". Nature. 550 (7675): 204–213. Bibcode:2017Natur.550..204A. doi:10.1038/nature24277. hdl:10230/34202. PMC 5776756. PMID 29022597.
- Richter F, Hoffman GE, Manheimer KB, Patel N, Sharp AJ, McKean D, et al. (October 2019). "ORE identifies extreme expression effects enriched for rare variants". Bioinformatics. 35 (20): 3906–3912. doi:10.1093/bioinformatics/btz202. PMC 6792115. PMID 30903145.
- Freedman AH, Clamp M, Sackton TB (January 2021). "Error, noise and bias in de novo transcriptome assemblies". Molecular Ecology Resources. 21 (1): 18–29. doi:10.1111/1755-0998.13156. PMID 32180366. S2CID 212739959.
- Teixeira MR (December 2006). "Recurrent fusion oncogenes in carcinomas". Critical Reviews in Oncogenesis. 12 (3–4): 257–71. doi:10.1615/critrevoncog.v12.i3-4.40. PMID 17425505. S2CID 40770452.
- Thind AS, Monga I, Thakur PK, Kumari P, Dindhoria K, Krzak M, et al. (November 2021). "Demystifying emerging bulk RNA-Seq applications: the application and utility of bioinformatic methodology". Briefings in Bioinformatics. 22 (6). doi:10.1093/bib/bbab259. PMID 34329375.
- Sangiovanni M, Granata I, Thind AS, Guarracino MR (April 2019). "From trash to treasure: detecting unexpected contamination in unmapped NGS data". BMC Bioinformatics. 20 (Suppl 4): 168. doi:10.1186/s12859-019-2684-x. PMC 6472186. PMID 30999839.
- "PubMed search: "RNA Seq" OR "RNA-Seq" OR "RNA sequencing" OR "RNASeq"". PubMed. Retrieved 20 June 2021.
- "PubMed search: ("RNA Seq" OR "RNA-Seq" OR "RNA sequencing" OR "RNASeq") AND "Medicine"". PubMed. Retrieved 20 June 2021.
- Weber AP (November 2015). "Discovering New Biology through Sequencing of RNA". Plant Physiology. 169 (3): 1524–31. doi:10.1104/pp.15.01081. PMC 4634082. PMID 26353759.
- Bainbridge MN, Warren RL, Hirst M, Romanuik T, Zeng T, Go A, et al. (September 2006). "Analysis of the prostate cancer cell line LNCaP transcriptome using a sequencing-by-synthesis approach". BMC Genomics. 7: 246. doi:10.1186/1471-2164-7-246. PMC 1592491. PMID 17010196.
- Cheung F, Haas BJ, Goldberg SM, May GD, Xiao Y, Town CD (October 2006). "Sequencing Medicago truncatula expressed sequenced tags using 454 Life Sciences technology". BMC Genomics. 7: 272. doi:10.1186/1471-2164-7-272. PMC 1635983. PMID 17062153.
- Emrich SJ, Barbazuk WB, Li L, Schnable PS (January 2007). "Gene discovery and annotation using LCM-454 transcriptome sequencing". Genome Research. 17 (1): 69–73. doi:10.1101/gr.5145806. PMC 1716268. PMID 17095711.
- Weber AP, Weber KL, Carr K, Wilkerson C, Ohlrogge JB (May 2007). "Sampling the Arabidopsis transcriptome with massively parallel pyrosequencing". Plant Physiology. 144 (1): 32–42. doi:10.1104/pp.107.096677. PMC 1913805. PMID 17351049.
- Nagalakshmi U, Wang Z, Waern K, Shou C, Raha D, Gerstein M, et al. (June 2008). "The transcriptional landscape of the yeast genome defined by RNA sequencing". Science. 320 (5881): 1344–9. Bibcode:2008Sci...320.1344N. doi:10.1126/science.1158441. PMC 2951732. PMID 18451266.
- Richter F (2021). "A broad introduction to RNA-Seq". WikiJournal of Science. 4 (1): 4. doi:10.15347/WJS/2021.004.
- Cummings BB, Marshall JL, Tukiainen T, Lek M, Donkervoort S, Foley AR, et al. (19 April 2017). "Improving genetic diagnosis in Mendelian disease with transcriptome sequencing". Science Translational Medicine. 9 (386). doi:10.1126/scitranslmed.aal5209. hdl:10230/35912. PMC 5548421. PMID 28424332.
- Kremer LS, Bader DM, Mertes C, Kopajtich R, Pichler G, Iuso A, et al. (12 June 2017). "Genetic diagnosis of Mendelian disorders via RNA sequencing". Nature Communications. 8 (1): 15824. Bibcode:2017NatCo...815824K. doi:10.1038/ncomms15824. PMC 5499207. PMID 28604674.
- Sandberg R (January 2014). "Entering the era of single-cell transcriptomics in biology and medicine". Nature Methods. 11 (1): 22–4. doi:10.1038/nmeth.2764. PMID 24524133. S2CID 27632439.
- "ENCODE Data Matrix". Retrieved 28 July 2013.
- "The Cancer Genome Atlas – Data Portal". Retrieved 28 July 2013.
Further reading
- Taguchi Y (2019). "Comparative Transcriptomics Analysis". Encyclopedia of Bioinformatics and Computational Biology. pp. 814–818. doi:10.1016/B978-0-12-809633-8.20163-5. ISBN 978-0-12-811432-2. S2CID 65302519.
External links

- Cresko B, Voelker R, Small C (2001). Bassham S, Catchen J (eds.). "RNA-seqlopedia". University of Oregon.: a high-level guide to designing and implementing an RNA-Seq experiment.