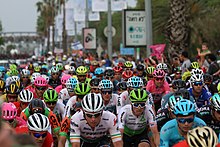
In a road bicycle race, the peloton (from French, originally meaning 'platoon') is the main group or pack of riders. Riders in a group save energy by riding close (drafting or slipstreaming) to (particularly behind) other riders. The reduction in drag is dramatic; riding in the middle of a well-developed group, drag can be reduced by as much as 95%. Exploitation of this potential energy saving leads to complex cooperative and competitive interactions between riders and teams in race tactics. The term is also used to refer to the community of professional cyclists in general.
Definition
More formally, a peloton is defined as "two or more cyclists riding in sufficiently close proximity to be located either in one of two basic positions: (1) behind cyclists in zones of reduced air pressure, referred to as ‘drafting’, or (2) in non-drafting positions where air pressure is highest. Cyclists in drafting zones expend less energy than in front positions." A peloton has similarly been defined "as a group of cyclists that are coupled together through the mutual energy benefits of drafting, whereby cyclists follow others in zones of reduced air resistance." A peloton is a complex system, which means that collective behavior emerges from simple rules of cyclists' interactions.
Formations

Pelotons are typically observed during bicycle races in which drafting is permitted, although pelotons also form from cyclist commuter traffic. Pelotons travel as an integrated unit in which each rider makes positional adjustments in response to the movements of adjacent riders and those ahead. Riders at the front are fully exposed to wind resistance, hence they experience higher fatigue loads than riders in drafting positions. After a period of time in front, leading riders maneuver farther back in the peloton to a drafting position to recover. Thus the peloton is in fluid motion as a continuous rotation of riders push from the back through to the leading edge, then falling away. Like bird flocks, peloton-like behavior that involves drafting or similar energy-saving mechanisms has been identified in a variety of biological systems.
The shape or formation of the peloton changes according to multiple factors. Comparatively high power output efforts due to high-speeds on flat topography, a strong headwind or inclines (hills) tends to spread out or lengthen the formation, often into single file. A slow pace or brisk tailwind in which cyclists' power outputs are low result in compact formations such that riders ride side-by-side, often filling roads from one side to the other. When two or more groups of riders have reason to contest control of the peloton, several lines may form, each seeking to impose debilitating fatigue on the other teams. Fatigue is a decisive factor in the outcome of every race. Cyclists' range of peripheral vision is a significant factor in peloton formation.
Thus these formations comprise two main phases of behavior: a compact, low-speed formation, and a single-file, high-speed formation. Peloton phases are indicated by thresholds in collective output that can be modeled mathematically and computationally. The principles of phase behavior identified by Trenchard et al. have been applied to optimize engineering problems.
Similarly, these thresholds in peloton formations define transitions between peloton cooperative behavior and free-riding behavior. Cooperation and free-riding in pelotons have been studied using game theory and as a social dilemma, and have also been considered in terms of equivalencies to aspects of economic theory.
Basic peloton behaviors have also been modelled with robots, and principles of peloton behavior have also been considered in relation to the future of collective robot behavior.
Models and simulations
Olds' model
Olds' analysis involved peloton breakaway and chasing groups. He identified the factors involved in determining the likelihood that a breakaway group would succeed in reaching the finish ahead of chasing groups. He identified the following critical factors: distance remaining in the race, the speed of the breakaway group, the number of riders in both the breakaway and chasing groups, how closely riders draft each other, course gradient and roughness, and headwinds and crosswinds (referred to as "demand" factors). Introducing riders' physiological variables including metabolic power production and time to exhausion ("supply" factors), Olds' presents an iterative algorithm for determining the mean power of each group and their relative times to exhaustion, thus determining whether the chasers will catch the breakaway.
Olds' key findings include that group mean velocity increases rapidly as a function of group size up to five or six riders, and then continues to increase but only gradually up to about 20 cyclists; wheel spacing is a significant determinant of group speed due to drafting advantages; mean velocity falls as a function of distance remaining; the required lead time for a breakaway group falls rapidly as the number in the breakaway group increases up to about 10 riders, but flattens as the number of riders in the breakaway group approaches the number of riders in the chasing group. Similarly, Olds' observed that if the chase-group size is less than the size of the breakaway group and the wheel spacing among the chasers is greater than 3 meters, a chasing group will never catch a lead group, assuming other factors remain constant between the groups.
Hoenigman et al.'s model
Agent-based computer models allow for any number of independent "agents" with assigned attributes to interact according to programmed rules of behavior. In this way, simulated global behaviors emerge which can be studied for their properties and compared with actual systems. For their cyclist agents, Hoenigman et al. assigned individual maximum-power-outputs over a heterogeneous range among peloton cyclists and individual and team cooperative attributes in which agents share the most costly front position, or defect by seeking lower-cost drafting positions within the peloton, both according to some probabality. Hoenigman et al. introduced power equations from the literature for non-drafting and drafting positions, an approximate anaerobic threshold as a percentage of cyclists' maximum power when traveling alone without drafting, and a time-to-exhaustion parameter. The authors also introduced a "breakaway" state in which defecting riders increase their speeds to a higher threshold either to breakaway or to catch a group ahead.
The authors performed experiments by varying the noted parameters over a simulated 160 kilometres (99 mi) flat road race containing 15 teams of 10 riders. Cooperators (those willing to take the most costly front position) spend 5 minutes at the front, then rotate to the back of the pack. Defectors spend only one minute at the front. As the race approaches the end, strategies change such that each agent increases their output incrementally based on their remaining energy up to 100% of their maximum power output. Results of the model shows that weaker riders are better off defecting, while cooperation is a good strategy for stronger riders. The results are realistic when compared with real-world competitive cycling and demonstrate the effectiveness of this kind of agent-based model which facilitates accurate identification and analysis of underlying principles of system (in this case, peloton) behavior.
Erick Ratamero's simulation
In his 2013 agent-based peloton simulation, Erick Ratamero applied Wilenski's agent-based flocking model that incorporates three main dynamical parameters: alignment, separation and cohesion. Wilenski's model originates from Craig Reynolds' flocking model that incorporates the same parameters, which he described as velocity matching, collision avoidance, and flock centering.
Ratamaro then applied Sayama's algorithm for cohesive and separating forces to adjust agents' acceleration based on their proportionate spacing within a defined field of vision. Ratamero then introduced cyclists' energetic parameters, adopting elements of Olds' equations for cyclists' energy expenditure, and cyclist performance results from Hoenigman, and Kyle's drafting equation. Ratamero then introduced a threshold energetic quantity to simulate the lactate threshold derived from Hoenigman, whereby cyclist-agents which expend energy above this level will fatigue and eventually fall back in position within the simulated peloton. Thus cyclist-agents expend their energy differentially within the peloton based on their positions and proximity to drafting positions.
Ratamero's model demonstrates that cyclists tend to expend energy more efficiently by participating in well-organized lines in which cyclists advance toward the front, even though they might spend more time in front non-drafting positions than some cyclists internal to the peloton whose continual positional adjustments may result in less time in optimal drafting positions. Ratamero's model exhibits self-organized convection-like behavior which Trenchard described as a phase of peloton behavior.
Trenchard et al.'s simulation
In their 2015 agent-based peloton simulation, Trenchard et al. applied Ratamero's dynamical model, but introduced a different way of modelling the energetic relationships between cyclist-agents. Whereas Ratamero applied a constant maximal sustainable output for all cyclists who then lose energy differentially according to their proximity to drafting positions, Trenchard et al. introduced different maximal sustainable capacities for each cyclist-agent whose positions are determined by the proportion of their maximal capacities to that of the front rider who sets the speed of the peloton. For this they apply the equation:
- PCR = ] / MSOfollow
where PCR is the "peloton convergence ratio", describing two coupled riders; the non-drafting front-rider sets the pace, while the follower obtains the drafting benefit of reduced power output at the same speed as the front rider. Two-cyclist coupling generalizes to multiple rider interactions.
"Pfront" is the power output of the front-rider as she sets the pace within the coupled system;
"d" expresses the follower’s energy savings due to drafting, as a fraction (percentage) of the front-rider’s power output;
"MSOfollow" is the maximal sustainable power output for the follower.
Thus, if Pfront exceeds the follower's MSO, the follower will be unable to sustain the speed of the pace-setting front-rider and must decelerate to a speed less than or equal to that speed representative of the limitation of MSO. A drafting cyclist may operate at or below MSO. If the cyclist is at MSO while drafting but conditions change (e.g., the rider falls too far behind or too far to the side of the optimal drafting position, with respect to the leader), then the follower must decelerate. If she is below MSO while drafting but temporarily falls outside drafting range, she can increase power output to maintain the pace of the leader as long as she does not exceed MSO. This algorithm produces a realistic simulation of oscillating phase behavior between compact and stretched pelotons as speeds vary throughout the course of a race. Trenchard et al. tested the model against an actual set of MSOs for 14 cyclists who participated in a velodrome (track) race. The simulation test produced a realistic simulation of the actual race in terms of phase oscillations and cyclist's relative positions.
Protocooperative behavior in pelotons
Trenchard proposed a theoretical framework for peloton "protocooperative" behavior, a form of cooperation that emerges naturally from physical interactive principles as opposed to ones driven by human competitive, sociological or economic motivations. In this way, protocooperative behavior involves universal principles which Trenchard hypothesizes may be found in many biological systems involving energy saving mechanisms. The parameters of protocooperative behavior include: 1. two or more cyclists coupled by drafting benefit; 2. cyclists' power output or speed; and 3. cyclists' maximal sustainable outputs (MSO). The main characteristics of protocooperative behavior are: 1. a comparatively low-speed phase in which cyclists naturally pass each other and share highest-cost front positions; and 2. a free-riding phase (essentially the singe-file phase identified above), in which cyclists can maintain speeds of those ahead, but cannot pass. The threshold between these two phases is equivalent to the coefficient of drafting (d), below which cooperative behavior occurs and above which free-riding (single-file) occurs up to a second threshold when coupled cyclists diverge. Applying the PCR equation (noted above), the range of cyclists’ MSOs in the free-riding phase is equivalent to the energy savings benefit of drafting (1-d). When driven to maximal speeds, pelotons tend to sort into sub-groups such that their MSO ranges equal the free-riding range (1-d).
Trenchard extracts the following principles:
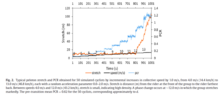
- Weaker cyclists can sustain the pace of the strongest rider between PCR = d and PCR = 1 (d < PCR < 1), as free-riders. In this PCR range, equivalent to the energy savings (1-d), these cyclists cannot pass and share the most costly front position. Stronger cyclists in drafting positions will always be able to pass weaker cyclists ahead.
- As speeds are increased up to maximal speeds set by the strongest riders, cyclists whose MSOs are below the PCR range (1-d), equivalent to the energy saved by drafting, will separate from the peloton (PCR ≥ 1).
- Cyclists can engage in cooperative behavior (passing and sharing most costly front position) in combinations of MSOs at speeds such that PCR ≤ d.
- These principles imply that if cyclists in a peloton exhibit a range of MSOs greater than the energy savings equivalent of drafting (1-d), over time and continual fluctuations in peloton speed, the peloton will divide such that sub-groups fit within the range equivalent of 1-d.
It is this sorting behavior that Trenchard hypothesizes to be a universal evolutionary principle among biological systems coupled by an energy-saving mechanism, which he and collaborators have developed further in relation to extinct trilobites and slime mold
Strategy
![]() | This section needs additional citations for verification. Please help improve this article by adding citations to reliable sources in this section. Unsourced material may be challenged and removed. (October 2024) (Learn how and when to remove this message) |
While the riders at the very front encounter the greatest air resistance (and also those on the windward side when there is a significant crosswind), those behind the first few riders near the front have critical advantages.
Being close to the front means that the rider can see and react to attacks from competitors, and changes in position, with far less effort. Gaps sometimes form in the peloton, and being close to the front reduces the risk of getting caught in the rear group if a break occurs in the peloton, for example, after a crash. Riders near the front are much less likely to have delays due to involvement in crashes.
There is increasing risk of delays or injury from involvement in crashes as one falls farther back in the peloton. In addition, riders are increasingly affected by the accordion effect, in which a change in speed becomes amplified as it propagates to the back of the peloton. The riders following must anticipate and brake early to avoid collisions when the peloton slows. Touching wheels for even a moment normally results in a crash, which spreads across the field in chain reaction as the densely packed riders cannot avoid hitting downed riders and bikes. The entire peloton behind the crash may be stopped.
Being close to the front is also critical in strong crosswind conditions. Cross winds create a significant fatigue penalty for everyone, unless riders form moving groups called echelons in which riders collaborate to form a 'paceline' in a racetrack pattern angled across the road, with the leading rider on the upwind side of the road. Riders for a paceline, such as an echelon, sequentially change positions at short intervals so that no one rider must long accumulate excessive fatigue from facing maximum wind resistance at the leading edge. Echelons are necessarily limited in size by the roadway's width.
When a large peloton is exposed to a significant crosswind on a narrow road, the peloton cannot avoid breaking into a number of small echelons. Teams aware of wind conditions ahead, strong enough to move to the front, well experienced in echelon riding, can gain an important time advantage in these circumstances.
It is critical for riders in contention to win a race to remain near (but not at) the front of the peloton, especially when approaching sharp turns that require braking. Resuming pace after a sharp turn (especially into wind) routinely causes division in a peloton. Once a division occurs, if the will and collective strength of those wisely placed at the front is greater than those behind, the gap between the groups will remain (or increase) to the end of the race, because the extra air resistance for a single rider attempting to move forward to reach the front group imposes an extravagant fatigue penalty, as compared to those who remained protected in the peloton. This is particularly true at high speed on flat roads.
When a team maneuvers to the front of the peloton, it has placed itself in position to dictate the tempo of the race. Teams of riders may prefer a faster or slower tempo depending on the team's tactics.
Being near or at the front of the peloton is critical when initiating a breakaway.
A few strong riders will always attempt to break away from the main peloton, attempting to build such a commanding lead early in the race that the peloton cannot catch up before the finish. Breakaways may succeed when break riders are strong, especially if none of the riders in the break is a danger man (in contention for a win in the overall contest), and if they all pull together as a team. The rider (or riders) who are in the lead and have also successfully broken away from the peloton are referred to as Tête de la Course (a French expression meaning “head of the race”). The peloton will not allow a break with a danger man to get far ahead. Strong teams who want to bring their sprinter into contention for the win come to the front of the peloton and dictate a harsh pace, imposing fatigue on rivals, meanwhile breakaway riders (who individually must spend much more time exposed to the wind than peloton members) sequentially succumb to fatigue and are normally caught. Otherwise successful breaks often fall into disarray just before the finish, where rider calculations regarding personal chances for victory destroy the uneasy break alliance, meanwhile the peloton is catching up quickly.
Tactical factors also apply. Team tactics generally involve clustering their members within the peloton in order to maximize their ability to affect the peloton. For example, if a team member is currently in a breakaway group out in front of the main peloton, the remaining team members will normally make no attempt to accelerate the peloton, to maximize the chances of success for their breakaway group rider. Rarely, they may move to the front of the peloton, and actively seek to check the progress of the peloton at a critical moment. This tactic has the best chance of success on narrow roads, with tight turns, where a single team can fill the road from one side to the other.
In races where the finish is on flat roads, within a few kilometers from the finish, strong teams form into lines, with their principal sprint contender at the rear. The leading rider of each contending team drives forward at the highest pace he can achieve, until he reaches the limit of his endurance, when he then pulls off to the side, allowing the succeeding team member in line to drive forward to his limit. The team sprinter slipstreams at the rear to minimize fatigue due to air resistance until the last hundred meters or so, when the sprinter will choose the moment to dash out from behind his lead-out rider to charge to the finish at the highest possible speed.
See also
- Glossary of bicycling
- Road bicycle racing
- Autobus (cycling) – Group of cyclists behind leading peloton
- Bike bus – Group of utility cyclists
- Platoon (automobile) – Group of vehicles travelling separately but following another
References
- Blocken, Bert (2018-06-30). "Aerodynamic drag in cycling pelotons: New insights by CFD simulation and wind tunnel testing". Journal of Wind Engineering and Industrial Aerodynamics. 179: 1. doi:10.1016/j.jweia.2018.06.011.
- Trenchard, Hugh, 2013. "Peloton phase oscillations," Chaos, Solitons & Fractals, Elsevier, vol. 56(C), pages 194–201.
- ^ Trenchard, Hugh & Richardson, Ashlin & Ratamero, Erick & Perc, Matjaž, 2014. "Collective behavior and the identification of phases in bicycle pelotons," Physica A: Statistical Mechanics and its Applications, Elsevier, vol. 405(C), pages 92–103.
- Alfredo Garcia, Carlos Llorca & Jorge Serra-Planelles (2020) Influence of peloton configuration on the interaction between sport cyclists and motor vehicles on two-lane rural roads, Journal of Transportation Safety & Security, 12:1, 136-150, DOI: 10.1080/19439962.2019.1591557
- Li, M., Chen, T., Du, H., Ma, N., & Xi, X. (2022). The speed and configuration of cyclist social groups: A field study. Physica A: Statistical Mechanics and its Applications, 592, 126849.
- Yau, Nathan (2018-07-27). "Calculating wind drag in the cycling peloton". FlowingData. Retrieved 2024-05-28.
- Trenchard, Hugh and Matjaž Perc. 2016. “Energy saving mechanisms, collective behavior and the variation range hypothesis in biological systems: A review.” Bio Systems 147, 40–66 .
- ^ Trenchard, Hugh, Carlton E. Brett and Matjaž Perc 2017. “Trilobite ‘pelotons’: possible hydrodynamic drag effects between leading and following trilobites in trilobite queues.” Palaeontology 60, 557–569.
- Trenchard, Hugh. “Cell pelotons: A model of early evolutionary cell sorting, with application to slime mold Dictyostelium discoideum.” Journal of Theoretical Biology 469 (2019): 75–95 .
- Trenchard, H. 2012. “American coot collective on-water dynamics.” Nonlinear Dynamics, Psychology, and Life Sciences, 17, 183–203.
- Belden, J., Mansoor, M.M., Hellum, A., Rahman, S.R., Meyer, A., Pease, C., Pacheco, J., Koziol, S. and Truscott, T.T., 2019. "How vision governs the collective behaviour of dense cycling pelotons." Journal of the Royal Society Interface, 16(156), p.20190197.
- Trenchard, Hugh & Ratamero, Erick & Richardson, Ashlin & Perc, Matjaž, 2015. "A deceleration model for bicycle peloton dynamics and group sorting," Applied Mathematics and Computation, Elsevier, vol. 251(C), pages 24-3
- Poitras, G., Cormier, G. and Nobelle, AS. 2018. "Novel Optimization Algorithm for Composite Steel Deck Floor Systems: Peloton Dynamics Optimization (PDO)." Building Tomorrow's Society, Canadian Society for Civil Engineering annual conference, June 13–16, 2018
- ^ Trenchard, H. 2015. "The peloton superorganism and protocooperative behavior," Applied Mathematics and Computation, Elsevier, vol. 270(C), pages 179–192
- Brouwer, T., & Potters, J. 2019. "Friends for (almost) a day: Studying breakaways in cycling races." Journal of Economic Psychology, 75(part B), . https://doi.org/10.1016/j.joep.2018.08.001
- Hoenigman, Rhonda, Elizabeth Bradley, and Allen Lim. "Cooperation in bike racing—when to work together and when to go it alone." Complexity 17, no. 2 (2011): 39–44.
- Mignot, J.F., 2016. "Strategic behavior in road cycling competitions." In: The economics of professional road cycling (pp. 207–231). Springer, Cham.
- Trenchard, Hugh, and Matjaz Perc. "Equivalences in biological and economical systems: Peloton dynamics and the rebound effect." PLOS ONE 11, no. 5 (2016): e0155395.
- Bedruz, Rhen Anjerome, Argel A. Bandala, Ryan Rhay Vicerra, Ronnie Concepcion, and Elmer Dadios. "Design of a Robot Controller for Peloton Formation Using Fuzzy Logic." In 2019 7th International Conference on Robot Intelligence Technology and Applications (RiTA), pp. 83-88. IEEE, 2019.
- Bedruz, Rhen Anjerome R., Jose Martin Z. Maningo, Arvin H. Fernando, Argel A. Bandala, Ryan Rhay P. Vicerra, and Elmer P. Dadios. "Dynamic Peloton Formation Configuration Algorithm of Swarm Robots for Aerodynamic Effects Optimization." In 2019 7th International Conference on Robot Intelligence Technology and Applications (RiTA), pp. 264–267. IEEE, 2019.
- Trenchard, H., 2018. "When Robots Get Bored and Invent Team Sports: A More Suitable Test than the Turing Test?." Information, 9(5), p.118.
- ^ Olds, T. 1998. "The mathematics of breaking away and chasing in cycling". Eur J App Phsiol, 77, 492–497
- Wooldridge, M. An Introduction to Multi-Agent Systems; Wiley: United Kingdom, 2009.
- ^ Hoenigman, R., Bradley, E., Lim, A.2011. "Cooperation in bike racing when to work together and when to go it alone." Complexity 17(2) 39–44
- Martins Ratamero, E., 2013, September. Modelling peloton dynamics in competitive cycling: a quantitative approach. In International Congress on Sports Science Research and Technology Support. Springer, Cham. 42-56
- Wilensky, U. 1998. Netlogo flocking model. Centre for Connected Learning and Computer-based modeling, Northwestern University, Evanston, Il.
- Reynold, C. 1987. "Flocks, Herds, and Schools: A Distributed Behavioral Model." SIGGRAPH '87 (Computer Graphics 21(4), July), 25–34.
- Sayama, H.2007. "Decentralized control and interactive design methods for large-scale heterogeneous self-organizing swarms." In: Advances in Artificial Life. Springer 675-684
- Olds, T.1998. The mathematics of breaking away and chasing in cycling. European journal of applied physiology and occupational physiology 77(6), 492-497
- Kyle, C.R. 1979. "Reduction of wind resistance and power output of racing cyclists and runners travelling in groups." Ergonomics 22(4) 387-397
- Hoenigman, 2011
- Trenchard, H. 2012. The complex dynamics of bicycle pelotons. arXiv preprint arXiv:1206.0816
- ^ Trenchard et al. (2015)
- Trenchard, H. 2015. "The peloton superorganism and protocooperative behavior," Applied Mathematics and Computation, Elsevier, vol. 270(C), at 183
- Trenchard, Hugh. “Cell pelotons: A model of early evolutionary cell sorting, with application to slime mold Dictyostelium discoideum.” Journal of Theoretical Biology 469 (2019): 75–95.
- Macur, Juliet (2009-07-06). "Sixth Sense Has Armstrong in Third Place". New York Times. Archived from the original on 2011-07-20.
- Ratamero, 2013
- Olds, 1998
- Ratamero, 2013
- Scelles, N., Mignot, J.-F., Cabaud, B. and François, A. (2018), "Temporary organizational forms and coopetition in cycling: What makes a breakaway successful in the Tour de France?", Team Performance Management, Vol. 24 No. 3/4, pp. 122–134. https://doi.org/10.1108/TPM-03-2017-0012
- Wolf, S. and Saupe, D., 2017. How to stay ahead of the pack: optimal road cycling strategies for two cooperating Riders. International Journal of Computer Science in Sport, 16(2), pp.88-100.
External links
- Hochman, Paul (2006-06-01). "Pack mentality". Fortune. New York City: Time Inc. ISSN 0015-8259. Archived from the original on 2007-12-18.
Cut deals with the enemy, maximize liquidity, punish the welshers, and other free-market lessons of the typical bike race.
- Miller, John W. (2010-07-23). "When the Middle of the Pack Is Good". The Wall Street Journal. New York City: Dow Jones & Company. ISSN 0099-9660.
At Tour de France, the Peloton Shields Top Riders, but It Comes With Rules; 'Moving Community'
- Blocken, Bert (2018). "Aerodynamic drag in cycling pelotons: New insights by CFD simulation and wind tunnel testing". Journal of Wind Engineering & Industrial Aerodynamics. 179: 319–337. doi:10.1016/j.jweia.2018.06.011.