An amplicon sequence variant (ASV) is any one of the inferred single DNA sequences recovered from a high-throughput analysis of marker genes. Because these analyses, also called "amplicon reads," are created following the removal of erroneous sequences generated during PCR and sequencing, using ASVs makes it possible to distinguish sequence variation by a single nucleotide change. The uses of ASVs include classifying groups of species based on DNA sequences, finding biological and environmental variation, and determining ecological patterns.
ASVs were first described in 2013, by Eren and colleagues. Before that, for many years the standard unit for marker-gene analysis was the operational taxonomic unit (OTU), which is generated by clustering sequences based on a threshold of similarity. Compared to ASVs, OTUs reflect a coarser notion of similarity. Though there is no single threshold, the most commonly chosen value is 3%, which means these units share 97% of the DNA sequence. ASV methods on the other hand are able to resolve sequence differences by as little as a single nucleotide change, thus avoiding similarity-based operational clustering units altogether. Therefore, ASVs represent a finer distinction between sequences.
ASVs are also referred to as exact sequence variants (ESVs), zero-radius OTUs (ZOTUs), sub-OTUs (sOTUs), haplotypes, or oligotypes.
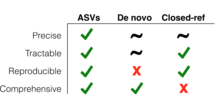
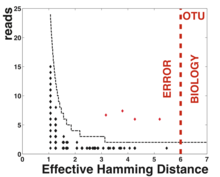



Uses of ASVs versus OTUs
The introduction of ASV methods was marked by a debate about their utility. Although OTUs do not provide such precise and accurate measurements of sequence variation, they are still an acceptable and valuable approach. In one research study, Glassman and Martiny confirmed the suitability of OTUs for investigating broad-scale ecological diversity. They concluded that OTUs and ASVs provided similar results, with ASVs enabling a slightly stronger detection of fungal and bacterial diversity. And their work indicated that even though species diversification can be measured more accurately with ASVs, the use of OTUs in well-constructed studies is generally valid to demonstrate diversification at broad scales.
Some have argued that ASVs should replace OTUs in marker-gene analysis. Their arguments focus on the precision, tractability, reproducibility, and comprehensiveness they can bring to marker-gene analysis. For these researchers, the utility of finer sequence resolution (precision) and the advantage of being able to easily compare sequences between different studies (tractability and reproducibility) make ASVs the better option for analyzing sequence differences. By contrast, since OTUs depend on the specifics of the similarity thresholds used to generate them, the units within any OTU can vary across researchers, experiments, and databases. Thus comparison across OTU-based studies and datasets can be very challenging.
ASV methods
Popular methods for resolving ASVs including DADA2, Deblur, MED, and UNOISE. These methods work broadly by generating an error model tailored to an individual sequencing run and employing algorithms that use the model to distinguish between true biological sequences and those generated by error.
References
- Eren AM, Maignien L, Sul WJ, Murphy LG, Grim SL, Morrison HG, Sogin ML (December 2013). "Oligotyping: Differentiating between closely related microbial taxa using 16S rRNA gene data". Methods in Ecology and Evolution. 4 (12): 1111–1119. Bibcode:2013MEcEv...4.1111E. doi:10.1111/2041-210X.12114. hdl:1912/6377. PMC 3864673. PMID 24358444.
- Porter TM, Hajibabaei M (January 2018). "Scaling up: A guide to high-throughput genomic approaches for biodiversity analysis". Molecular Ecology. 27 (2): 313–338. Bibcode:2018MolEc..27..313P. doi:10.1111/mec.14478. PMID 29292539.
- ^ Callahan BJ, McMurdie PJ, Holmes SP (December 2017). "Exact sequence variants should replace operational taxonomic units in marker-gene data analysis". The ISME Journal. 11 (12): 2639–2643. Bibcode:2017ISMEJ..11.2639C. doi:10.1038/ismej.2017.119. PMC 5702726. PMID 28731476.
- Glassman SI, Martiny JB (July 2018). "Broadscale Ecological Patterns Are Robust to Use of Exact Sequence Variants versus Operational Taxonomic Units". mSphere. 3 (4). doi:10.1128/mSphere.00148-18. PMC 6052340. PMID 30021874.
- Callahan BJ, McMurdie PJ, Rosen MJ, Han AW, Johnson AJ, Holmes (2015-08-06). "DADA2: High resolution sample inference from amplicon data". bioRxiv. doi:10.1101/024034.
- Amir A, McDonald D, Navas-Molina JA, Kopylova E, Morton JT, Zech Xu Z, et al. (2017-04-25). Gilbert JA (ed.). "Deblur Rapidly Resolves Single-Nucleotide Community Sequence Patterns". mSystems. 2 (2). doi:10.1128/mSystems.00191-16. PMC 5340863. PMID 28289731.
- Eren AM, Morrison HG, Lescault PJ, Reveillaud J, Vineis JH, Sogin ML (March 2015). "Minimum entropy decomposition: unsupervised oligotyping for sensitive partitioning of high-throughput marker gene sequences". The ISME Journal. 9 (4): 968–979. Bibcode:2015ISMEJ...9..968E. doi:10.1038/ismej.2014.195. PMC 4817710. PMID 25325381.
- Edgar RC (2016-10-15). "UNOISE2: improved error-correction for Illumina 16S and ITS amplicon sequencing". bioRxiv. doi:10.1101/081257.