The Minkowski distance or Minkowski metric is a metric in a normed vector space which can be considered as a generalization of both the Euclidean distance and the Manhattan distance. It is named after the Polish mathematician Hermann Minkowski.

Definition
The Minkowski distance of order
(where is an integer) between two points is defined as:For metric as a result of the Minkowski inequality. When the distance between and is but the point is at a distance from both of these points. Since this violates the triangle inequality, for it is not a metric. However, a metric can be obtained for these values by simply removing the exponent of The resulting metric is also an F-norm.
the Minkowski distance is aMinkowski distance is typically used with Manhattan distance and the Euclidean distance, respectively. In the limiting case of reaching infinity, we obtain the Chebyshev distance:
being 1 or 2, which correspond to theSimilarly, for
reaching negative infinity, we have:The Minkowski distance can also be viewed as a multiple of the power mean of the component-wise differences between and
The following figure shows unit circles (the level set of the distance function where all points are at the unit distance from the center) with various values of :
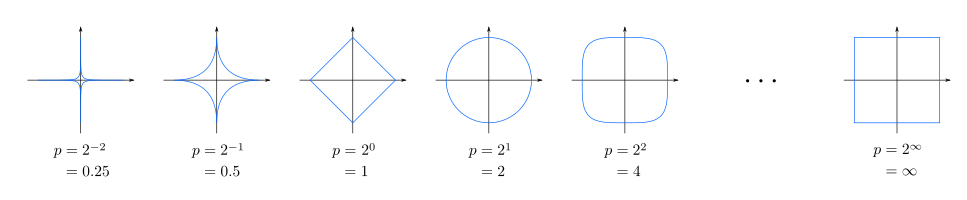
Applications
The Minkowski metric is very useful in the field of machine learning and AI. Many popular machine learning algorithms use specific distance metrics such as the aforementioned to compare the similarity of two data points. Depending on the nature of the data being analyzed, various metrics can be used. The Minkowski metric is most useful for numerical datasets where you want to determine the similarity of size between multiple datapoint vectors.
See also
- Generalized mean – N-th root of the arithmetic mean of the given numbers raised to the power n
- – Function spaces generalizing finite-dimensional p norm spaces space
- Norm (mathematics) – Length in a vector space
- – Function spaces generalizing finite-dimensional p norm spacesPages displaying short descriptions of redirect targets -norm
References
- Şuhubi, Erdoğan S. (2003), "Chapter V: Metric Spaces", Functional Analysis, Springer Netherlands, pp. 261–356, doi:10.1007/978-94-017-0141-9_5, ISBN 9789401701419
- Zezula, Pavel; Amato, Giuseppe; Dohnal, Vlastislav; Batko, Michal (2006), "Chapter 1, Foundations of Metric Space Searching, Section 3.1, Minkowski Distances", Similarity Search: The Metric Space Approach, Advances in Database Systems, Springer, p. 10, doi:10.1007/0-387-29151-2, ISBN 9780387291512
External links
- Unit Balls for Different p-Norms in 2D and 3D at wolfram.com
- Unit-Norm Vectors under Different p-Norms at wolfram.com
- Simple IEEE 754 implementation in C++
- NPM JavaScript Package/Module
Lp spaces | |||
---|---|---|---|
Basic concepts | |||
L spaces | |||
L spaces | |||
spaces | |||
Maps | |||
Inequalities | |||
Results |
| ||
Applications & related |